DetDiffusion: Synergizing Generative and Perceptive Models for Enhanced Data Generation and Perception
CVPR 2024(2024)
摘要
Current perceptive models heavily depend on resource-intensive datasets,
prompting the need for innovative solutions. Leveraging recent advances in
diffusion models, synthetic data, by constructing image inputs from various
annotations, proves beneficial for downstream tasks. While prior methods have
separately addressed generative and perceptive models, DetDiffusion, for the
first time, harmonizes both, tackling the challenges in generating effective
data for perceptive models. To enhance image generation with perceptive models,
we introduce perception-aware loss (P.A. loss) through segmentation, improving
both quality and controllability. To boost the performance of specific
perceptive models, our method customizes data augmentation by extracting and
utilizing perception-aware attribute (P.A. Attr) during generation.
Experimental results from the object detection task highlight DetDiffusion's
superior performance, establishing a new state-of-the-art in layout-guided
generation. Furthermore, image syntheses from DetDiffusion can effectively
augment training data, significantly enhancing downstream detection
performance.
更多查看译文
AI 理解论文
溯源树
样例
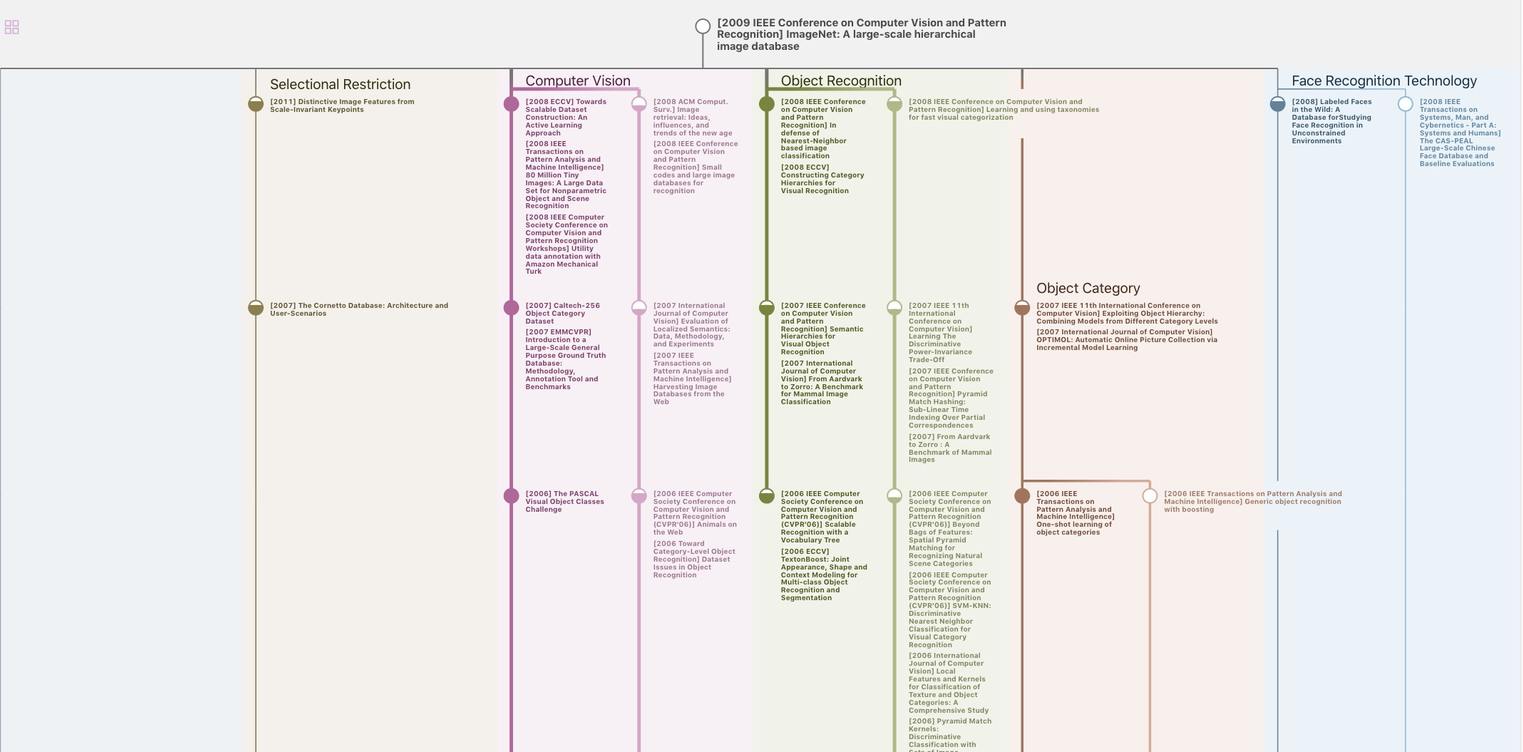
生成溯源树,研究论文发展脉络
Chat Paper
正在生成论文摘要