Sparse Basis Approach for Lightweight AI System Design.
International Conference on Electronics, Information and Communications(2024)
摘要
In recent years, the demand for image processing tasks has increasingly been delegated to AI, with Convolutional Neural Network (CNN) being a commonly used model for image processing. The convolution operation within CNN involves extensive computation, leading to amount of time requirements. This paper introduces an optimization algorithm specifically designed for the convolution operation in CNN models. The comparison is made between the conventional convolution method and the proposed sparse basis approach method, evaluating the required number of operations and data storage for each. The experiment utilizes Google's CFU playground platform to establish a VexRiscV CPU operating at a frequency of 200MHz for profiling the sparse basis approach algorithm. This profiling aids in determining whether to do software/hardware partitioning. The algorithm proposed in this paper is applicable to various CNN models, including LeNet, AlexNet, VGG16, VGG19, and others. Furthermore, this paper introduces dataflow analysis for the optimized convolution operation to provide effective reconfigurable support across different CNN models. The importance of dataflow in hardware modeling is discussed, along with a comparison of the impact of different dataflow code implementations on CPU execution. In contrast to traditional behavioral code profiling, profiling dataflow code allows for a more accurate measurement of the intrinsic complexity of the algorithm.
更多查看译文
关键词
Sparse matrix,Convolution,Dataflow,CFU playground
AI 理解论文
溯源树
样例
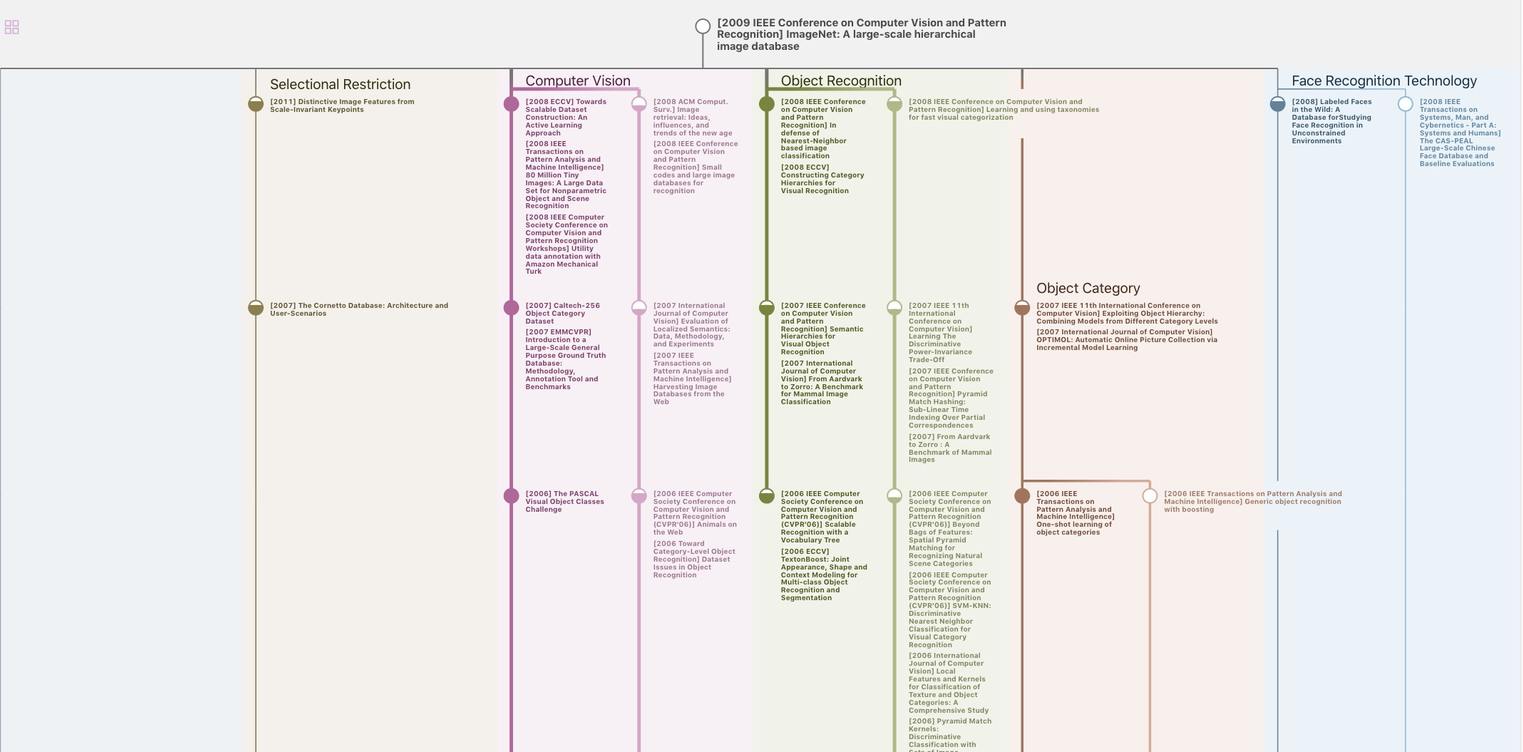
生成溯源树,研究论文发展脉络
Chat Paper
正在生成论文摘要