A multi-scale persistent spatiotemporal transformer for long-term urban traffic flow prediction
Journal of Electronic Science and Technology(2024)
摘要
Long-term urban traffic flow prediction is an important task in the field of intelligent transportation, as it can help optimize traffic management and improve travel efficiency. To improve prediction accuracy, a crucial issue is how to model spatiotemporal dependency in urban traffic data. In recent years, many studies have adopted spatiotemporal neural networks to extract key information from traffic data. However, most models ignore the semantic spatial similarity between long-distance areas when mining spatial dependency. They also ignore the impact of predicted time steps on the next unpredicted time step for making long-term predictions. Moreover, these models lack a comprehensive data embedding process to represent complex spatiotemporal dependency. This paper proposes a multi-scale persistent spatiotemporal transformer (MSPSTT) model to perform accurate long-term traffic flow prediction in cities. MSPSTT adopts an encoder-decoder structure and incorporates temporal, periodic, and spatial features to fully embed urban traffic data to address these issues. The model consists of a spatiotemporal encoder and a spatiotemporal decoder, which rely on temporal, geospatial, and semantic space multi-head attention modules to dynamically extract temporal, geospatial, and semantic characteristics. The spatiotemporal decoder combines the context information provided by the encoder, integrates the predicted time step information, and is iteratively updated to learn the correlation between different time steps in the broader time range to improve the model's accuracy for long-term prediction. Experiments on four public transportation datasets demonstrate that MSPSTT outperforms the existing models by up to 9.5% on three common metrics.
更多查看译文
关键词
Graph neural network,Multi-head attention mechanism,Spatio-temporal dependency,Traffic flow prediction
AI 理解论文
溯源树
样例
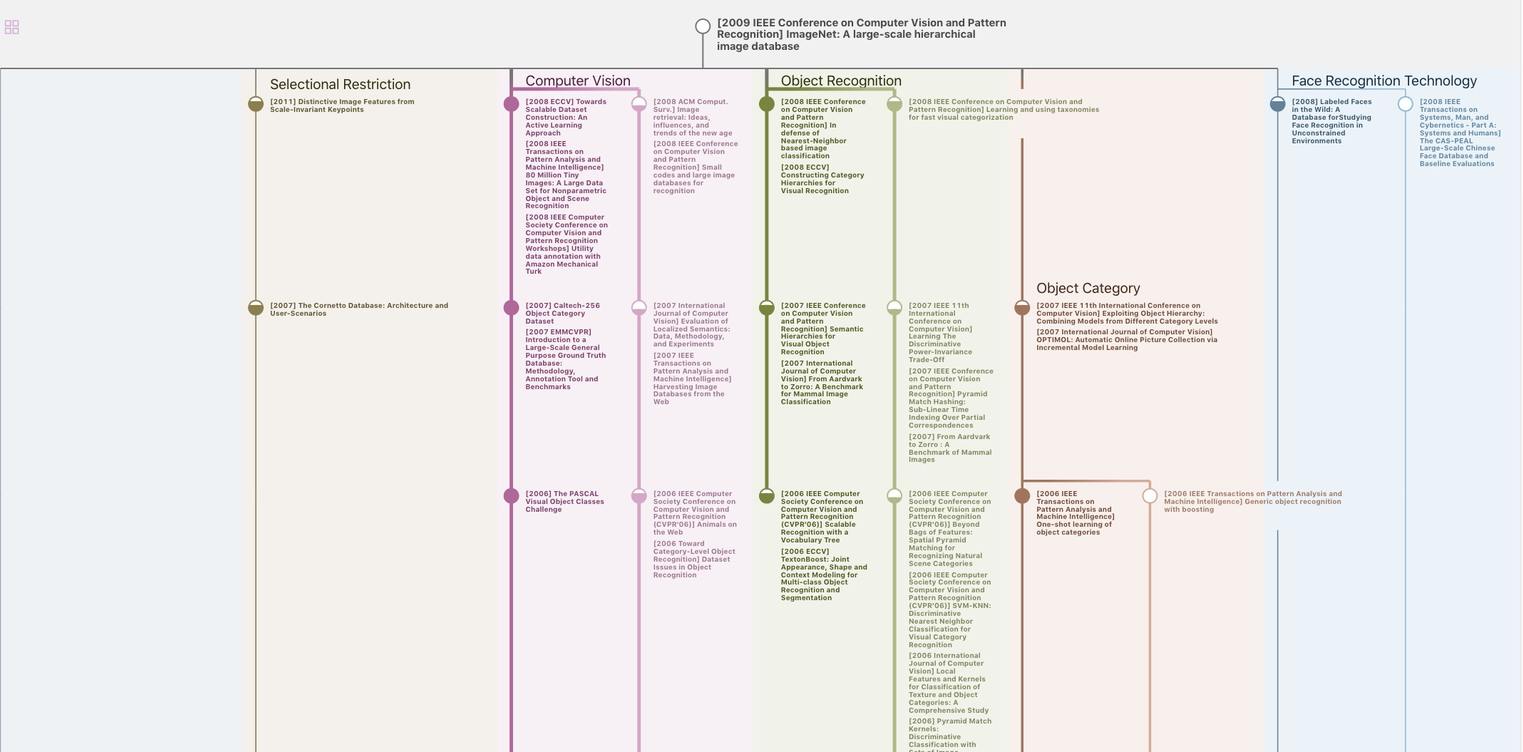
生成溯源树,研究论文发展脉络
Chat Paper
正在生成论文摘要