Adamer: Adapting Transformer Modules For Training-Efficient Image Restoration
2023 China Automation Congress (CAC)(2023)
摘要
Recently, Transformer model shines in the field of image restoration due to its unique global modeling capabilities. However, as Transformer models get larger and larger, training from scratch becomes expensive on some edge devices. In addition, due to the low computing power of edge devices, complete finetuning of the Transformer model becomes unaffordable (becomes very expensive). In our paper, we innovatively utilize the already trained partially structured network to Adapt pretrained Transformer modules (Adamer) for training-efficient image restoration. We froze some weights and introduced fast and memory-friendly Adapters. We propose Adaptation Transformer Block (ATB) to fusion of local information from CNN and global information from MDTA. Adamer achieving performance comparable to prior arts with lower computing resources in some major image restoration tasks, including image deraining, image deblurring, and image denoising.
更多查看译文
关键词
Image Restoration,Parameter-Efficient Fine-tuning,Transformer
AI 理解论文
溯源树
样例
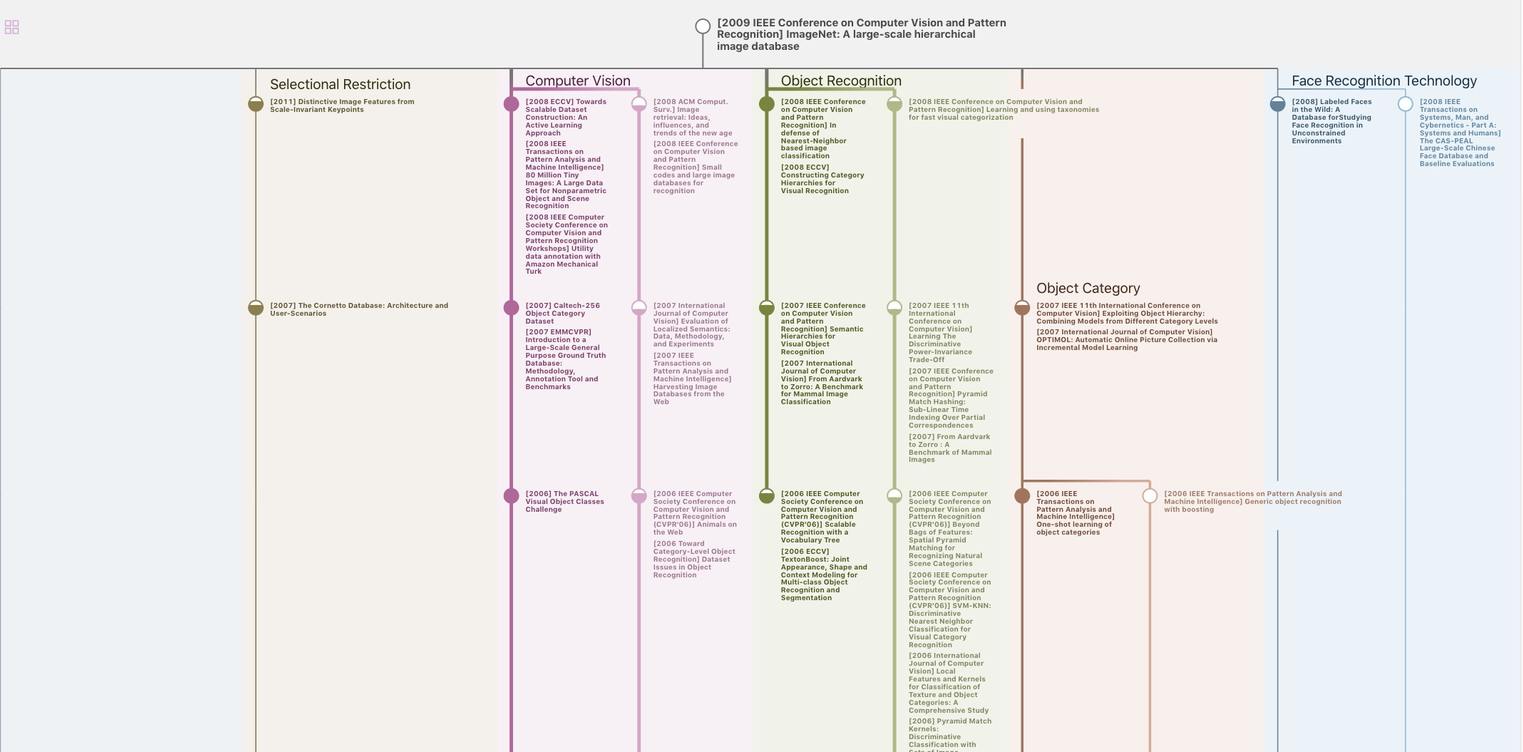
生成溯源树,研究论文发展脉络
Chat Paper
正在生成论文摘要