Neuron-centric Hebbian Learning
arxiv(2024)
摘要
One of the most striking capabilities behind the learning mechanisms of the
brain is the adaptation, through structural and functional plasticity, of its
synapses. While synapses have the fundamental role of transmitting information
across the brain, several studies show that it is the neuron activations that
produce changes on synapses. Yet, most plasticity models devised for artificial
Neural Networks (NNs), e.g., the ABCD rule, focus on synapses, rather than
neurons, therefore optimizing synaptic-specific Hebbian parameters. This
approach, however, increases the complexity of the optimization process since
each synapse is associated to multiple Hebbian parameters. To overcome this
limitation, we propose a novel plasticity model, called Neuron-centric Hebbian
Learning (NcHL), where optimization focuses on neuron- rather than
synaptic-specific Hebbian parameters. Compared to the ABCD rule, NcHL reduces
the parameters from 5W to 5N, being W and N the number of weights and
neurons, and usually N ≪ W. We also devise a "weightless" NcHL model, which
requires less memory by approximating the weights based on a record of neuron
activations. Our experiments on two robotic locomotion tasks reveal that NcHL
performs comparably to the ABCD rule, despite using up to ∼97 times less
parameters, thus allowing for scalable plasticity.
更多查看译文
AI 理解论文
溯源树
样例
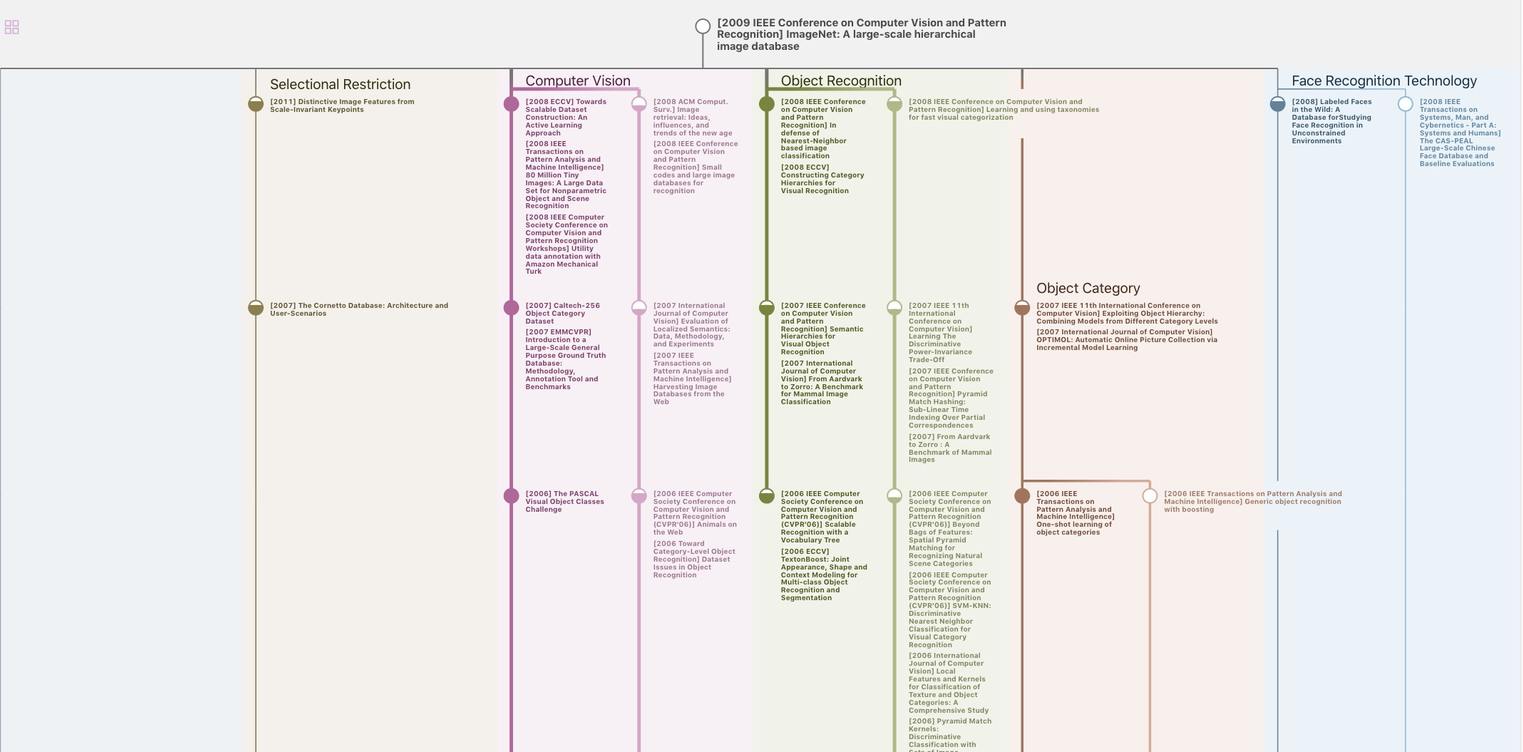
生成溯源树,研究论文发展脉络
Chat Paper
正在生成论文摘要