VADAR: A Vision-based Anomaly Detection Algorithm for Railroads.
Euromicro Symposium on Digital Systems Design(2023)
摘要
Detecting damages and anomalies on railroads is a tedious and expensive task. This paper proposes the Vision-based Anomaly Detection Algorithm for Railroads (VADAR), which can find rail damages and foreign objects on the trackbed in monochrome images captured by a train-mounted camera system. VADAR analyzes the input image with three Autoencoders (AEs), a segmentation network, and a one-class classifier. The detection of unknown anomalies justifies our architecture's advantage, i.e., no anomalies are necessary for training VADAR. In experiments with a dataset of over 218,000 images, VADAR achieves a detection accuracy of 95% and a recall rate of 70% for smaller and up to 100% for bigger instances of several anomaly classes. Compared with a state-of-the-art approach which is based on more expensive equipment, VADAR achieves accuracy and recall rates (for anomalies of particular interest) of about 22pps and up to 45pps higher, respectively. With a setting that achieves 83.5% accuracy, VADAR's recall rate outperforms the state-of-the-art approach for every anomaly class and object size.
更多查看译文
AI 理解论文
溯源树
样例
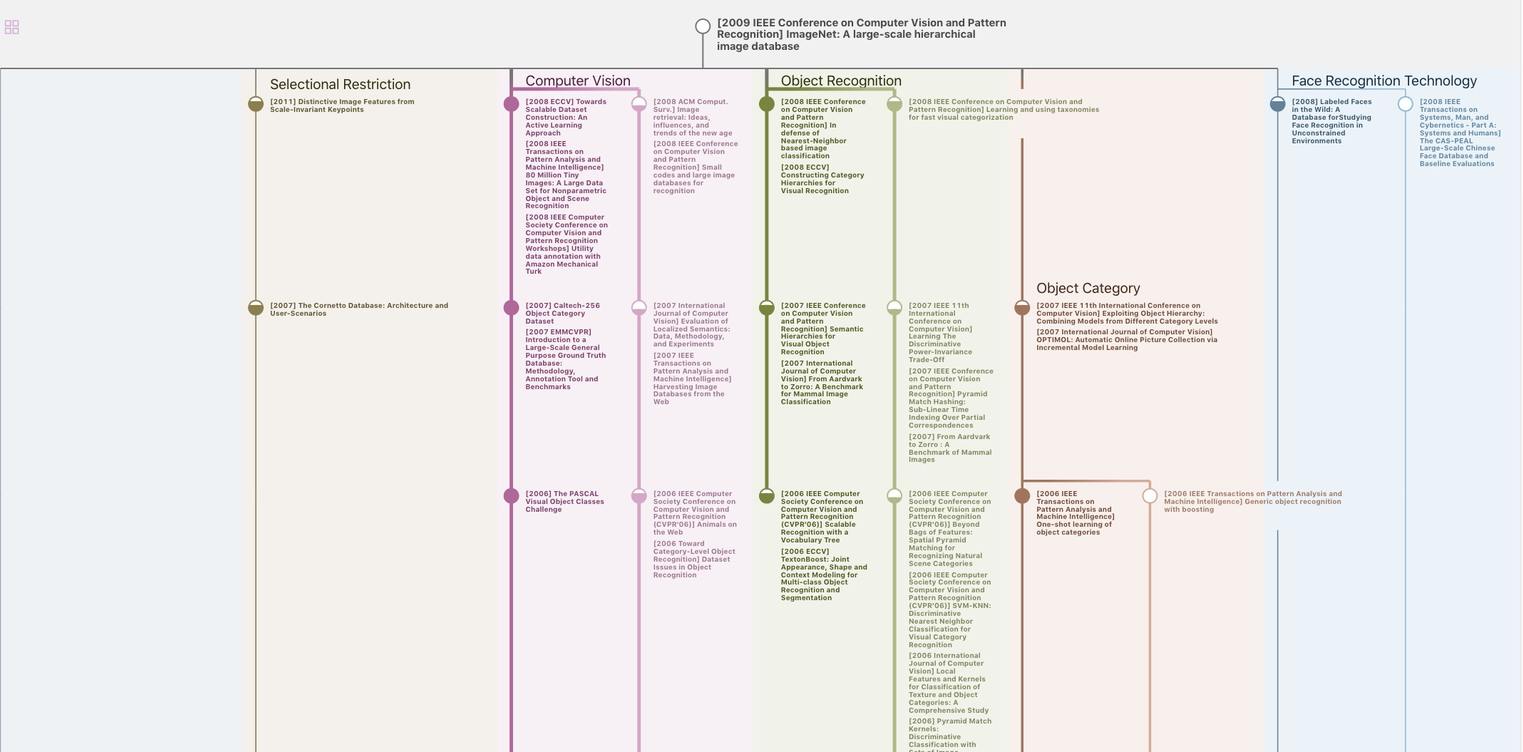
生成溯源树,研究论文发展脉络
Chat Paper
正在生成论文摘要