Enhancing Pseudo-Labeling Performance in Object Detection Using Gaussian Mixture Modeled Uncertainty.
International Conference on Electronics, Information and Communications(2024)
摘要
Object detection research has been rapidly advancing. However, it requires large amounts of training data, where labeling massive datasets incurs great cost and time. To address this problem, semi-supervised learning techniques have been increasingly explored, among which pseudo-labeling has become popular due to its straightforward approach. However, pseudo-labeling has limitations with confidence score-based filtering. In this paper, we propose a method to extract uncertainties using Gaussian mixture models and effectively incorporate them into the labeling process to overcome these limitations. The proposed method achieves more reliable pseudo-labeling results and experiments show a 0.8% performance improvement compared to the existing approach.
更多查看译文
AI 理解论文
溯源树
样例
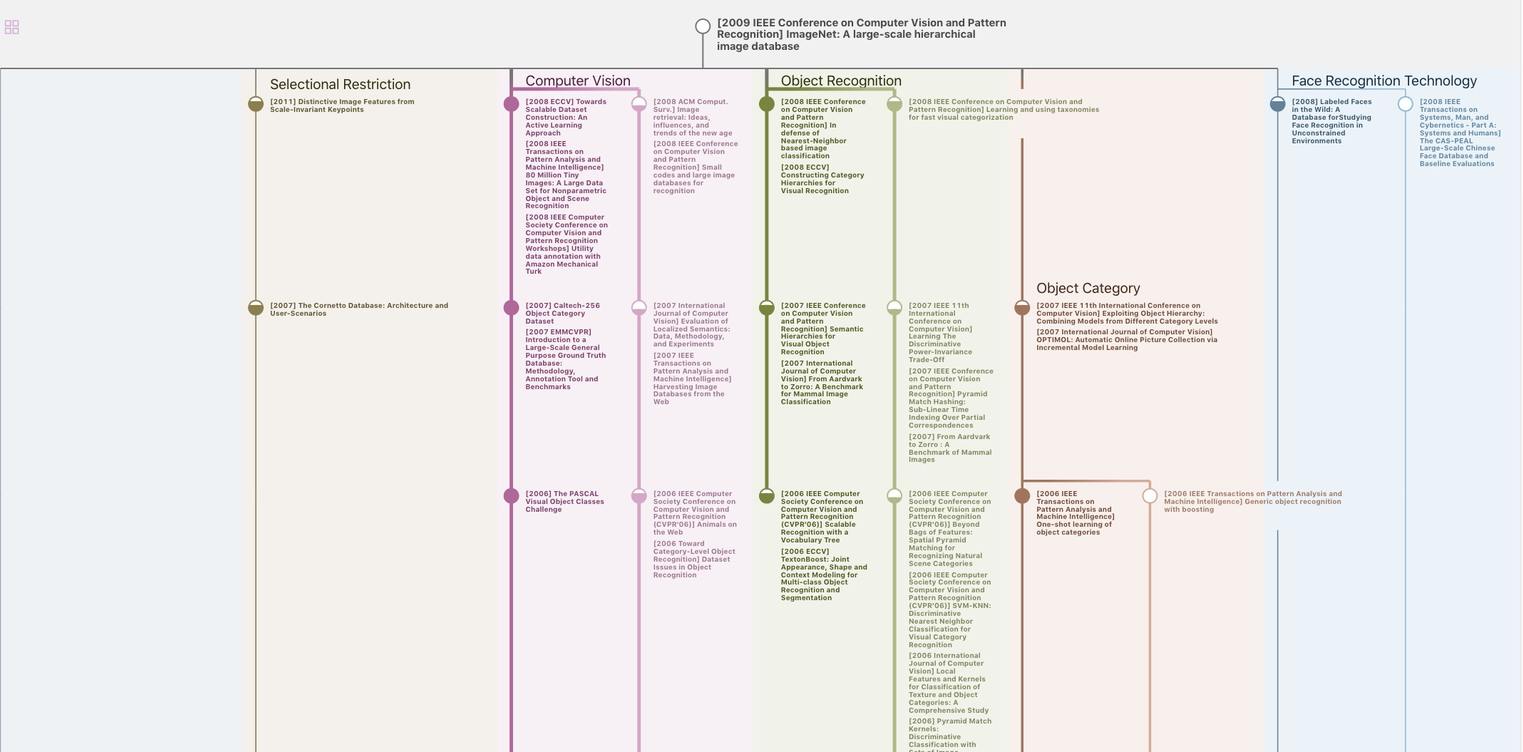
生成溯源树,研究论文发展脉络
Chat Paper
正在生成论文摘要