Early diagnosis of polycystic ovarian syndrome (PCOS) using machine learning: an ensemble learning approach
2023 International Conference on Modeling, Simulation & Intelligent Computing (MoSICom)(2023)
摘要
In recent years, women’s reproductive health has become a significant concern. Issues like preterm abortions, infertility, ovarian disorders, and declining fertility rates are prevalent. Polycystic Ovarian Syndrome (PCOS), a common reproductive disorder, often leads to infertility due to irregular cycles and elevated androgens. Despite its unclear cause and cure, early detection and intervention are vital. Researchers are exploring AI-based diagnostics to expedite diagnosis and mitigate clinical challenges. Our methodology revolves around leveraging non-invasive parameters to construct a feature vector optimized for machine learning algorithms. We utilize Principal Component Analysis (PCA) as a crucial step for dimensionality reduction, which streamlines the dataset’s representation while retaining vital information. To bolster the accuracy of PCOS diagnosis, we deploy a majority voting ensemble model that incorporates five base models. This ensemble approach not only enhances classification precision but also addresses issues like overfitting and model robustness, making it especially valuable when dealing with datasets of limited size. The accuracy, precision, f1-score, and recall for the suggested model are found to be 84.3%, 76.1%, 81%, and 84.3% respectively. Our research shows that our ensemble model performs better overall and across individual classes than the fundamental models. This development represents a substantial advancement in the field of PCOS diagnosis, showcasing the pivotal role of machine learning in enhancing diagnostic precision.
更多查看译文
AI 理解论文
溯源树
样例
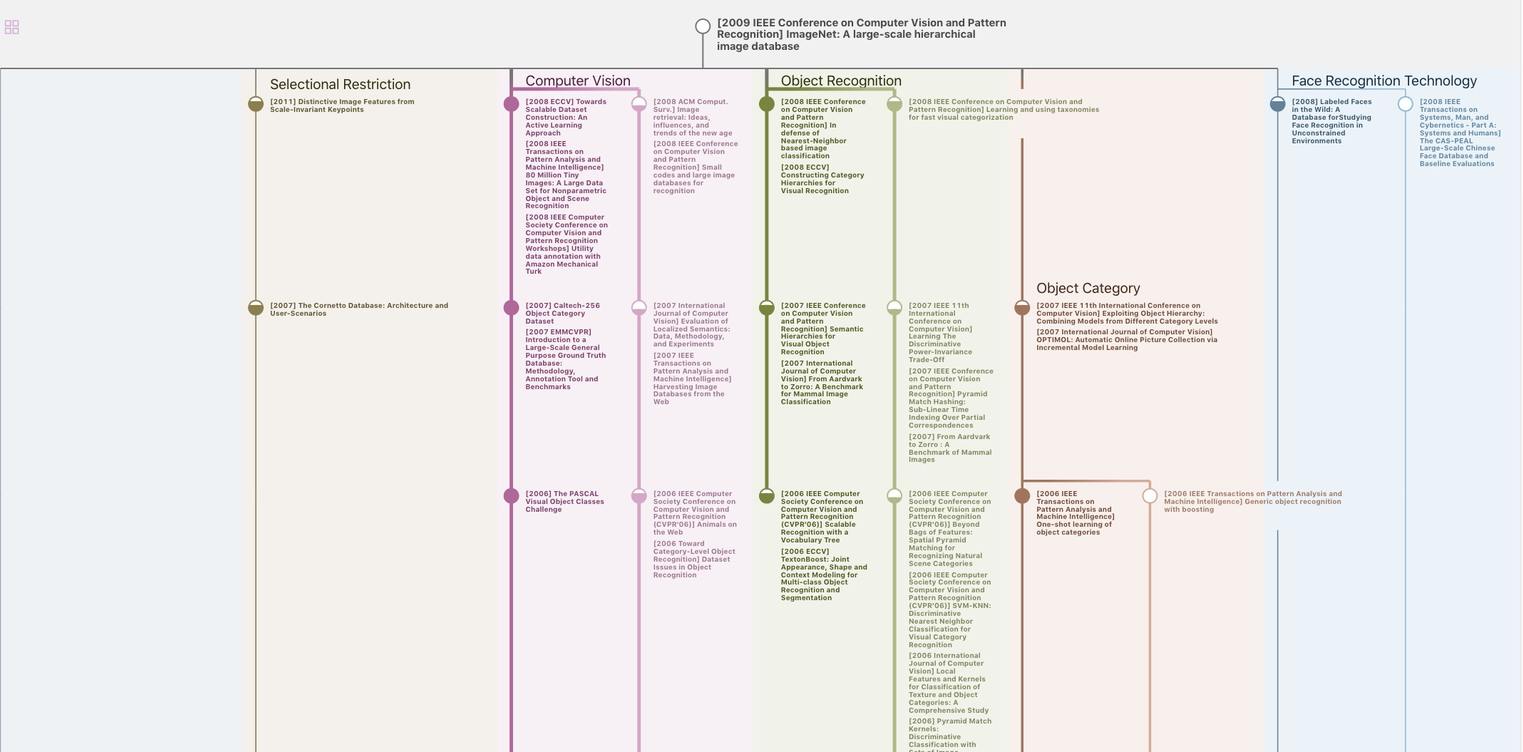
生成溯源树,研究论文发展脉络
Chat Paper
正在生成论文摘要