Reinforcement Learning from Delayed Observations via World Models
arxiv(2024)
摘要
In standard Reinforcement Learning settings, agents typically assume
immediate feedback about the effects of their actions after taking them.
However, in practice, this assumption may not hold true due to physical
constraints and can significantly impact the performance of RL algorithms. In
this paper, we focus on addressing observation delays in partially observable
environments. We propose leveraging world models, which have shown success in
integrating past observations and learning dynamics, to handle observation
delays. By reducing delayed POMDPs to delayed MDPs with world models, our
methods can effectively handle partial observability, where existing approaches
achieve sub-optimal performance or even degrade quickly as observability
decreases. Experiments suggest that one of our methods can outperform a naive
model-based approach by up to
input based delayed environment, for the first time showcasing delay-aware
reinforcement learning on visual observations.
更多查看译文
AI 理解论文
溯源树
样例
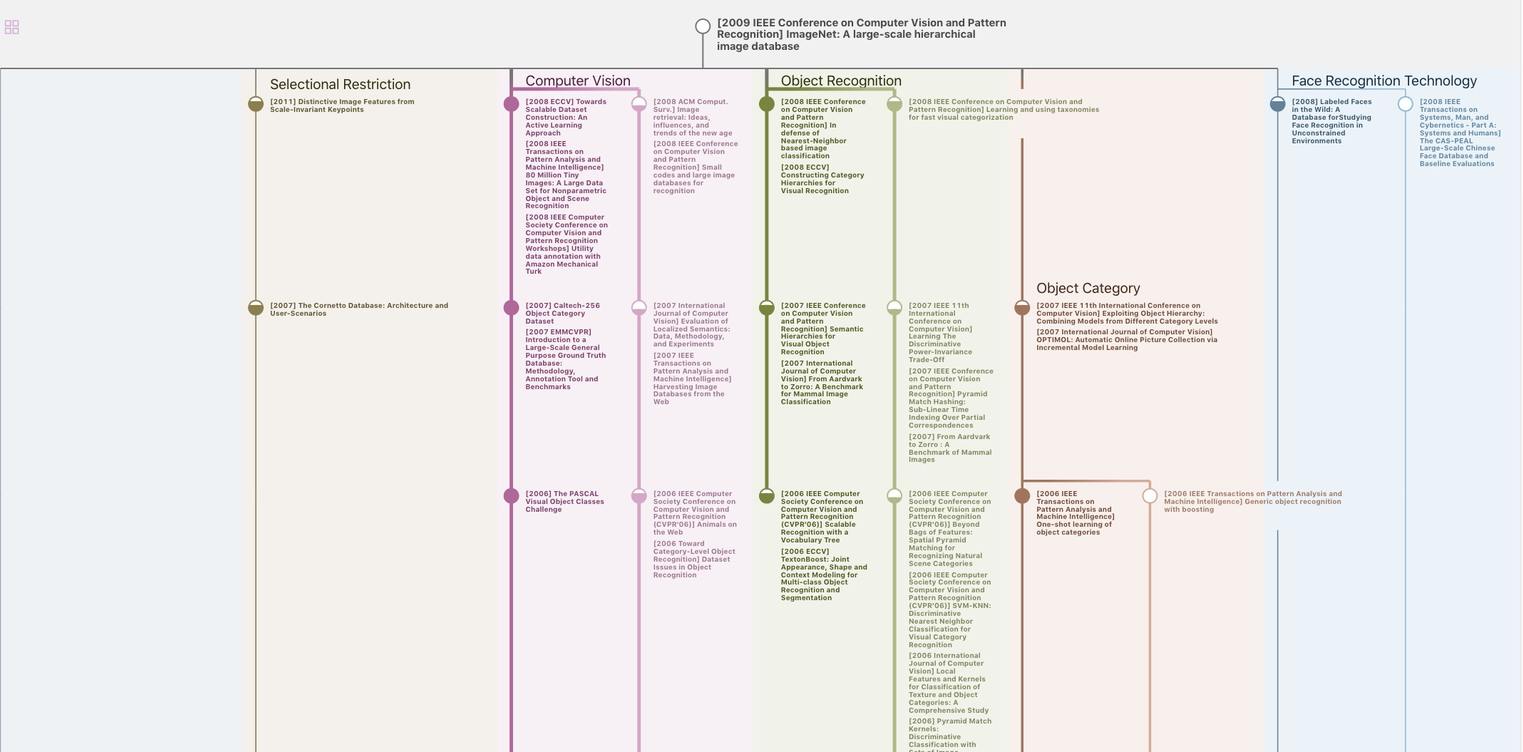
生成溯源树,研究论文发展脉络
Chat Paper
正在生成论文摘要