Genetic Programming With Flexible Region Detection for Fine-Grained Image Classification
IEEE Transactions on Evolutionary Computation(2024)
摘要
Fine-grained image classification (FGIC) is an important computer vision task with many real-world applications. However, FGIC is challenging due to intra-class variations and inter-class similarities, especially when there is limited training data. To address these challenges, a new genetic programming approach with flexible region detection, GP-RD, is proposed for different FGIC tasks, i.e., flower and fish classification tasks. The proposed GP-RD approach can automatically highlight the object, detect regions of interest, extract effective features, and combine global, local, and/or color features for classification. The performance of GP-RD is evaluated on flower and fish classification tasks within the FGIC domain, utilizing datasets with varying classes. In comparison with seven benchmark methods, GP-RD achieves significantly better performance in most comparisons. Further analysis demonstrates the interpretability, effectiveness, and efficiency of the proposed approach.
更多查看译文
关键词
Genetic Programming,Region Detection,Fine-Grained Image Classification,Feature Extraction
AI 理解论文
溯源树
样例
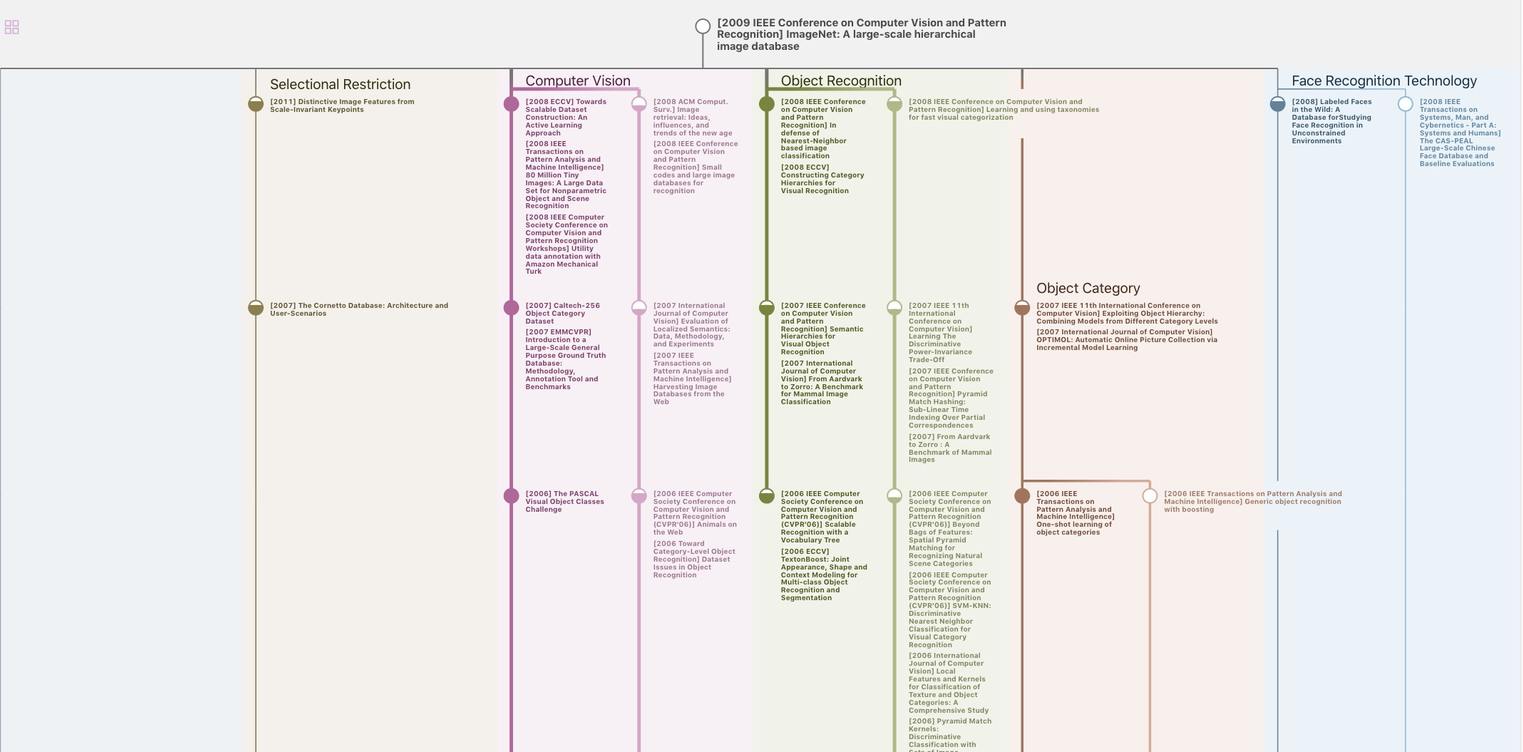
生成溯源树,研究论文发展脉络
Chat Paper
正在生成论文摘要