ViTGaze: Gaze Following with Interaction Features in Vision Transformers
arxiv(2024)
摘要
Gaze following aims to interpret human-scene interactions by predicting the
person's focal point of gaze. Prevailing approaches often use multi-modality
inputs, most of which adopt a two-stage framework. Hence their performance
highly depends on the previous prediction accuracy. Others use a
single-modality approach with complex decoders, increasing network
computational load. Inspired by the remarkable success of pre-trained plain
Vision Transformers (ViTs), we introduce a novel single-modality gaze following
framework, ViTGaze. In contrast to previous methods, ViTGaze creates a brand
new gaze following framework based mainly on powerful encoders (dec. param.
less than 1
within self-attention can be transferred to interactions between humans and
scenes. Leveraging this presumption, we formulate a framework consisting of a
4D interaction encoder and a 2D spatial guidance module to extract human-scene
interaction information from self-attention maps. Furthermore, our
investigation reveals that ViT with self-supervised pre-training exhibits an
enhanced ability to extract correlated information. A large number of
experiments have been conducted to demonstrate the performance of the proposed
method. Our method achieves state-of-the-art (SOTA) performance among all
single-modality methods (3.4
very comparable performance against multi-modality methods with 59
parameters less.
更多查看译文
AI 理解论文
溯源树
样例
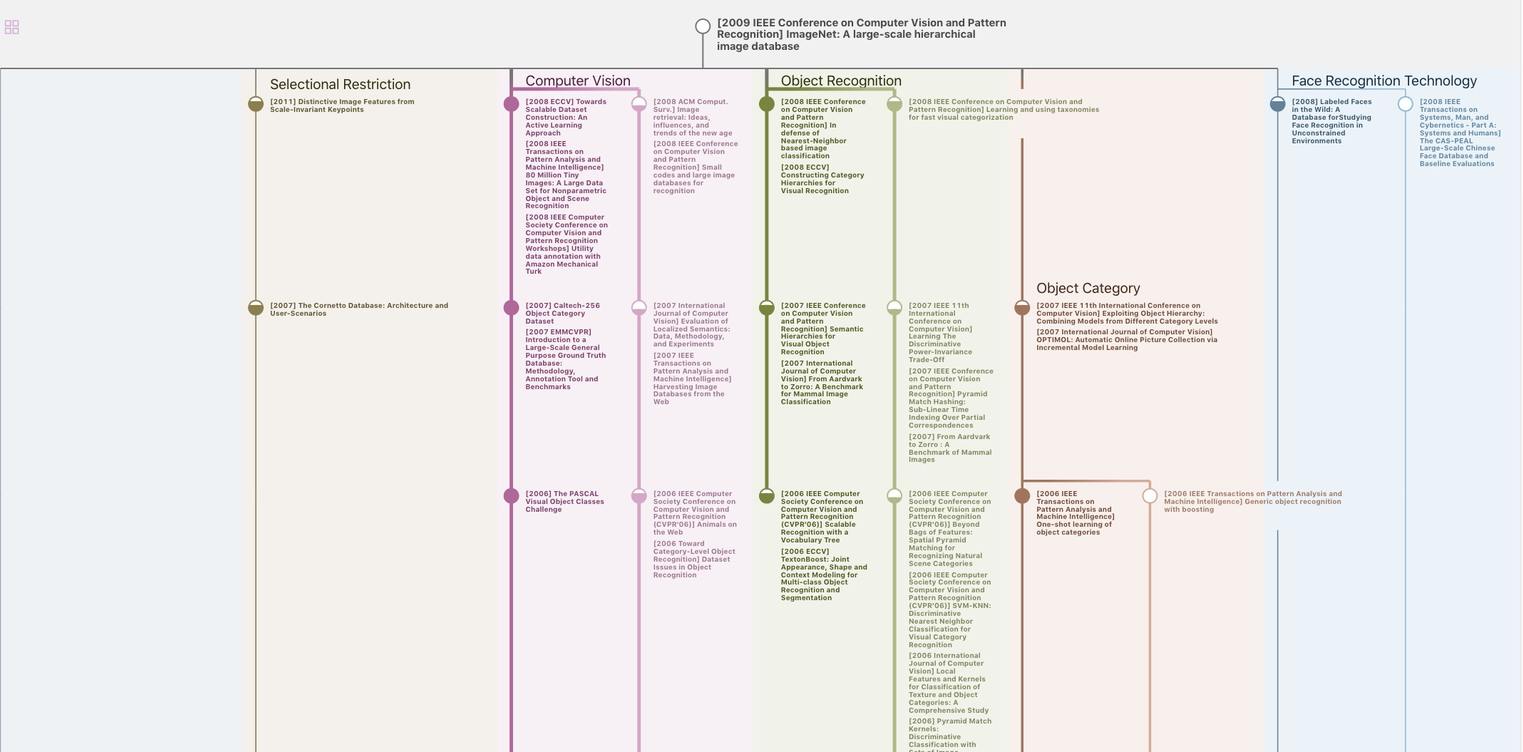
生成溯源树,研究论文发展脉络
Chat Paper
正在生成论文摘要