Caveline Detection at the Edge for Autonomous Underwater Cave Exploration and Mapping.
International Conference on Machine Learning and Applications(2023)
摘要
This paper explores the problem of deploying machine learning (ML)-based object detection and segmentation models on edge platforms to enable realtime caveline detection for Autonomous Underwater Vehicles (AUVs) used for under-water cave exploration and mapping. We specifically investigate three ML models, i.e., U-Net, Vision Transformer (ViT), and YOLOv8, deployed on three edge platforms: Raspberry Pi-4, Intel Neural Compute Stick 2 (NCS2), and NVIDIA Jetson Nano. The experimental results unveil clear tradeoffs between model accuracy, processing speed, and energy consumption. The most accurate model has shown to be U-Net with an 85.53 F1-score and 85.38 Intersection Over Union (IoU) value. Meanwhile, the highest inference speed and lowest energy consumption are achieved by the YOLOv8 model deployed on Jetson Nano operating in the high-power and low-power modes, respectively. The comprehensive quantitative analyses and comparative results provided in the paper highlight important nuances that can guide the deployment of caveline detection systems on underwater robots for ensuring safe and reliable AUV navigation during underwater cave exploration and mapping missions.
更多查看译文
关键词
Edge Computing,Object Detection,Segmentation,Underwater Robots,Visual Servoing
AI 理解论文
溯源树
样例
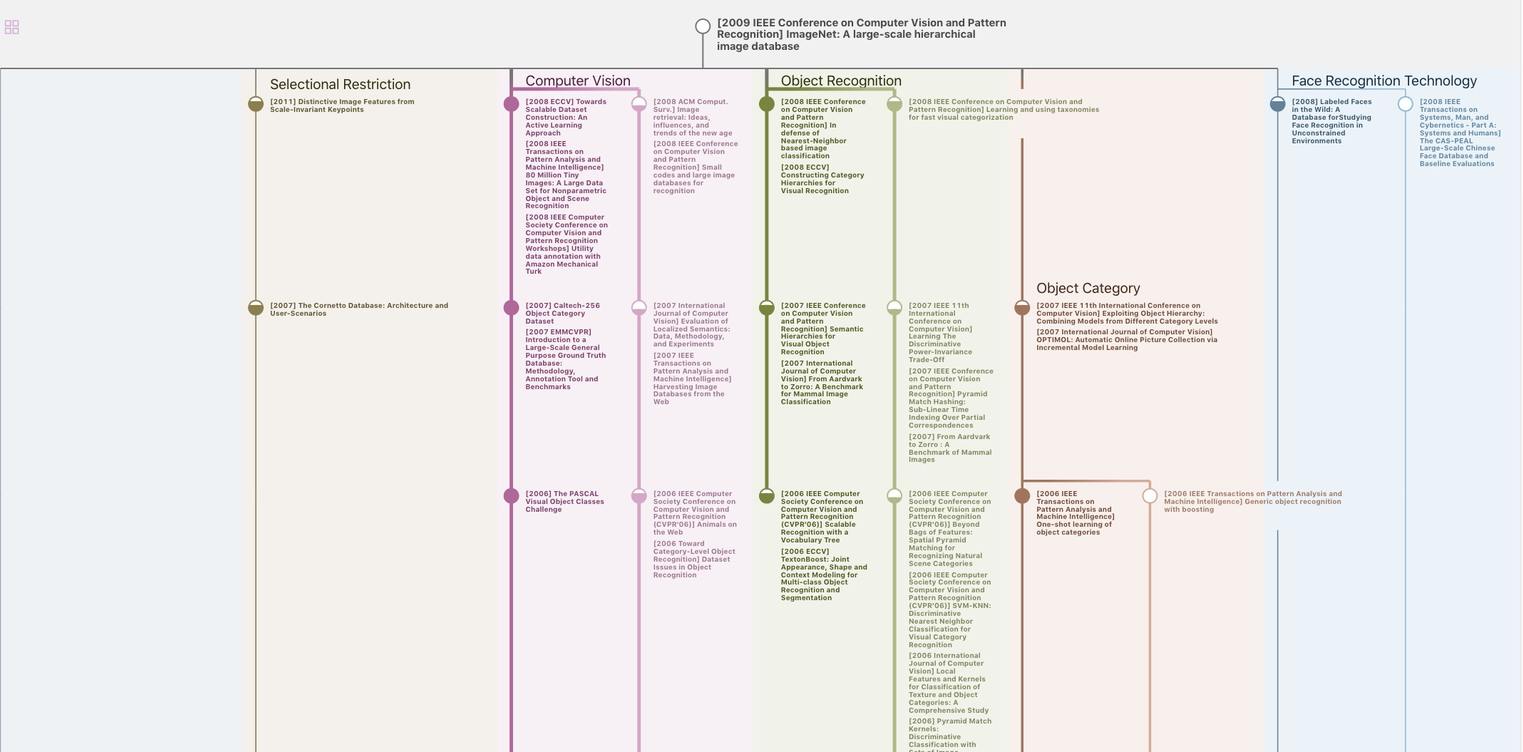
生成溯源树,研究论文发展脉络
Chat Paper
正在生成论文摘要