Federated Ophthalmic Disease Diagnosis Based on Weighted Averaging and Local Optimization
2023 China Automation Congress (CAC)(2023)
摘要
Deep learning and computer vision (CV) can easily recognize pictures that the human doctor would have to spend a lot of time and labor to process. Thus, they have a wide range of applications in the field of ophthalmology, which is highly dependent on fundus images. However, training a robust and highly accurate model requires a large number of labeled high-quality images, which is often difficult for a single institution to provide. A natural solution is to use data from multiple medical centers to train the model. But due to privacy protection, data heterogeneity, and other problems, these data cannot be shared directly. Based on a real case of ophthalmic disease diagnosis with multiple data sources, this paper proposes solutions to the heterogeneity of data amount and features between datasets, establishes a heterogeneous federated learning framework, and applies federated learning to the diagnosis of multiple types of ophthalmic diseases for the first time. The experimental results show that federated learning can greatly improve the diagnostic accuracy of clients with less data, and the accuracy of clients with more data can also be slightly improved. The ablation experiment has demonstrated the effectiveness of the proposed method for handling data heterogeneity. Our research shows that through heterogeneous federated learning, we can use multi-source, non-independent-identical data to build accurate disease diagnosis models, and make clients who cannot obtain effective models get a practical level disease diagnosis model.
更多查看译文
关键词
Ophthalmic Disease Diagnosis,Federated Learning,Data Heterogeneity
AI 理解论文
溯源树
样例
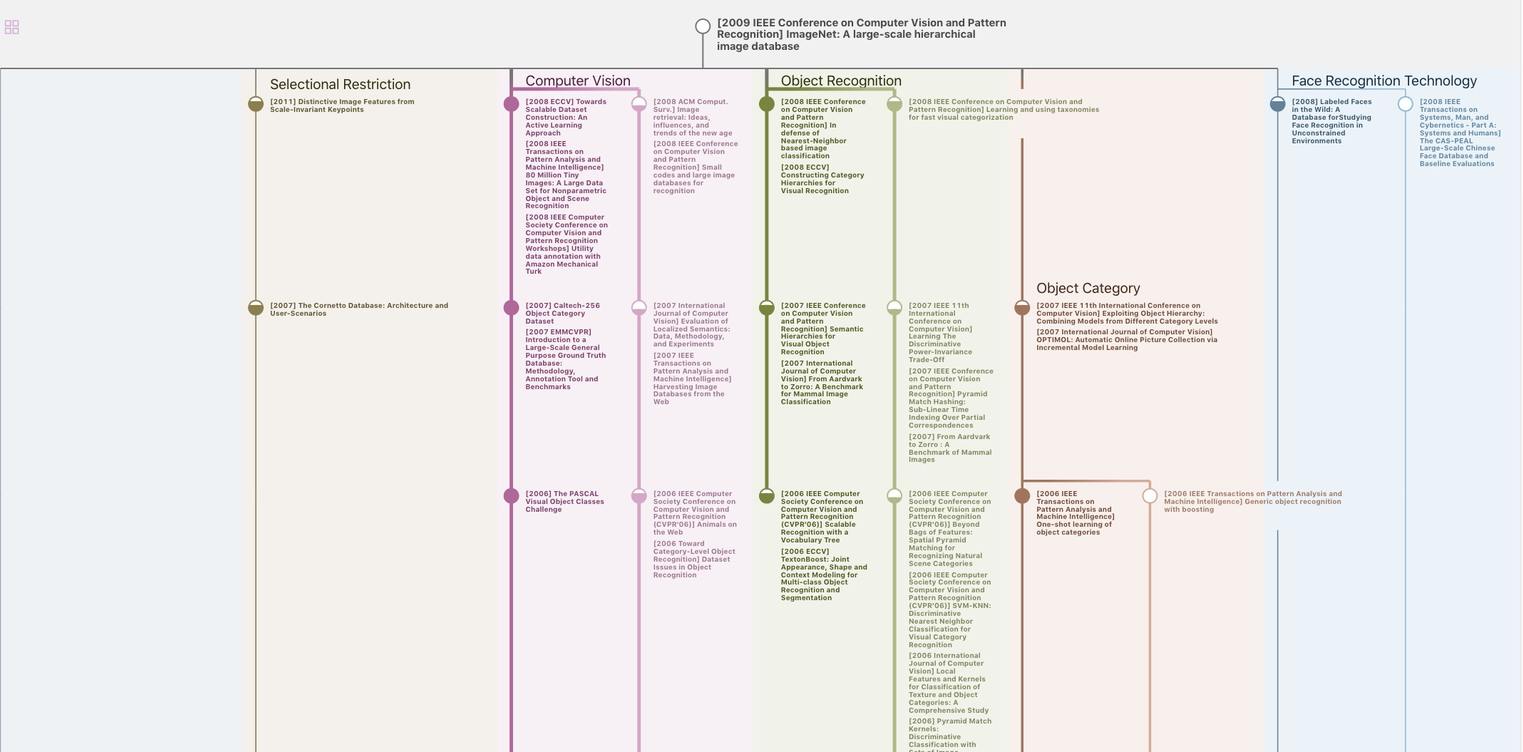
生成溯源树,研究论文发展脉络
Chat Paper
正在生成论文摘要