Graph regularized autoencoding-inspired non-negative matrix factorization for link prediction in complex networks using clustering information and biased random walk
The Journal of Supercomputing(2024)
摘要
The task of link prediction has become a fundamental research problem in the analysis of complex networks. However, most existing non-negative matrix factorization (NMF) methods are decoding models and solely consider a single type of structural information around node pairs in networks, resulting in sub-optimal prediction accuracy. To solve these limitations, we propose a novel model, namely Graph Regularized Autoencoder-inspired Non-negative Matrix Factorization via jointly Clustering information and Biased random walk (GRANMFCB for short), for link prediction. Specifically, GRANMFCB comprises both encoder and decoder components, fully leveraging the advantages of autoencoders. In addition, clustering information and high-order structures are utilized to preserve abundant structural information around node pairs and against sparsity of networks. Finally, effective iterative multi-multiplication updating rules are proposed to optimize the objective function and the convergence is strictly proved. Extensive experimental results on twelve real-world networks show that our proposed model outperforms the state-of-the-art approaches. Codes are available at https://github.com/litongf/GRANMFCB .
更多查看译文
关键词
Link prediction,Autoencoding-inspired,Clustering information,Biased random walk
AI 理解论文
溯源树
样例
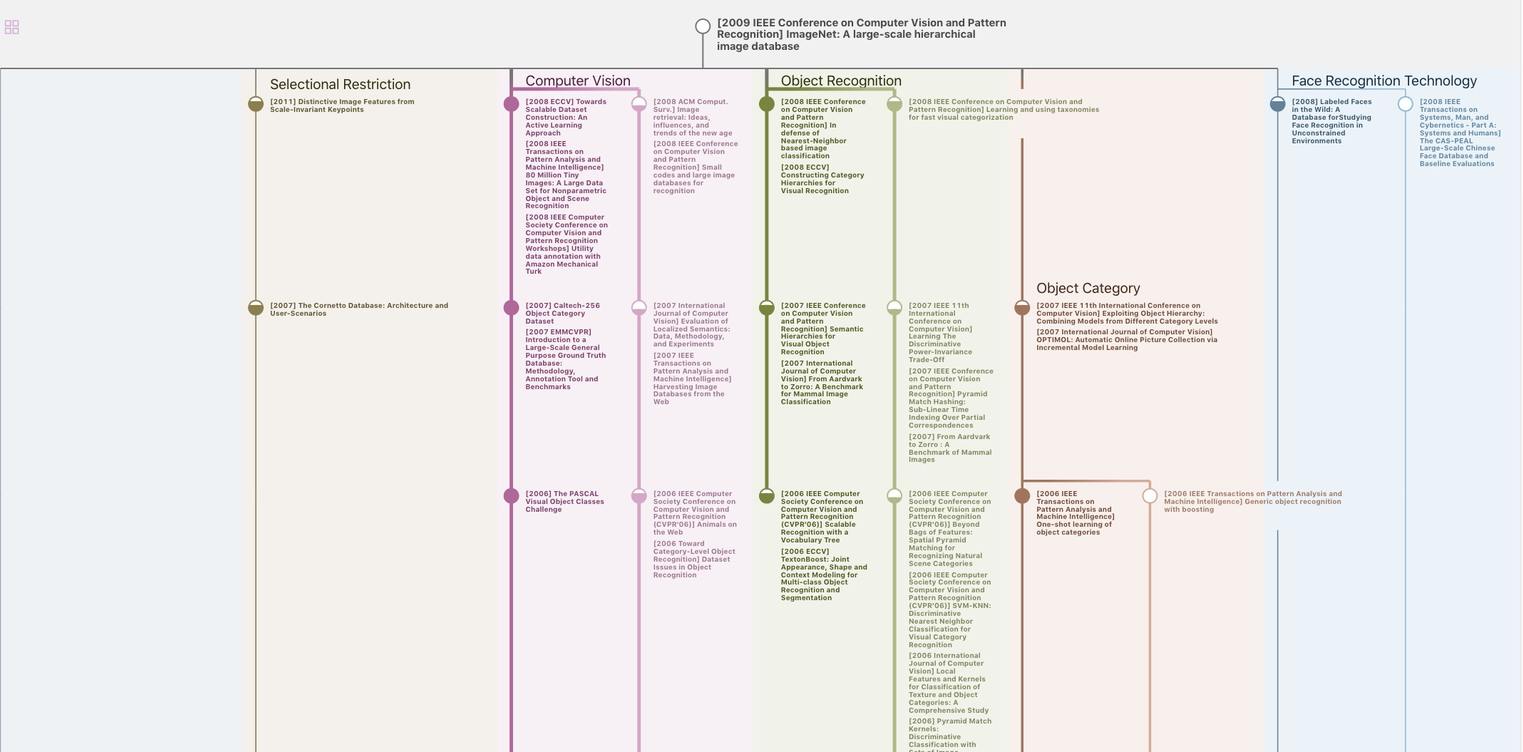
生成溯源树,研究论文发展脉络
Chat Paper
正在生成论文摘要