Adversarial Attacks on Video Quality Assessment Models
Knowledge-Based Systems(2024)
摘要
Most currently developed video quality assessment (VQA) algorithms have achieved excellent performance by using deep neural network (DNN). However, DNN is vulnerable to adversarial attacks, as an efficient surrogate for validating the model robustness, and there lack adversarial attack methods against VQA models. To this end, we propose a spatiotemporal attack network to generate adversarial examples for evaluating the robustness of VQA models that contains a spatial subnetwork and a temporal subnetwork. The proposed network, dubbed the Space-Time Quality Attack Network (STQA-Net1), first computes the just noticeable difference (JND) maps of a video sequence as the input of the spatial subnetwork. The spatial subnetwork encodes the computed maps as spatial features and feeds the spatial features to the temporal subnetwork. Then, the spatial features are fused with the output of the temporal subnetwork and the fused features are decoded as attack weight maps. A visual constraint is used to control the visibility of perturbations and guide the generation of perturbation maps by multiplying JND maps with attack weight maps. Finally, the generated perturbation maps are added to the original video to form an adversarial example. Further, we also try to design a two-branch network to generate two opposite examples in a targeted attack scenario. The proposed attack methods against six state-of-the-art VQA algorithms are thoroughly tested on three VQA databases. The experimental results show that the proposed attack methods are very effective for testing the robustness of VQA models.
更多查看译文
关键词
Video quality assessment,adversarial attack,visual constraint,spatiotemporal attack
AI 理解论文
溯源树
样例
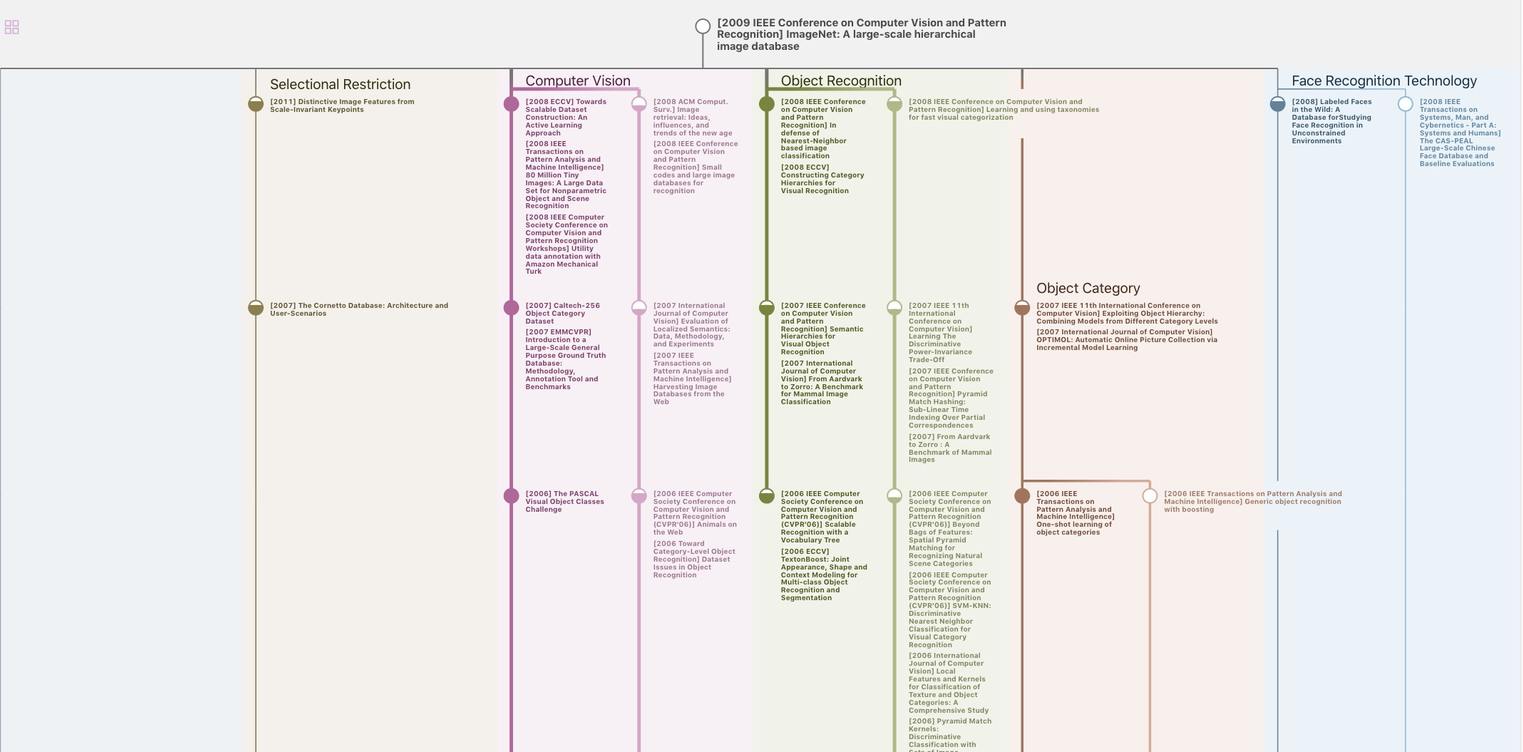
生成溯源树,研究论文发展脉络
Chat Paper
正在生成论文摘要