Hierarchical Digital Twin for Efficient 6G Network Orchestration via Adaptive Attribute Selection and Scalable Network Modeling
arxiv(2024)
摘要
Achieving a holistic and long-term understanding through accurate network
modeling is essential for orchestrating future networks with increasing service
diversity and infrastructure complexities. However, due to unselective data
collection and uniform processing, traditional modeling approaches undermine
the efficacy and timeliness of network orchestration. Additionally, temporal
disparities arising from various modeling delays further impair the centralized
decision-making with distributed models. In this paper, we propose a new
hierarchical digital twin paradigm adapting to real-time network situations for
problem-centered model construction. Specifically, we introduce an adaptive
attribute selection mechanism that evaluates the distinct modeling values of
diverse network attributes, considering their relevance to current network
scenarios and inherent modeling complexity. By prioritizing critical attributes
at higher layers, an efficient evaluation of network situations is achieved to
identify target areas. Subsequently, scalable network modeling facilitates the
inclusion of all identified elements at the lower layers, where more
fine-grained digital twins are developed to generate targeted solutions for
user association and power allocation. Furthermore, virtual-physical domain
synchronization is implemented to maintain accurate temporal alignment between
the digital twins and their physical counterparts, spanning from the
construction to the utilization of the proposed paradigm. Extensive simulations
validate the proposed approach, demonstrating its effectiveness in efficiently
identifying pressing issues and delivering network orchestration solutions in
complex 6G HetNets.
更多查看译文
AI 理解论文
溯源树
样例
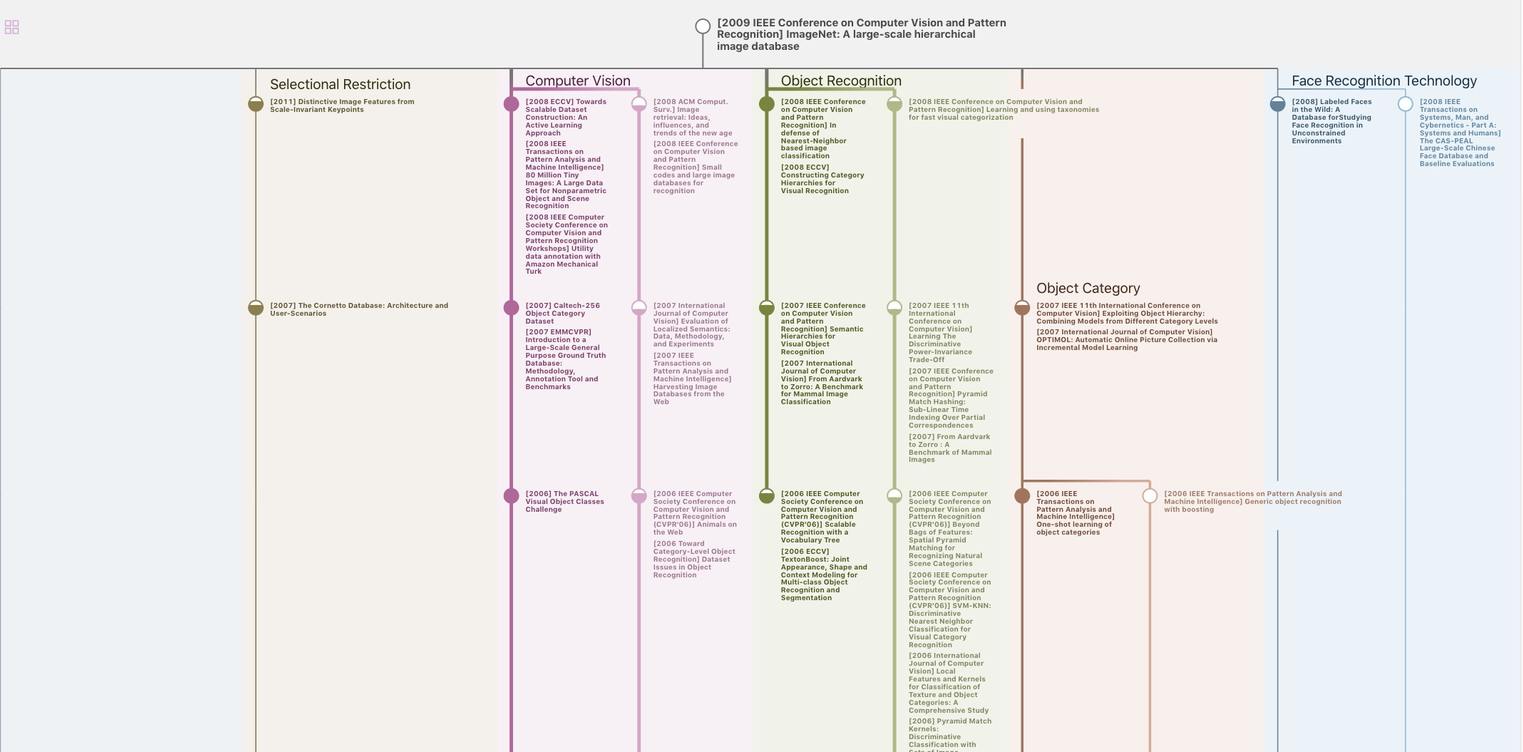
生成溯源树,研究论文发展脉络
Chat Paper
正在生成论文摘要