An attention graph stacked autoencoder for anomaly detection of electro-mechanical actuator using spatio-temporal multivariate signals
Chinese Journal of Aeronautics(2024)
摘要
Health monitoring of electro-mechanical actuator (EMA) is critical to ensure the security of airplanes. It is difficult or even impossible to collect enough labeled failure or degradation data from actual EMA. The autoencoder based on reconstruction loss is a popular model that can carry out anomaly detection with only consideration of normal training data, while it fails to capture spatio-temporal information from multivariate time series signals of multiple monitoring sensors. To mine the spatio-temporal information from multivariate time series signals, this paper proposes an attention graph stacked autoencoder for EMA anomaly detection. Firstly, attention graph convolution is introduced into autoencoder to convolve temporal information from neighbor features to current features based on different weight attentions. Secondly, stacked autoencoder is applied to mine spatial information from those new aggregated temporal features. Finally, based on the benchmark reconstruction loss of normal training data, different health thresholds calculated by several statistic indicators can carry out anomaly detection for new testing data. In comparison with traditional stacked autoencoder, the proposed model could obtain higher fault detection rate and lower false alarm rate in EMA anomaly detection experiment.
更多查看译文
关键词
anomaly detection,spatio-temporal information,multivariate time series signals,attention graph convolution,stacked autoencoder
AI 理解论文
溯源树
样例
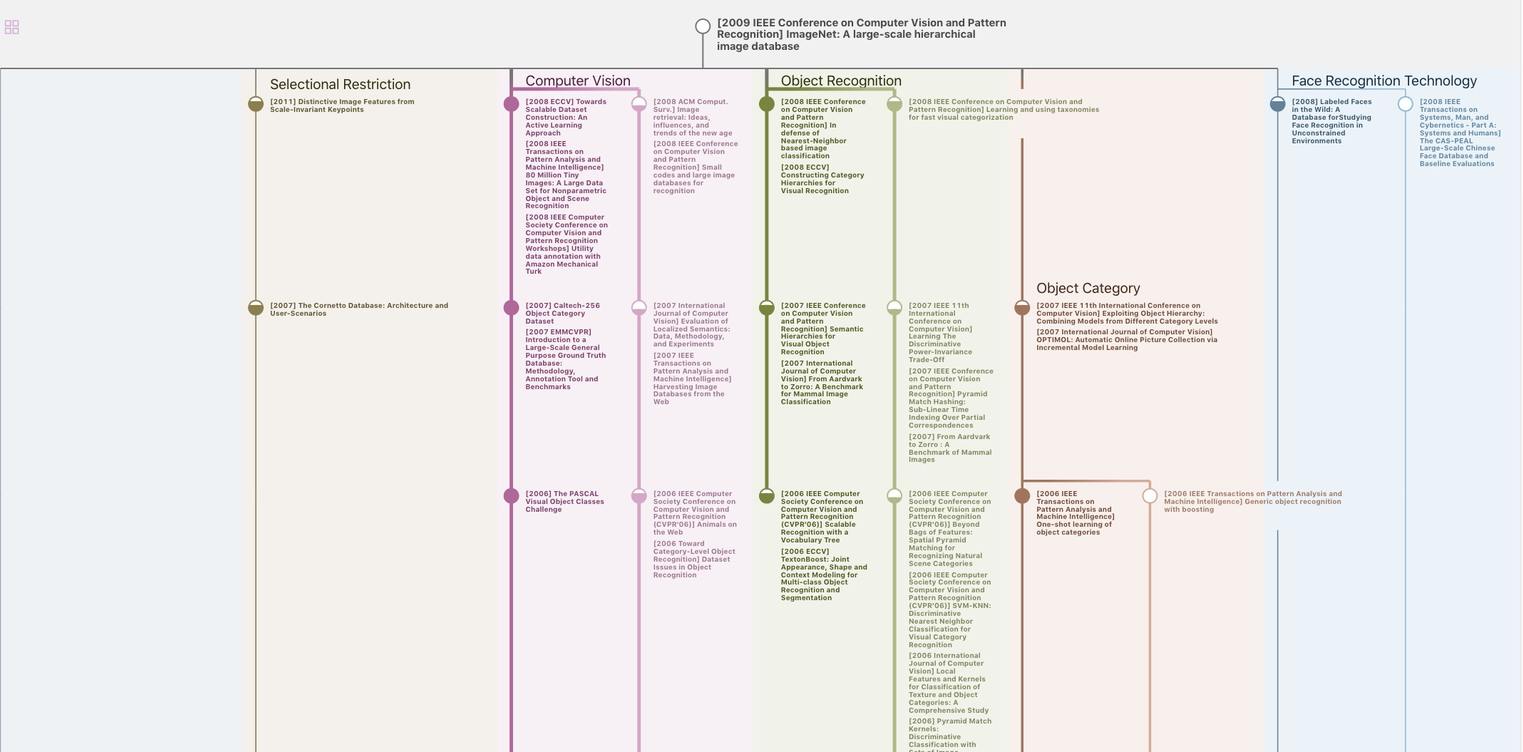
生成溯源树,研究论文发展脉络
Chat Paper
正在生成论文摘要