Illuminating the Black Box: A Comparative Study of Explainable AI for Interpreting Time Series Data in Vehicle Power Net Consumption Models.
International Conference on Machine Learning and Applications(2023)
摘要
Energy efficiency is crucial for the quick and widespread adoption of electric-powered vehicles because higher energy consumption results in a reduced electrical range. To leverage energy efficiency potentials in the low-volt power net of the vehicle complex and intertwined software-hardware interde-pendencies as well as operation strategies need to be optimized by the engineers. Since these systemic dependencies are not transparent and the amount of information exchanged among power consumers is high, a data-driven approach for root-cause explanation and analysis is investigated. We thus propose to make use of in -car communication data to determine the function-related causes of energy consumption of control units and their actuators with the help of Explainable AI (XAI). Therefore, we apply a step-wise experimental procedure validating the suitability of XAI methods with a virtual, thus transparent power consumer first before applying them to real-world automotive data. We demonstrate the suitability of four selected XAI methods for power consumers of the electrical system of a passenger car attaining acceptable results in terms of quality and efficiency. This expedites automated and reliable data-driven knowledge generation in the domain of automotive energy efficiency.
更多查看译文
关键词
artificial intelligence,data science,explainable artificial intelligence,automotive electronics,electronic control unit,energy efficiency,power consumption prediction
AI 理解论文
溯源树
样例
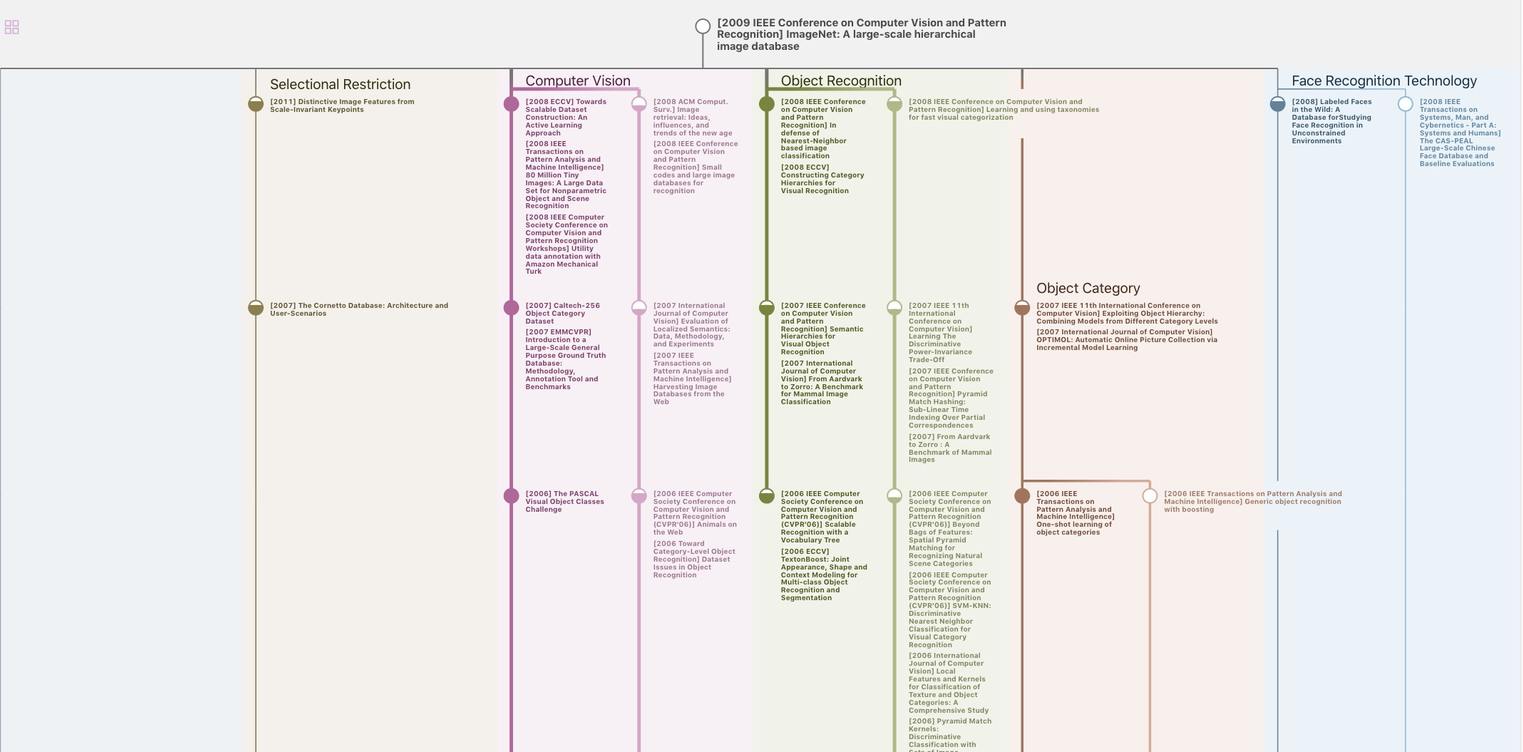
生成溯源树,研究论文发展脉络
Chat Paper
正在生成论文摘要