A Novel Semantic Global Descriptor for 3D Point Cloud Place Recognition
2023 IEEE 6th International Conference on Electronics and Communication Engineering (ICECE)(2023)
摘要
Place recognition provides global pose constraints to Simultaneous Localization and Mapping (SLAM) systems to eliminate cumulative errors over time. Semantic-driven LiDAR-based methods have been in great graces due to its accurate range information, strong illumination invariance and descriptive semantic information. However, the performance improvement has encountered bottlenecks due to inaccurate pose estimation and lack of encoding function with strong representation ability. To tackle these issues, a novel semantic-driven LiDAR-based global descriptor is proposed in this paper. A two-step global Generalized semantic iterative closest points (GSICP) algorithm is proposed to estimate accurate relative pose transformation to align point clouds. After achieving invariance of pose, point clouds are divided into bins. Subsequently an effective encoding function which can aggregate information of each bin and its neighborhood is designed to construct the descriptor. Whether the loop closure occurs is determined by the similarity score of descriptors. Detailed experiments constructed on the KITTI odometry dataset demonstrate the outperformed performance of proposed method.
更多查看译文
AI 理解论文
溯源树
样例
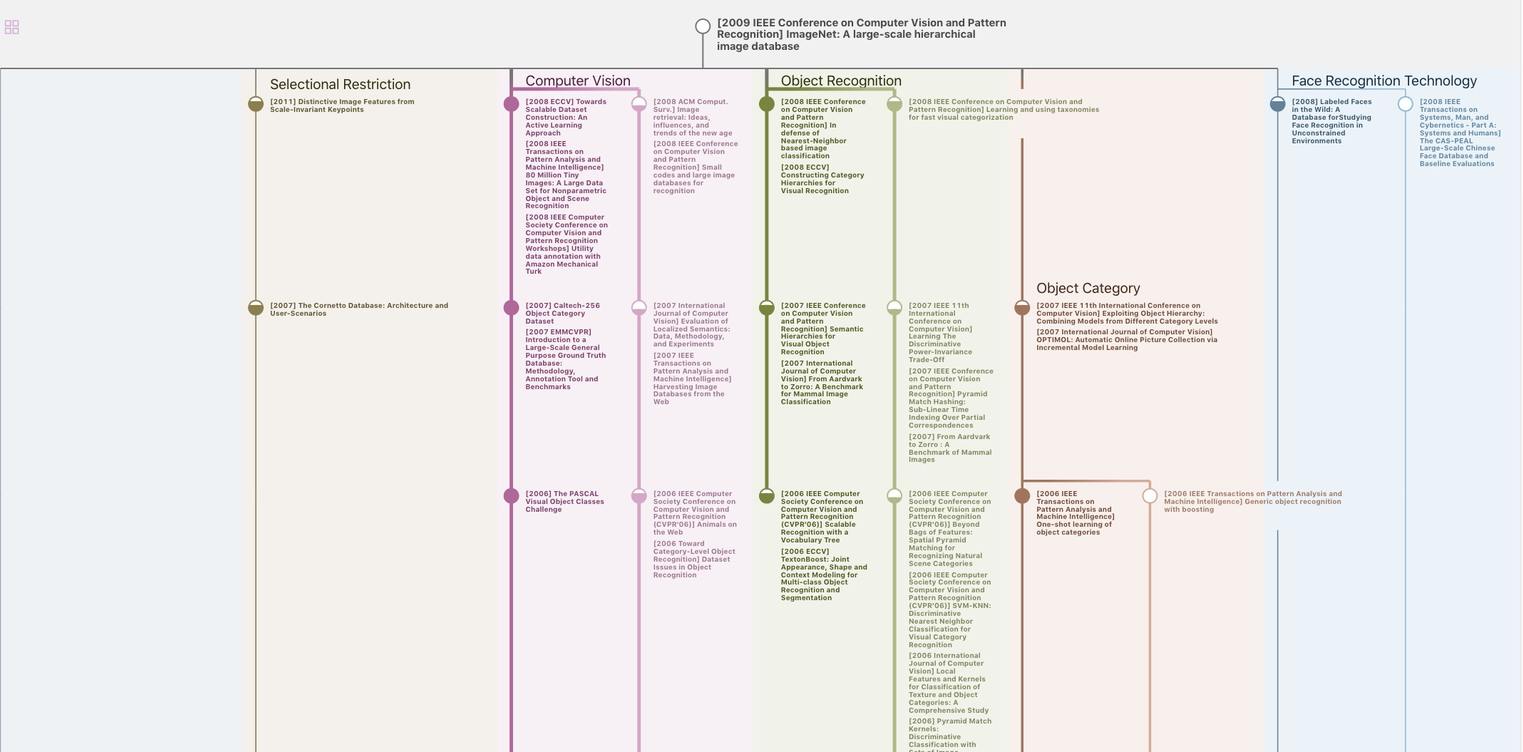
生成溯源树,研究论文发展脉络
Chat Paper
正在生成论文摘要