Molecular descriptor-enhanced graph neural network for energetic molecular property prediction
Science China Materials(2024)
摘要
Energetic molecules (EMs) play an important role in both military and civilian applications. Traditionally, determining the physicochemical parameters of EMs requires experimental workload and inherent risks while new-rising machine learning (ML) methods are promising to address this challenge. In this work, we report a molecular descriptor-enhanced graph neural network (MD-enhanced GNN) model to accurately and fast predict three detonation parameters of EMs. This model integrates sequence-based molecular descriptors and structure-based graph vectors, offering a comprehensive framework that does not require custom descriptors. Accordingly, we construct an EMs dataset that includes 18,991 CHNO EMs and compare our model with sole molecular fingerprint/descriptor and GNN methods. It is found that our proposed MD-enhanced GNN integration method achieves superior accuracy with R2 over 0.93 and a learning speed improvement of over 20
更多查看译文
关键词
energetic molecules,molecular descriptors,graph neural network
AI 理解论文
溯源树
样例
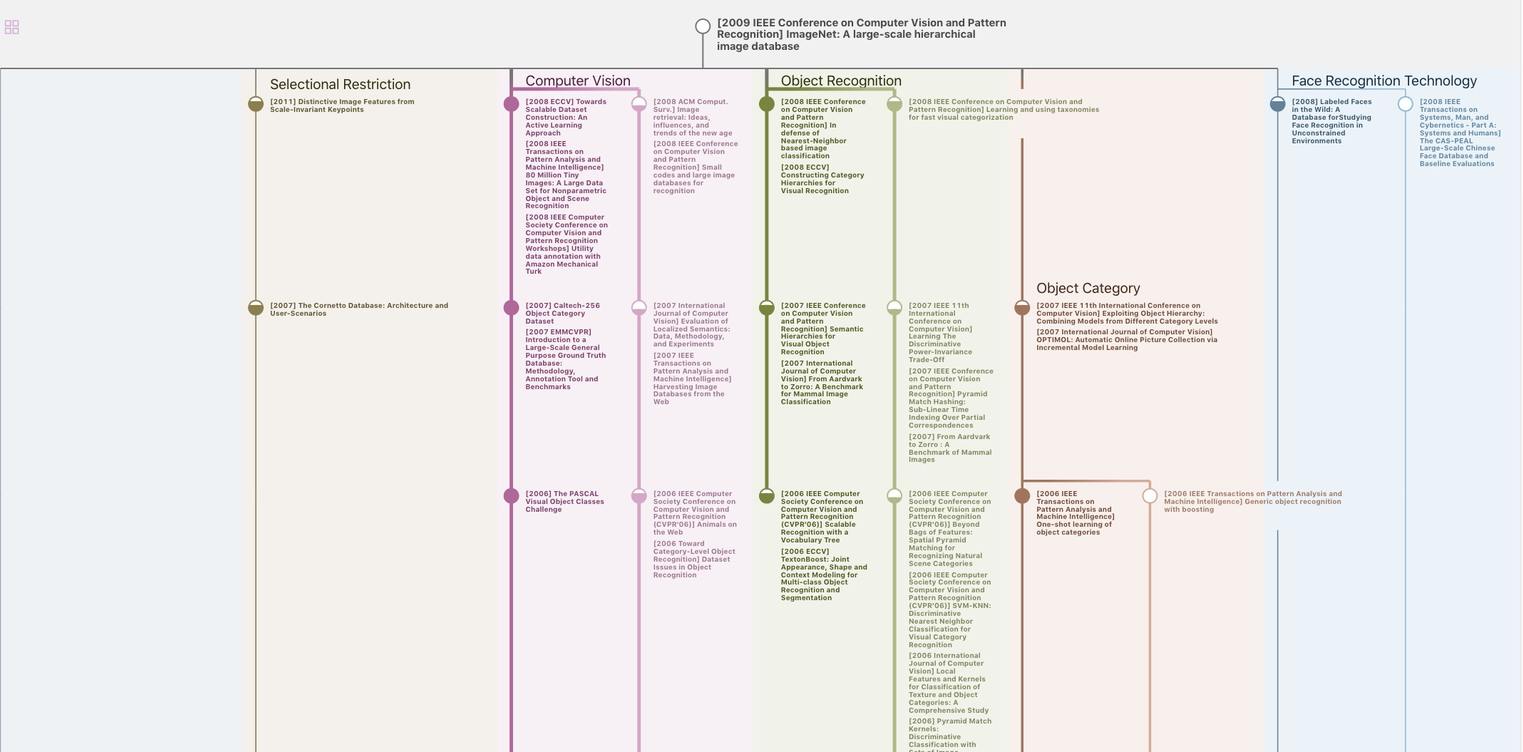
生成溯源树,研究论文发展脉络
Chat Paper
正在生成论文摘要