Global Convolutional Self-Action Module for Fast Brain Tumor Image Segmentation
IEEE TRANSACTIONS ON EMERGING TOPICS IN COMPUTATIONAL INTELLIGENCE(2024)
摘要
Integrating frameworks of Fermi normalization and fast data density functional transform (fDDFT), we established a new global convolutional self-action module to reduce the computational complexity in modern deep convolutional neural networks (CNNs). The Fermi normalization conflates mathematical properties of sigmoid function and z-score normalization with high efficiency. Global convolutional kernels embedded in the fDDFT simultaneously extract global features from whole input images through long-range dependency. The fDDFT endows the transformed images with a smoothness property, so the images can be substantially down-sampled before the global convolutions and then resized back to the original dimensions without losing accuracy. To inspect the feasibility of the synergy of Fermi normalization and fDDFT and the combinational effect with modern CNNs, we applied the dimension-fusion U-Net as a backbone and utilized the datasets from BraTS 2020. Experimental results exhibited that the model embedded with the module saved 57%-60% computational costs and raised 50%-53% inferencing speeds compared to the naive D-UNet model. Furthermore, the module enhanced the accuracy of brain tumor image segmentation. The dice scores of the work are 0.9221 for whole tumors, 0.8760 for tumor cores, 0.8659 for enhancing tumors, and 0.8362 for peritumoral edema. These results exhibit comparable performance to the winner of BraTS 2020. Our results also validate that image inputs processed by the module provide aligned and unified bases, establishing a specific space with optimized feature map combinations to reduce computational complexity efficiently. The module significantly boosted the performance of training and inferencing without losing model accuracy.
更多查看译文
关键词
Image segmentation,Tumors,Feature extraction,Brain modeling,Transforms,Computer architecture,Computational modeling,Fast data density functional transform,fermi normalization,global convolutions,long-range dependency,self-action
AI 理解论文
溯源树
样例
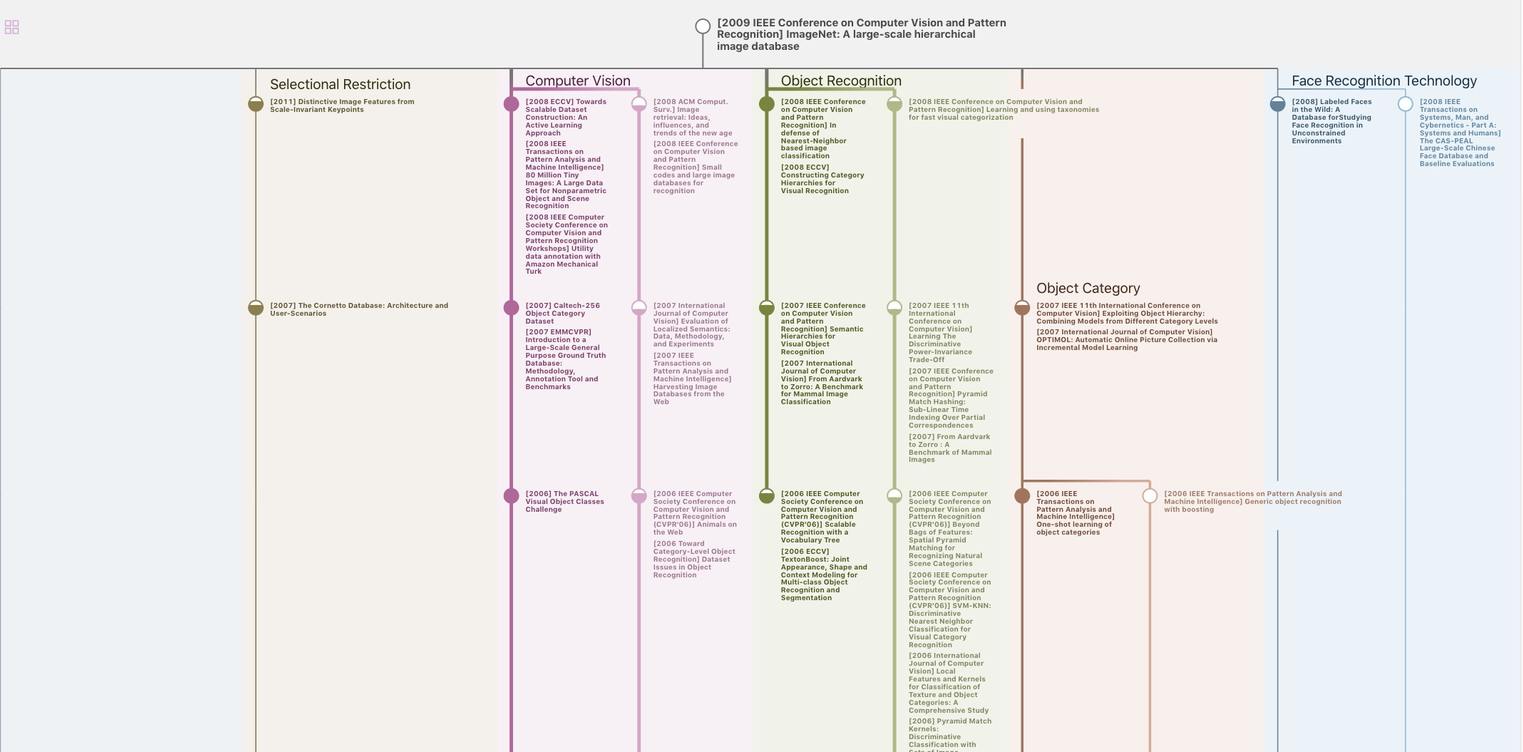
生成溯源树,研究论文发展脉络
Chat Paper
正在生成论文摘要