SCOF: Security-Aware Computation Offloading Using Federated Reinforcement Learning in Industrial Internet of Things with Edge Computing
IEEE Transactions on Services Computing(2024)
摘要
Industry 5.0 facilitates the intelligent upgrade of smart factories in Industrial Internet of Things (IIoT), and also introduces a plethora of data processing challenges. Mobile edge computing offloads data to edge servers for processing, easing the data processing pressure and reducing system cost. However, smart factories contain numerous sensitive information, and offloading them to edge servers directly may pose a risk of data leakage. To address these challenges, we investigate a localedge collaborative smart factory system. Specifically, we firstly model the tasks as a directed acyclic graph, and formulate the offloading problem as a Markov decision process, considering the optimization of latency, energy consumption and the number of overtime tasks. Then, we propose a security-aware computation offloading method using federated reinforcement learning in IIoT, named SCOF. SCOF employs federated learning, keeping data for local computation and uploading model parameters to edge servers for aggregation. The data transmission passes through an artificial noise channel to protect against eavesdropping. Meanwhile, SCOF utilizes differential privacy to protect data security and employs deep reinforcement learning for selecting near-optimal offloading decisions. Finally, abundant experiments are conducted under a real dataset. The results show that SCOF has better perfomance than the state-of-the-art baseline algorithms.
更多查看译文
关键词
Industrial Internet of Things,Mobile Edge Computing,Federated Learning,Differential Privacy,Deep Reinforcement Learning
AI 理解论文
溯源树
样例
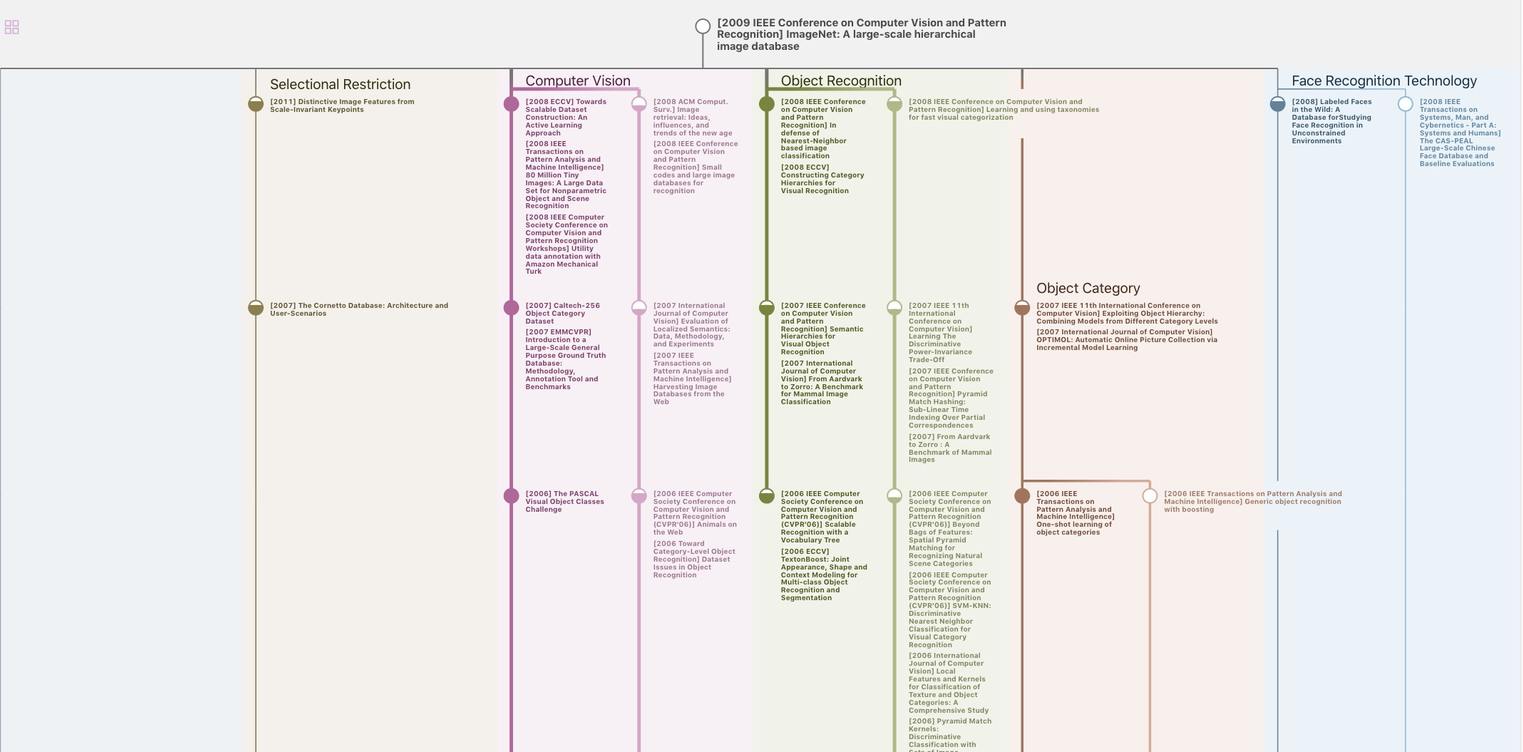
生成溯源树,研究论文发展脉络
Chat Paper
正在生成论文摘要