Challenges and Countermeasures of Federated Learning Data Poisoning Attack Situation Prediction
Mathematics(2024)
摘要
Federated learning is a distributed learning method used to solve data silos and privacy protection in machine learning, aiming to train global models together via multiple clients without sharing data. However, federated learning itself introduces certain security threats, which pose significant challenges in its practical applications. This article focuses on the common security risks of data poisoning during the training phase of federated learning clients. First, the definition of federated learning, attack types, data poisoning methods, privacy protection technology and data security situational awareness are summarized. Secondly, the system architecture fragility, communication efficiency shortcomings, computing resource consumption and situation prediction robustness of federated learning are analyzed, and related issues that affect the detection of data poisoning attacks are pointed out. Thirdly, a review is provided from the aspects of building a trusted federation, optimizing communication efficiency, improving computing power technology and personalized the federation. Finally, the research hotspots of the federated learning data poisoning attack situation prediction are prospected.
更多查看译文
关键词
federated learning,data poisoning,situation prediction,privacy protection
AI 理解论文
溯源树
样例
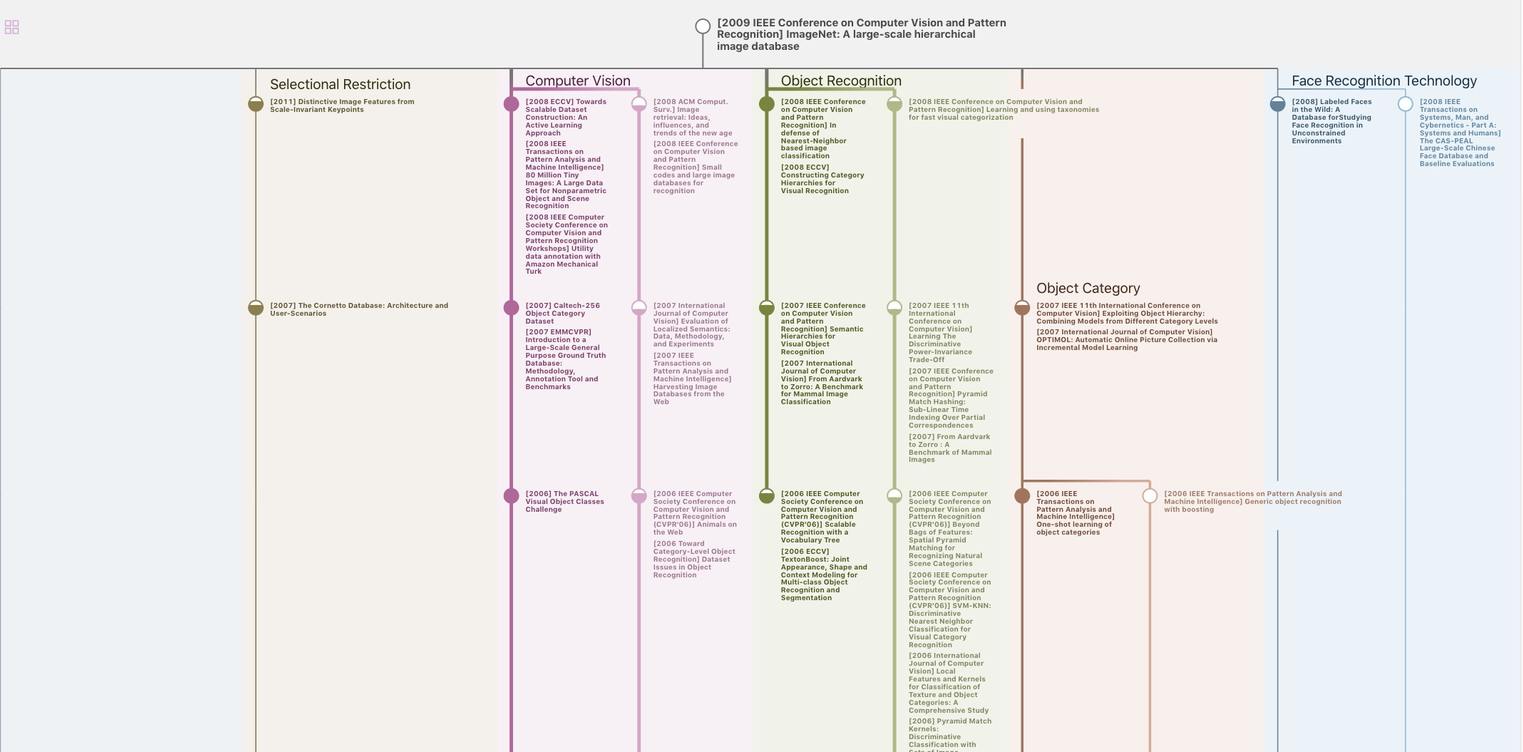
生成溯源树,研究论文发展脉络
Chat Paper
正在生成论文摘要