Equivariant Ensembles and Regularization for Reinforcement Learning in Map-based Path Planning
arxiv(2024)
摘要
In reinforcement learning (RL), exploiting environmental symmetries can
significantly enhance efficiency, robustness, and performance. However,
ensuring that the deep RL policy and value networks are respectively
equivariant and invariant to exploit these symmetries is a substantial
challenge. Related works try to design networks that are equivariant and
invariant by construction, limiting them to a very restricted library of
components, which in turn hampers the expressiveness of the networks. This
paper proposes a method to construct equivariant policies and invariant value
functions without specialized neural network components, which we term
equivariant ensembles. We further add a regularization term for adding
inductive bias during training. In a map-based path planning case study, we
show how equivariant ensembles and regularization benefit sample efficiency and
performance.
更多查看译文
AI 理解论文
溯源树
样例
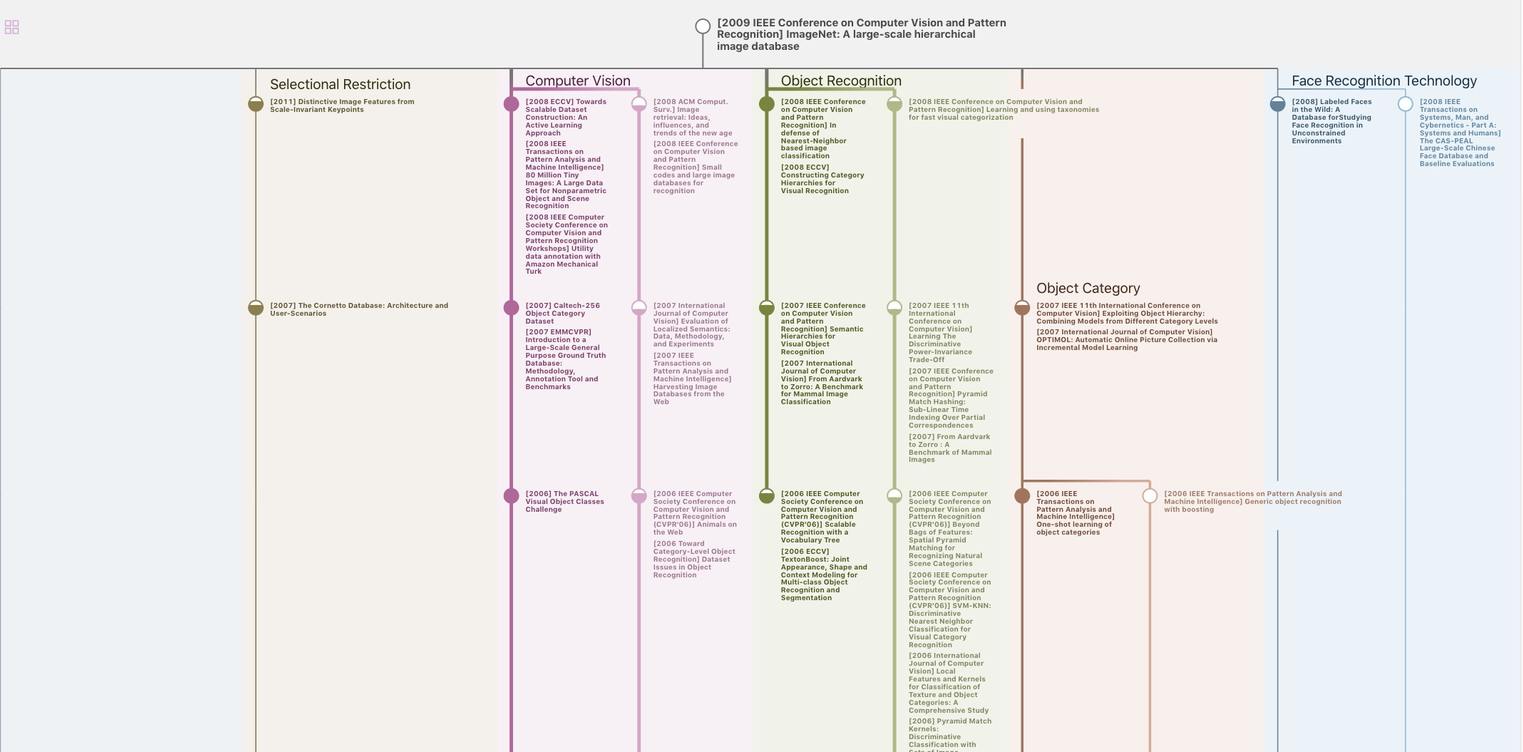
生成溯源树,研究论文发展脉络
Chat Paper
正在生成论文摘要