Epileptic Electroencephalogram Signal Classification Based on Shearlet and Contourlet Transforms.
International Conference on Machine Learning and Applications(2023)
摘要
According to the World Health Organization (2023), epilepsy is a chronic brain disorder that affects around 50 million people of all ages worldwide, mainly (approximately 80%) those living in low- and middle-income regions. The most common method used to diagnose epilepsy is Electroencephalography (EEG), which involves monitoring brain activity. However, an-alyzing EEG recordings to detect epileptic seizures can be a tiresome and time-consuming task, even for experts. In addition, different physicians may have varying levels of experience, leading to differing diagnostic opinions. As the main contribution of this work, we propose and evaluate a novel method that combines time-frequency transforms and 1D convolutional neural networks to extract and process EEG features. Experiments performed on a widely used public data set show that our approach is competitive when compared to other EEG signal classification methods.
更多查看译文
关键词
Epilepsy,electroencephalogram signals,shear-lets,contourlets,convolutional neural networks
AI 理解论文
溯源树
样例
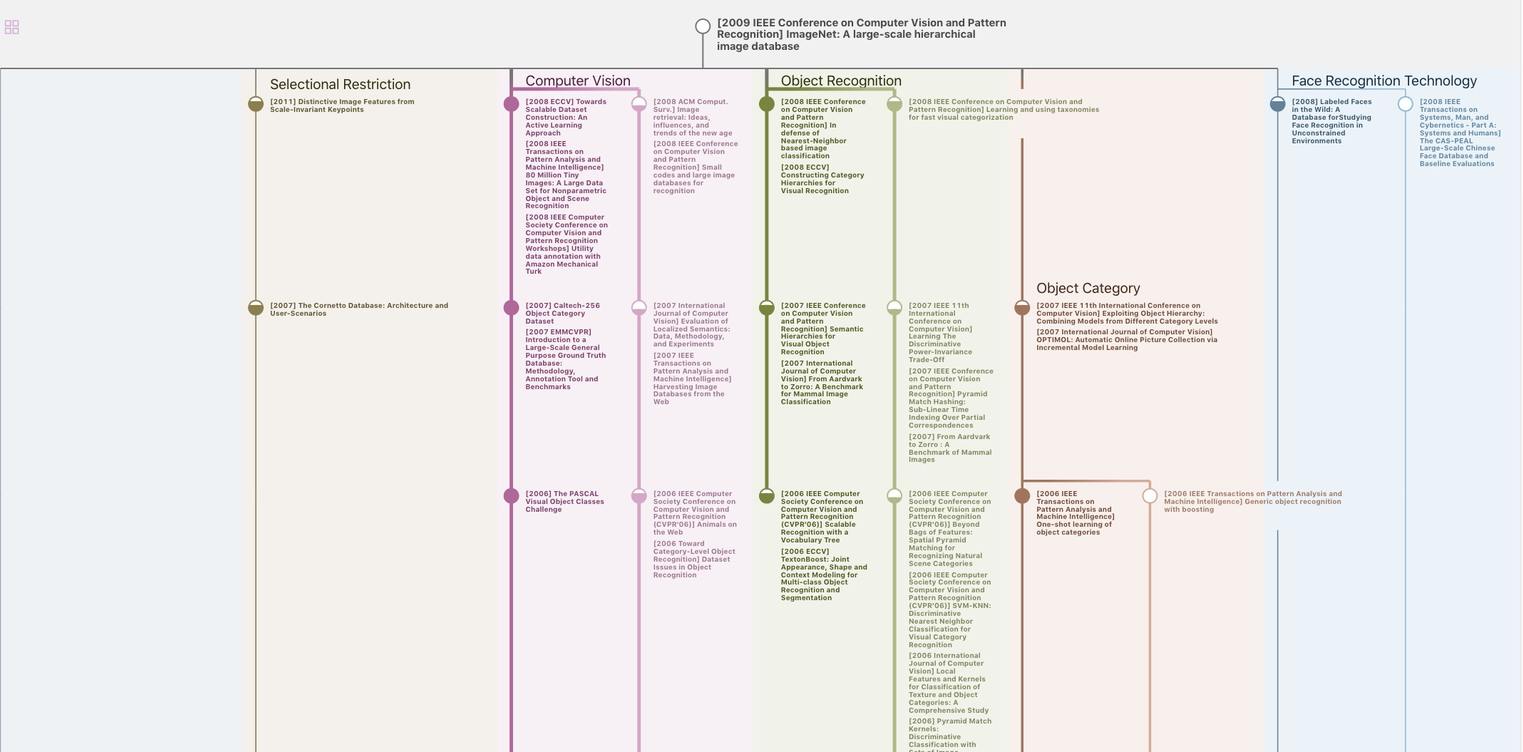
生成溯源树,研究论文发展脉络
Chat Paper
正在生成论文摘要