Using Deep Discriminative Clustering to Explore Unsupervised Open Domain Adaptation
2023 China Automation Congress (CAC)(2023)
摘要
Closed Set Domain Adaptation (CSDA) always assumes that domains share the same label space, and this assumption is no longer satisfied with reality. In real world, the label spaces of different domains are often asymmetric, and target domain has unknown instances that are not available in source domain, making the acquisition of “unknown” knowledge crucial. Therefore, Open Set Domain Adaptation (OSDA) is proposed to solve the problem of unequal label spaces. OSDA is not only to reduce domain discrepancy, but also to identify known and unknown classes, and to suppress negative migration caused by unknown samples. In this paper, our proposed Knowledge Discrimination Module (KDM) can extract rich semantic features from known and unknown samples effectively, and Class Matching Clustering (CMC) can aggregate known class features to achieve implicit alignment of known classes in source and target domains, and separate unknown class features from them to alienate them from known class feature space, thus allowing unknown samples to be correctly distinguished and aggregated together. The method outperforms other methods on Digits and Office-31 standard benchmarks, and the validity and feasibility of our method is verified by ablation experiments and visual analysis.
更多查看译文
AI 理解论文
溯源树
样例
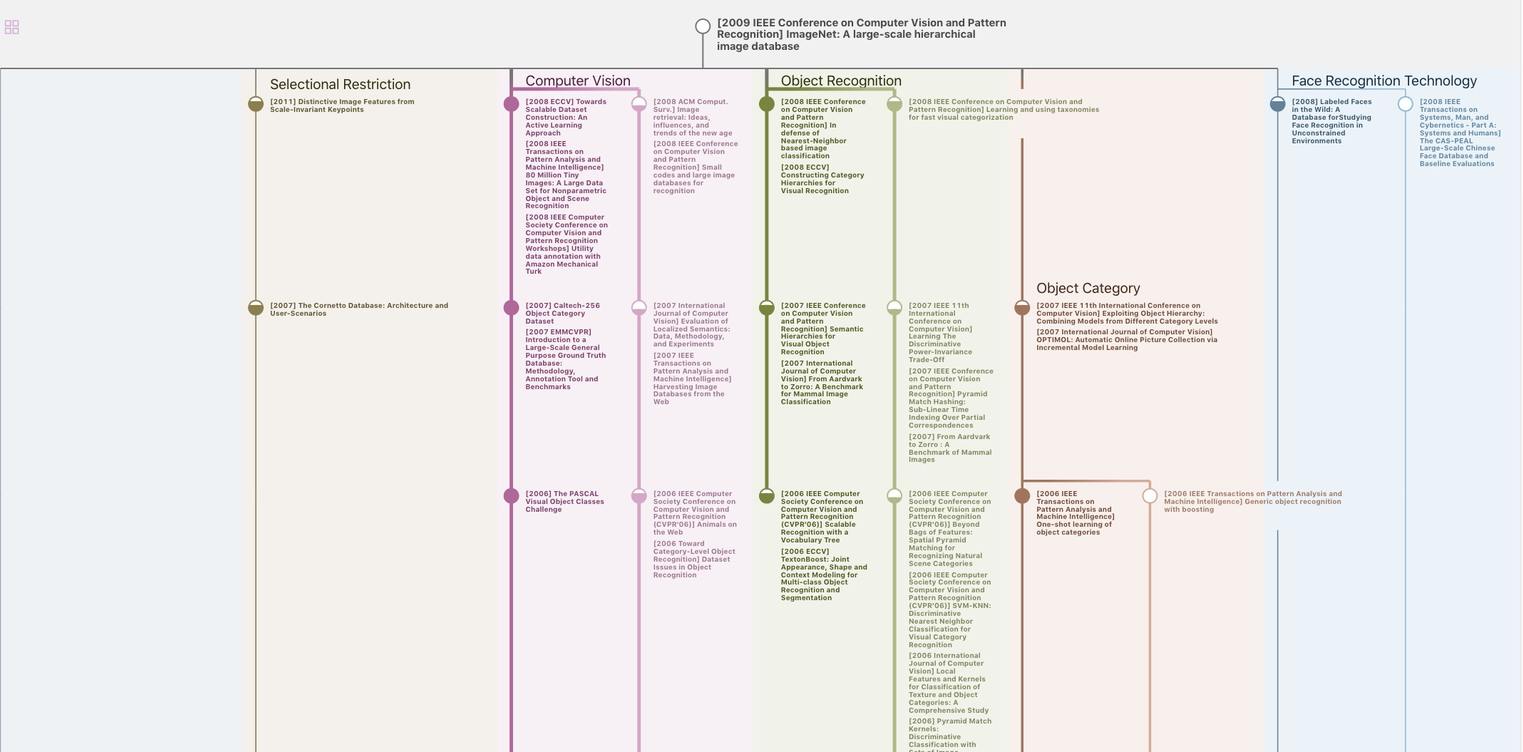
生成溯源树,研究论文发展脉络
Chat Paper
正在生成论文摘要