Forecasting Critical Overloads based on Heterogeneous Smart Grid Simulation.
International Conference on Machine Learning and Applications(2023)
摘要
Climate change mitigation poses a great challenge for our society. The need to reduce greenhouse gas emissions facilitates the expansion of renewable energy sources and electromobility. This transition is an already ongoing process, and with the worldwide increasing energy consumption, we face the need for automatic control and monitoring of the future electrical grid. To ensure a calculable and stable Low Voltage grid we need reliable load forecasting in order to avoid critical overloads and potential financial losses. This paper presents a novel concept for forecasting critical overloads based on an LSTM recurrent neural network. Our algorithm was tested using a one-year simulation of a rural Low Voltage grid section containing a grid-friendly energy community. Our results show the successful detection of 29 overloads within 12 simulated weeks. We reach a recall of 100% and a precision of 85%. Furthermore, we proved the ability of our LSTM to forecast two weeks with an MAE of 12.41 kW for the month of July. When optimizing the weather forecast data, we can lower this to 6.89 kW.
更多查看译文
关键词
Smart Grids,Load Forecasting,Energy Community,Simulation,Deep Learning
AI 理解论文
溯源树
样例
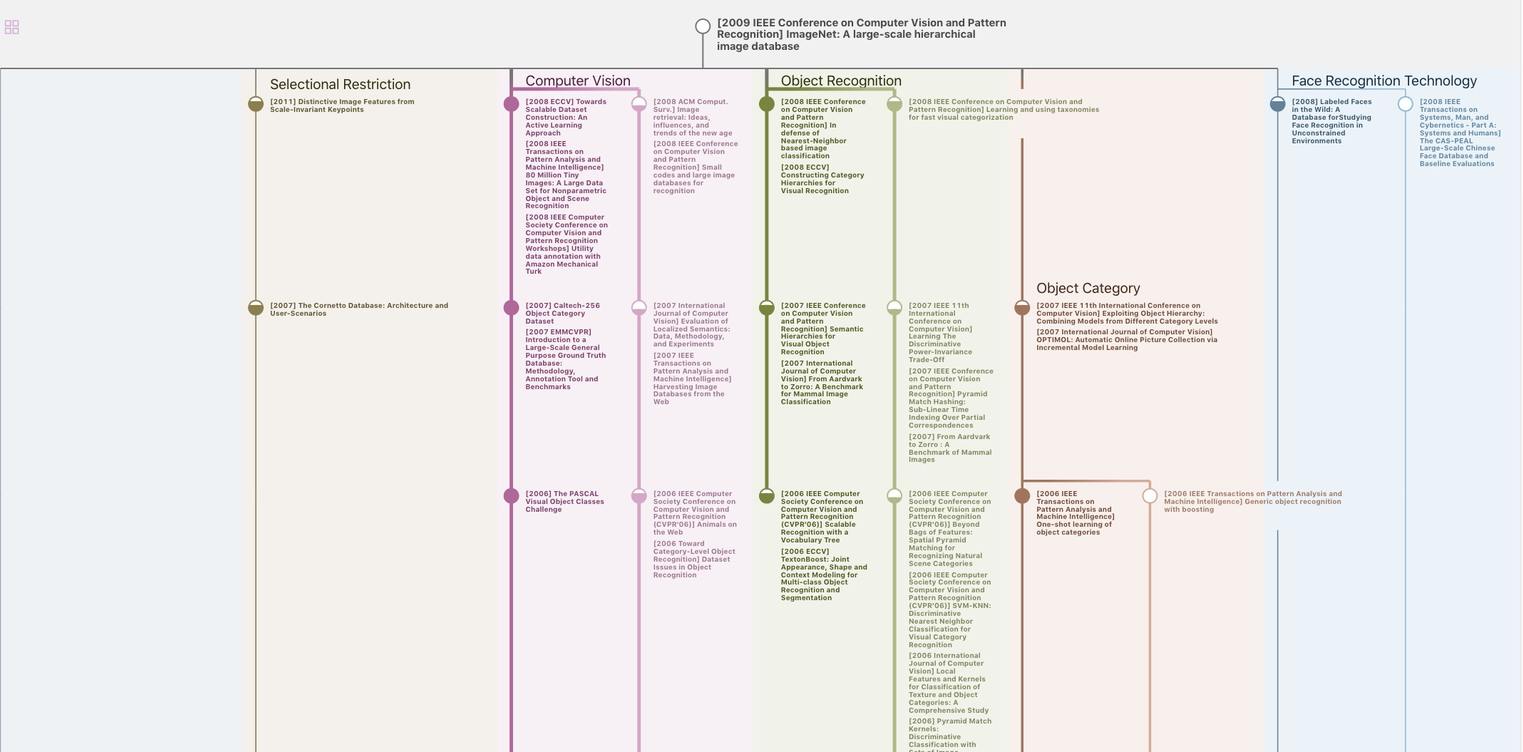
生成溯源树,研究论文发展脉络
Chat Paper
正在生成论文摘要