A hybrid neural ordinary differential equation model of the cardiovascular system
JOURNAL OF THE ROYAL SOCIETY INTERFACE(2024)
摘要
In the human cardiovascular system (CVS), the interaction between the left and right ventricles of the heart is influenced by the septum and the pericardium. Computational models of the CVS can capture this interaction, but this often involves approximating solutions to complex nonlinear equations numerically. As a result, numerous models have been proposed, where these nonlinear equations are either simplified, or ventricular interaction is ignored. In this work, we propose an alternative approach to modelling ventricular interaction, using a hybrid neural ordinary differential equation (ODE) structure. First, a lumped parameter ODE model of the CVS (including a Newton-Raphson procedure as the numerical solver) is simulated to generate synthetic time-series data. Next, a hybrid neural ODE based on the same model is constructed, where ventricular interaction is instead set to be governed by a neural network. We use a short range of the synthetic data (with various amounts of added measurement noise) to train the hybrid neural ODE model. Symbolic regression is used to convert the neural network into analytic expressions, resulting in a partially learned mechanistic model. This approach was able to recover parsimonious functions with good predictive capabilities and was robust to measurement noise.
更多查看译文
关键词
cardiovascular system,ordinary differential equations,time series,neural network,symbolic regression
AI 理解论文
溯源树
样例
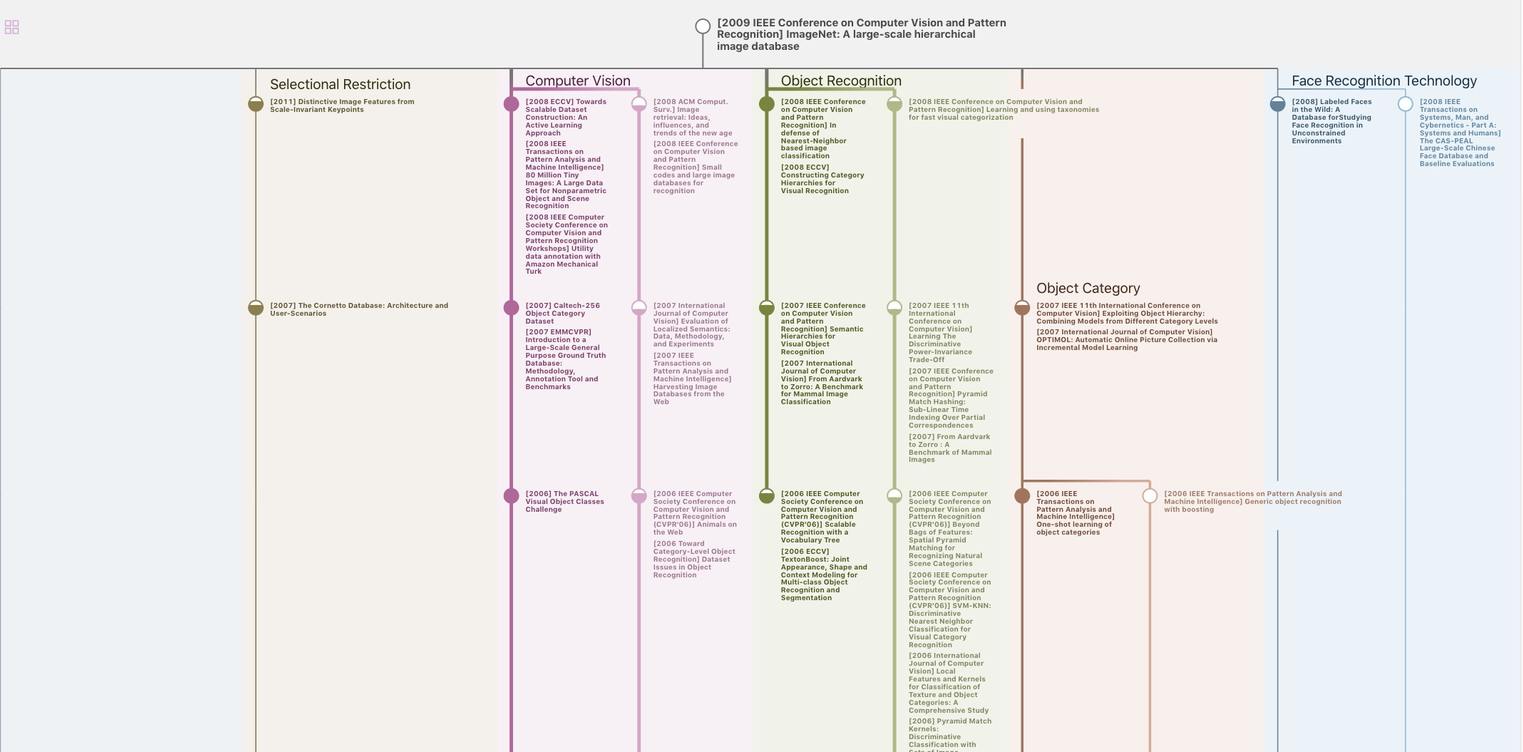
生成溯源树,研究论文发展脉络
Chat Paper
正在生成论文摘要