An Ordering of Divergences for Variational Inference with Factorized Gaussian Approximations
arxiv(2024)
摘要
Given an intractable distribution p, the problem of variational inference
(VI) is to compute the best approximation q from some more tractable family
𝒬. Most commonly the approximation is found by minimizing a
Kullback-Leibler (KL) divergence. However, there exist other valid choices of
divergences, and when 𝒬 does not contain p, each divergence
champions a different solution. We analyze how the choice of divergence affects
the outcome of VI when a Gaussian with a dense covariance matrix is
approximated by a Gaussian with a diagonal covariance matrix. In this setting
we show that different divergences can be ordered by the amount that
their variational approximations misestimate various measures of uncertainty,
such as the variance, precision, and entropy. We also derive an impossibility
theorem showing that no two of these measures can be simultaneously matched by
a factorized approximation; hence, the choice of divergence informs which
measure, if any, is correctly estimated. Our analysis covers the KL divergence,
the Rényi divergences, and a score-based divergence that compares ∇log
p and ∇log q. We empirically evaluate whether these orderings hold
when VI is used to approximate non-Gaussian distributions.
更多查看译文
AI 理解论文
溯源树
样例
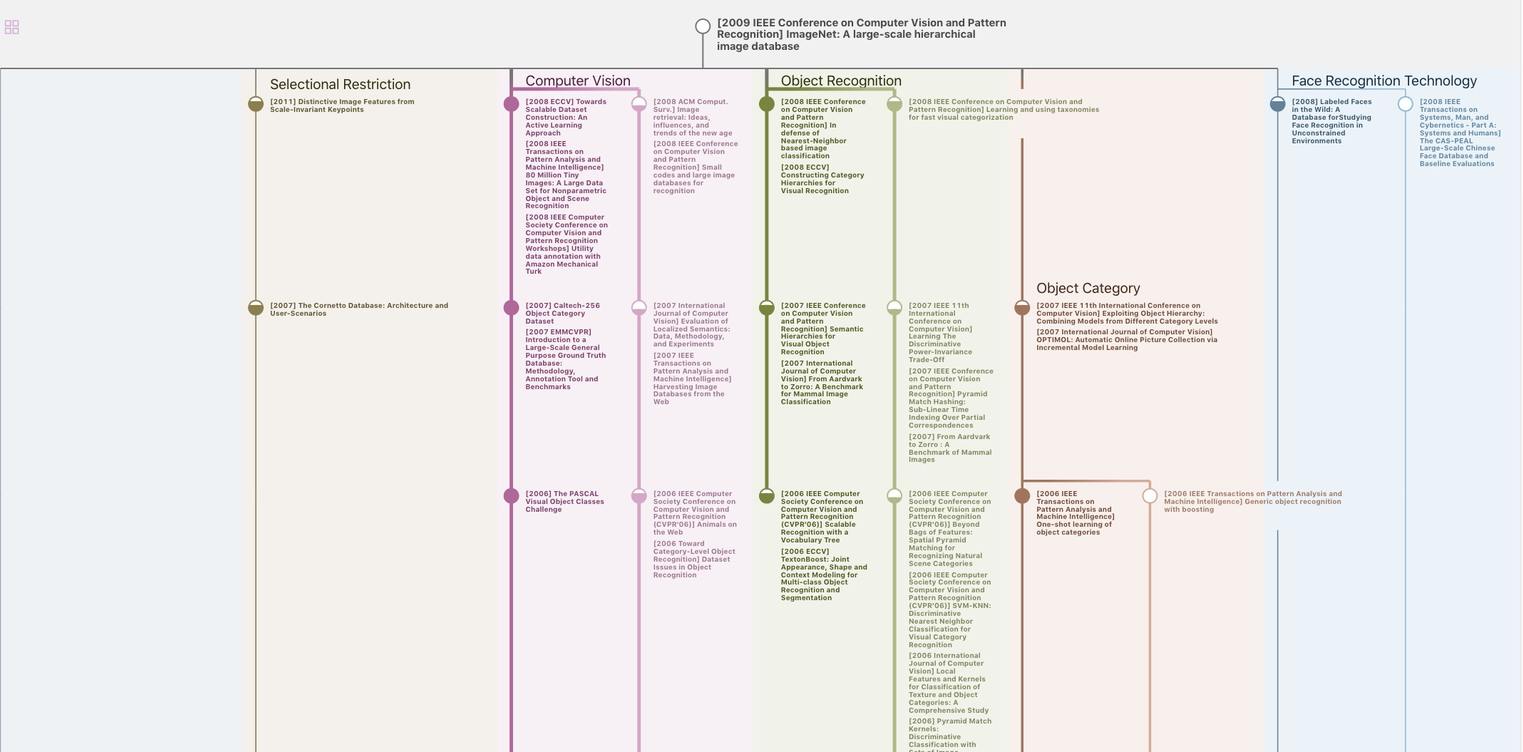
生成溯源树,研究论文发展脉络
Chat Paper
正在生成论文摘要