Graph Pyramid Autoformer for Long- Term Traffic Forecasting.
International Conference on Machine Learning and Applications(2023)
摘要
Accurate traffic forecasting is vital to an intelligent transportation system. Although many deep learning models have achieved state-of-art performance for short-term traffic forecasting of up to 1 hour, long-term traffic forecasting that spans multiple hours remains a major challenge. To that end, we develop Graph Pyramid Autoformer (GPA), an attention-based spatial-temporal graph neural network that uses a novel pyramid autocorrelation attention mechanism. It enables learning from long temporal sequences on graphs and improves long-term traffic forecasting accuracy. We demonstrate the efficacy of the GPA using two benchmark traffic datasets: Los Angeles' METR-LA and the Bay Area's PEMS-BAY. Notably, our model has outperformed a range of existing state-of-the-art methods, delivering up to a 25 % improvement in the accuracy of long-term traffic forecasts. Our code is available at: https://github.com/WeiheneZlExplainable-Graph-Autoformer.
更多查看译文
AI 理解论文
溯源树
样例
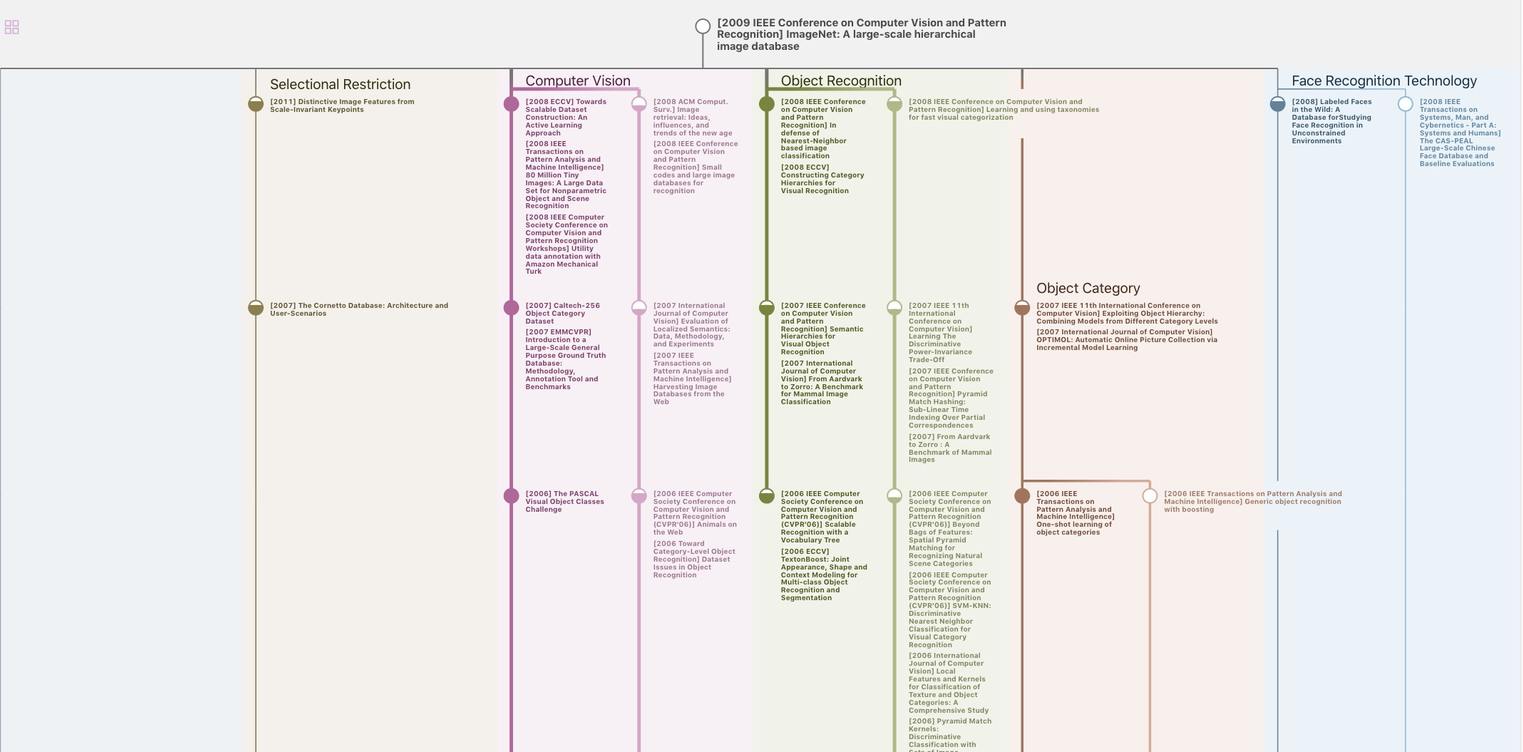
生成溯源树,研究论文发展脉络
Chat Paper
正在生成论文摘要