GTMFuse: Group-Attention Transformer-Driven Multiscale Dense Feature-Enhanced Network for Infrared and Visible Image Fusion
Knowledge-Based Systems(2024)
摘要
Infrared and visible images captured by different devices can be seamlessly integrated into a single composite image through the application of image fusion techniques. However, many existing convolutional neural network-based methods for infrared and visible image fusion have exhibited limited capability for effectively amalgamating information from the source images. Consequently, we propose a group-attention transformer into the multiscale feature enhanced network for infrared and visible image fusion, which we abbreviate as GTMFuse. Specifically, GTMFuse employs multiscale dual-channel encoders to independently process the source image and extract multiscale features. Among the encoders, the group-attention transformer module is utilized to facilitate more comprehensive long-range feature dependency modeling at each scale. This innovative module seamlessly combines a fixed-direction stripe attention mechanism with channel attention and window attention, enabling comprehensive global long-range information capture and interaction with feature information across the source images. The multiscale features obtained from the group-attention transformer module are integrated into the fused image through a meticulously designed dense fusion block. Furthermore, this study introduces a novel dataset named HBUT-IV, encompassing surveillance images captured from multiple viewpoints. The HBUT-IV dataset serves as a valuable benchmark for assessing the efficacy of fusion methods. Extensive experiments are conducted on four datasets employing nine comparative methods, revealing the superior performance of the GTMFuse approach. The implementation code is accessible at https://github.com/XingLongH/GTMFuse.
更多查看译文
关键词
Infrared and visible image fusion,Deep learning,Group-attention,Multiscale feature
AI 理解论文
溯源树
样例
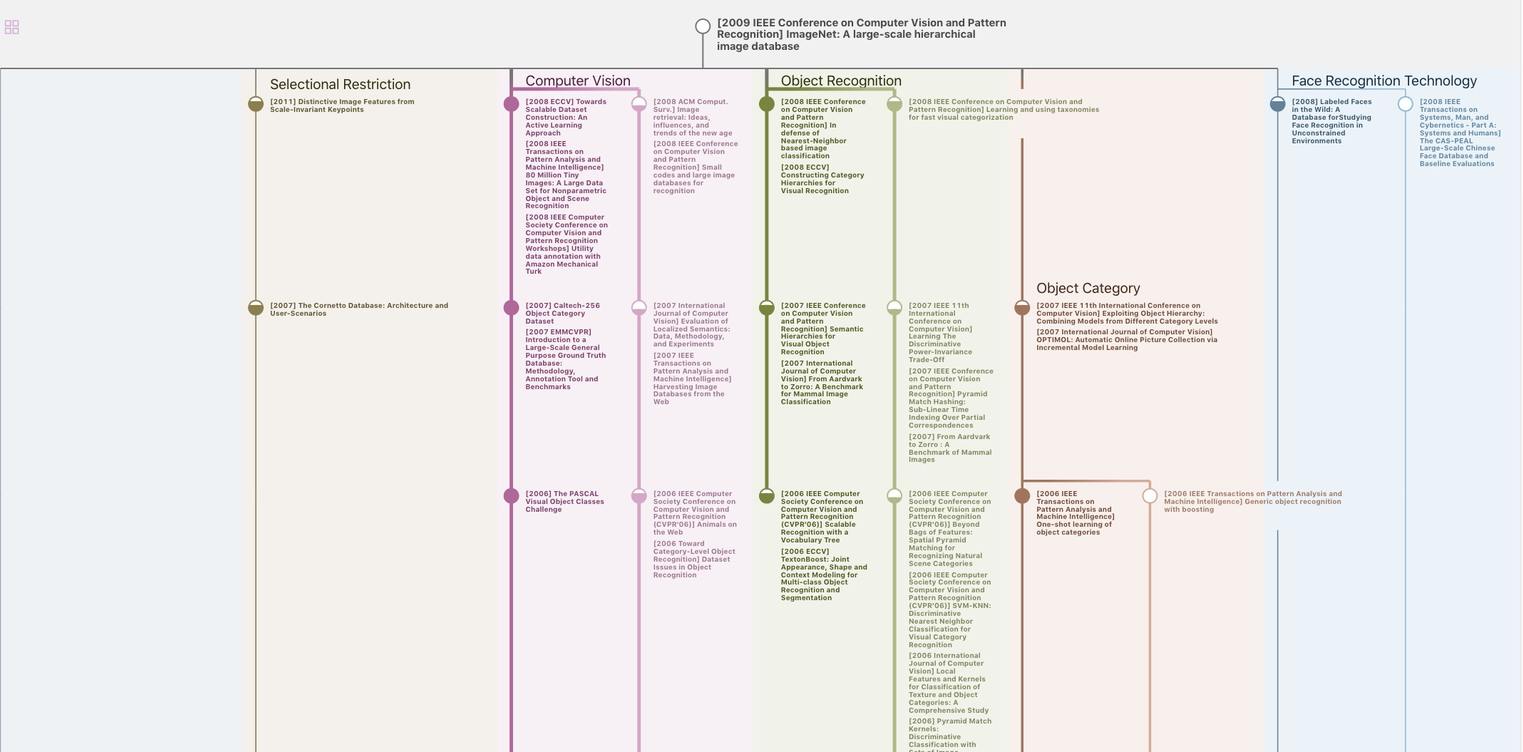
生成溯源树,研究论文发展脉络
Chat Paper
正在生成论文摘要