An Interpretable Deep Learning Approach for Bearing Remaining Useful Life
2023 China Automation Congress (CAC)(2023)
摘要
Deep learning has achieved excellent performance in bearing life prediction. However, compared to traditional methods that improve performance while sacrificing interpretability, it has brought about many unknown issues. To address the aforementioned problems, the patch and positional transformer recurrent neural network (PPTRNN) method takes center stage. Firstly, it employs the patch mask mechanism and the transformer to visualize the attention maps of deep models in bearing life prediction. Nextly, the model's expressive power concerning periodic signal position offsets is enhanced through periodic positional encoding. Under these circumstances, the results of bearing life prediction on the XJTU-SY dataset validate that the proposed PPTRNN method outperforms other approaches. The visualization of PPTRNN effectively distinguishes features from different life intervals. Furthermore, the attention maps explain the model's attention levels to signals at various positions over the entire lifespan, providing partial insights into the principles behind the deep model's perception.
更多查看译文
关键词
PPTRNN,self-attention,deep learning,RUL,interpretability
AI 理解论文
溯源树
样例
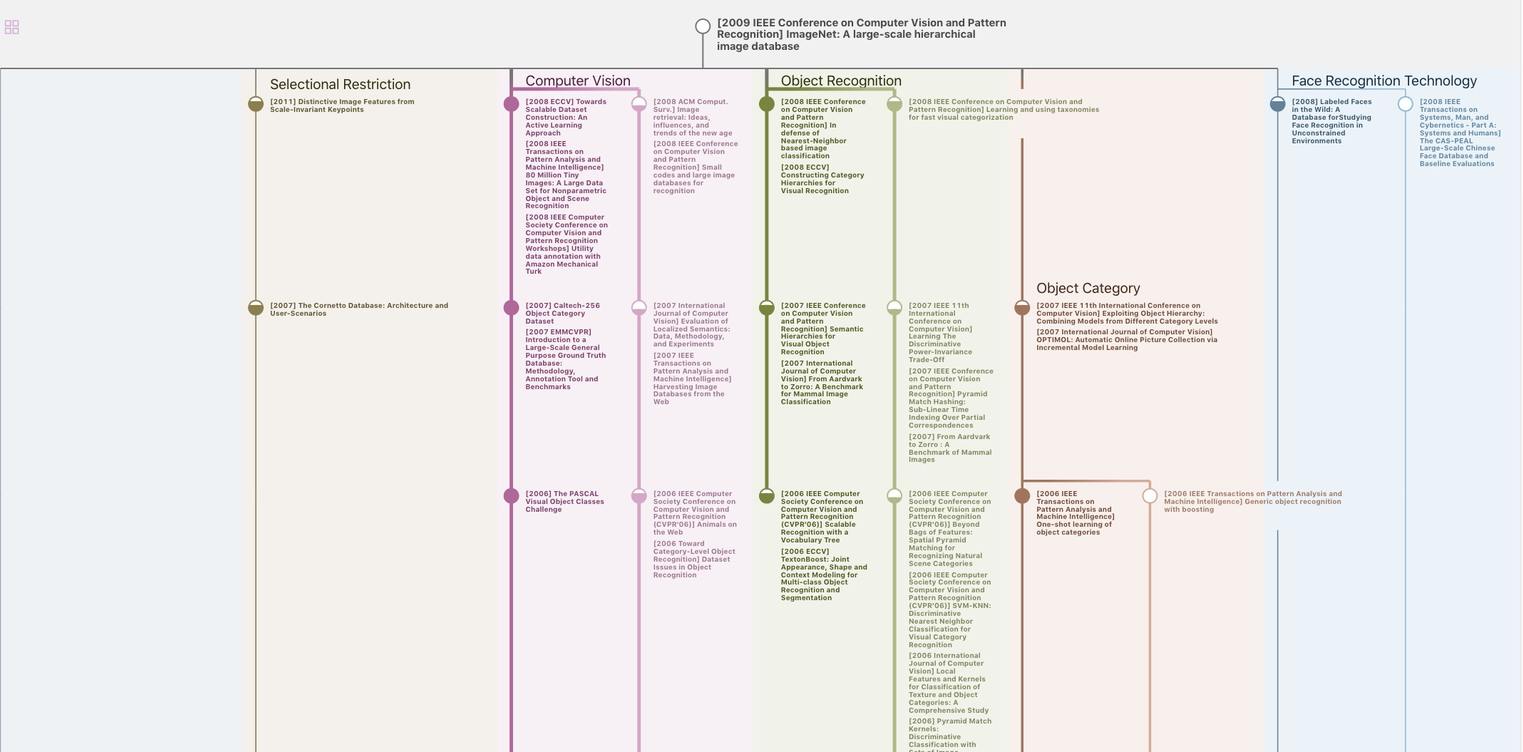
生成溯源树,研究论文发展脉络
Chat Paper
正在生成论文摘要