Scalable QoS-Aware Multipath Routing in Hybrid Knowledge-Defined Networking with Multi-Agent Deep Reinforcement Learning
IEEE Transactions on Mobile Computing(2024)
摘要
Multipath routing remains a challenging issue in traffic engineering (TE) as existing solutions are incapable of handling the evolving network dynamics and stringent quality-of-service (QoS) requirements. To address it, multi-agent deep reinforcement learning (MADRL) is a promising technique that provides more elaborate multipath routing strategies. However, prevalent MADRL-based solutions still suffer inapplicability as they fail to ensure both scalability and QoS awareness. In this paper, we leverage the emerging hybrid knowledge-defined networking (KDN) architecture, and propose a collaborative MADRL-based multipath routing algorithm. Two novel mechanisms, i.e., parallel agent replication and periodic policy synchronization, are devised for agent design to ensure the practicality of the proposed method. In addition, an efficient communication mechanism is established to facilitate multi-agent collaboration by enabling scalable observation and reward exchange. Featuring a multi-agent twin-actor-critic (MA-TAC) learning structure and a proximal policy optimization (PPO) -based training process, the proposed algorithm consists of alternately scheduled execution and training phases for practical deployment. We compare the performance of our proposed method with those of several benchmark methods. Extensive simulation results demonstrate that the proposed method achieves significantly better scalability, QoS awareness, and stability than the benchmark methods under various environment settings.
更多查看译文
关键词
Multi-agent deep reinforcement learning,traffic engineering,multipath routing,knowledge-defined networking
AI 理解论文
溯源树
样例
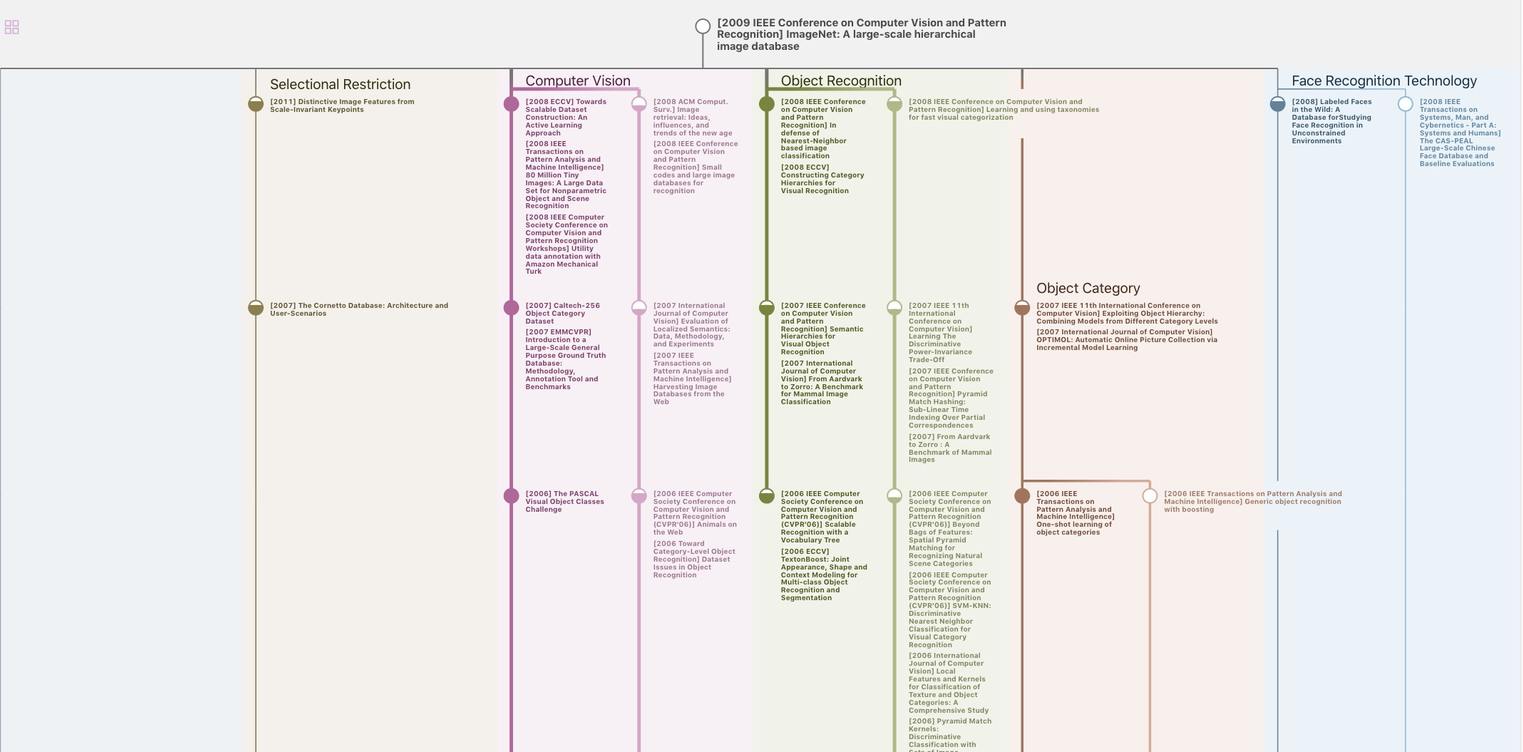
生成溯源树,研究论文发展脉络
Chat Paper
正在生成论文摘要