Adaptive Online Optimization Control Method Based on Reinforcement Learning
2023 China Automation Congress (CAC)(2023)
摘要
Large envelope deformable aircraft pose higher requirements for guidance and control technology, requiring control law parameters to be able to adaptively optimize online based on flight uncertainty and dynamically enhance flight control capabilities. At present, coefficient freezing is commonly used in engineering to design typical feature work points, but in large envelope flight, there are more and more feature work points, and traditional design methods are more complex and highly dependent on manual experience; On the other hand, traditional gain scheduling methods can only switch preset control laws based on flight status. Once the control laws are deployed, their performance is solidified, and their adaptive ability is relatively limited, making it impossible to perform online optimization. This article adopts deep reinforcement learning to design a universal PI state feedback control law parameter self-learning architecture, achieving autonomous learning of control law parameters at different working points; At the same time, the trained parameter adjustment network is deployed together with the control law, and online learning optimization of control law parameters is achieved during flight based on the control effect, enhancing adaptive ability. Finally, the effectiveness of the proposed method was verified using a specific pitch angle tracking control scenario as an example.
更多查看译文
关键词
Adaptive control,Online learning,Reinforcement learning,Deep learning
AI 理解论文
溯源树
样例
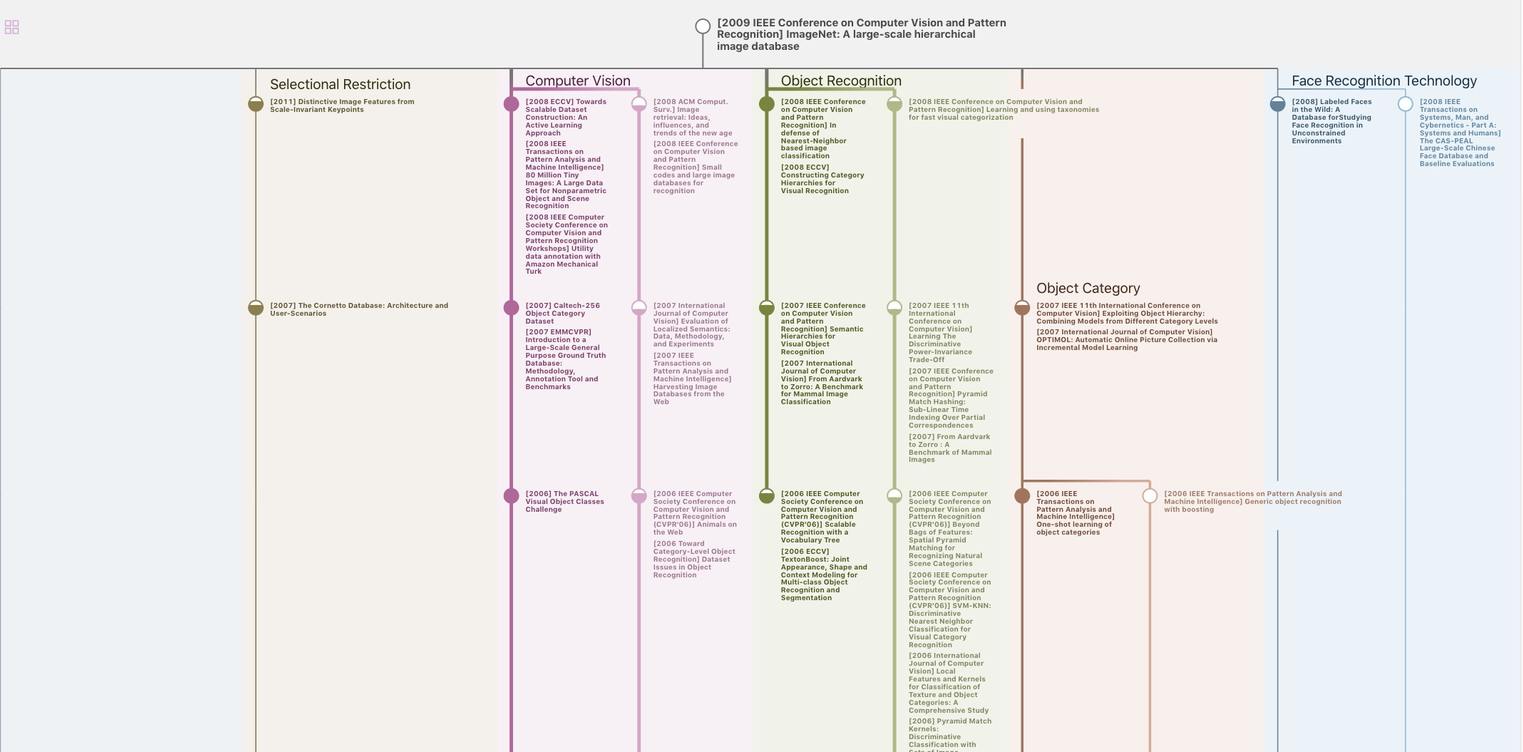
生成溯源树,研究论文发展脉络
Chat Paper
正在生成论文摘要