Improved Generalization from Limiting Attention in a Transformer for Sleep Stage Classification.
International Conference on Electronics, Information and Communications(2024)
摘要
A transformer architecture has been employed effectively on many tasks such as natural language processing and vision recognition. The most important and general requirement of utilizing the transformer-based architecture is that the model has to be trained on a large-scale dataset before it can be fine-tuned for downstream tasks. However, in our experiments, we figure out that the transformer-based architecture has better generalization capability to extract features from data samples in sleep stage classification than CNN-based architectures, even with a small-scale dataset without any extra pretraining step. In this paper, we show the strength of the transformer architecture with regard to generalization capability over the conventional CNN architecture in sleep stage classification tasks specifically using a small-scale dataset.
更多查看译文
AI 理解论文
溯源树
样例
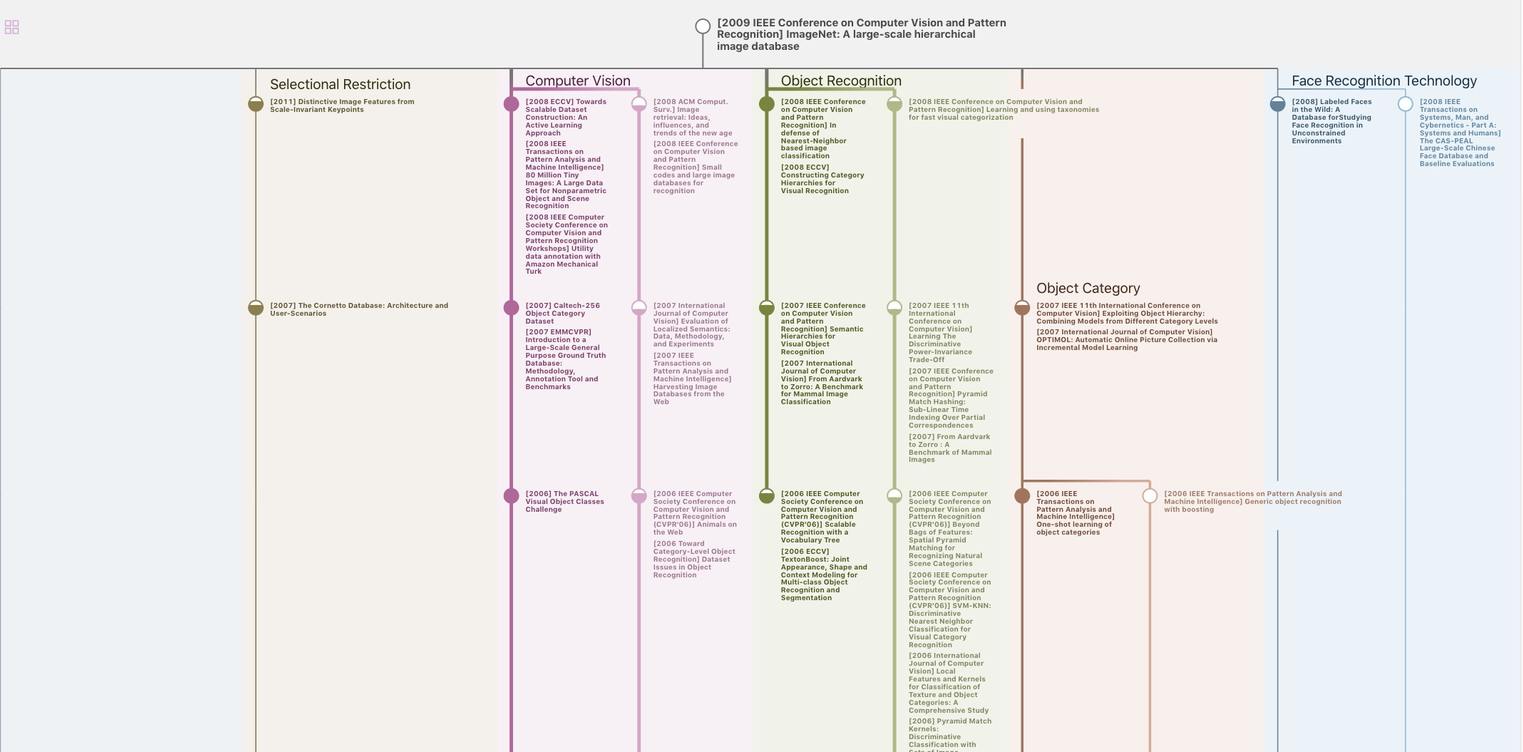
生成溯源树,研究论文发展脉络
Chat Paper
正在生成论文摘要