A methodology to guide companies in using Explainable AI-driven interfaces in manufacturing contexts
Procedia Computer Science(2024)
摘要
Nowadays, the increasing integration of artificial intelligence (AI) technologies in manufacturing processes is raising the need of users to understand and interpret the decision-making processes of complex AI systems. Traditional black-box AI models often lack transparency, making it challenging for users to comprehend the reasoning behind their outputs. In contrast, Explainable Artificial Intelligence (XAI) techniques provide interpretability by revealing the internal mechanisms of AI models, making them more trustworthy and facilitating human-AI collaboration. In order to promote XAI models’ dissemination, this paper proposes a matrix-based methodology to design XAI-driven user interfaces in manufacturing contexts. It helps in mapping the users’ needs and identifying the “explainability visualization types” that best fits the end users’ requirements for the specific context of use. The proposed methodology was applied in the XMANAI European Project (https://ai4manufacturing.eu), aimed at creating a novel AI platform to support XAI-supported decision making in manufacturing plants. Results showed that the proposed methodology is able to guide companies in the correct implementation of XAI models, realizing the full potential of AI while ensuring human oversight and control.
更多查看译文
关键词
explainable AI (XAI),artificial intelligence (AI),manufacturing,Human-Machine Interaction,user interface
AI 理解论文
溯源树
样例
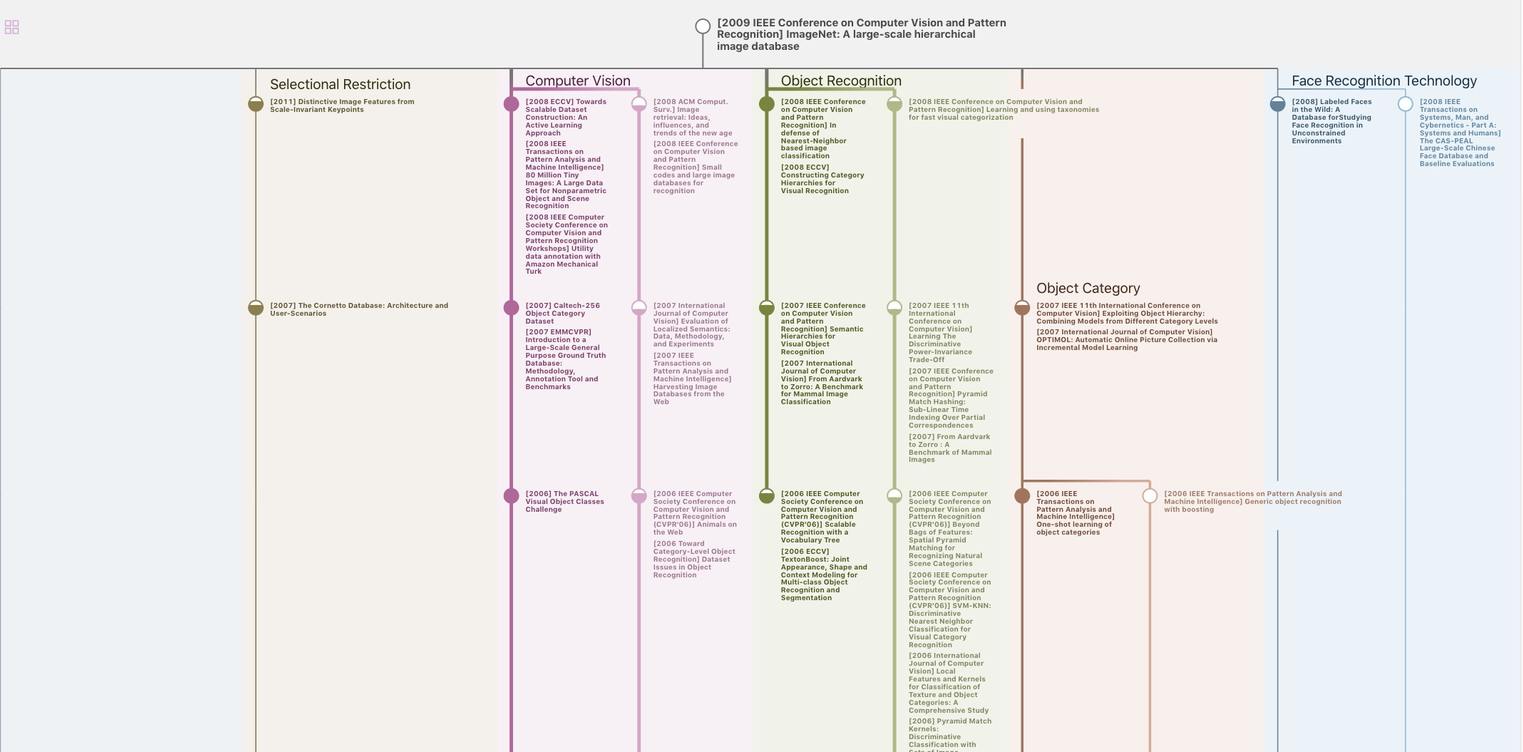
生成溯源树,研究论文发展脉络
Chat Paper
正在生成论文摘要