Fairness Evaluation for Uplift Modeling in the Absence of Ground Truth
arxiv(2024)
摘要
The acceleration in the adoption of AI-based automated decision-making
systems poses a challenge for evaluating the fairness of algorithmic decisions,
especially in the absence of ground truth. When designing interventions, uplift
modeling is used extensively to identify candidates that are likely to benefit
from treatment. However, these models remain particularly susceptible to
fairness evaluation due to the lack of ground truth on the outcome measure
since a candidate cannot be in both treatment and control simultaneously. In
this article, we propose a framework that overcomes the missing ground truth
problem by generating surrogates to serve as a proxy for counterfactual labels
of uplift modeling campaigns. We then leverage the surrogate ground truth to
conduct a more comprehensive binary fairness evaluation. We show how to apply
the approach in a comprehensive study from a real-world marketing campaign for
promotional offers and demonstrate its enhancement for fairness evaluation.
更多查看译文
AI 理解论文
溯源树
样例
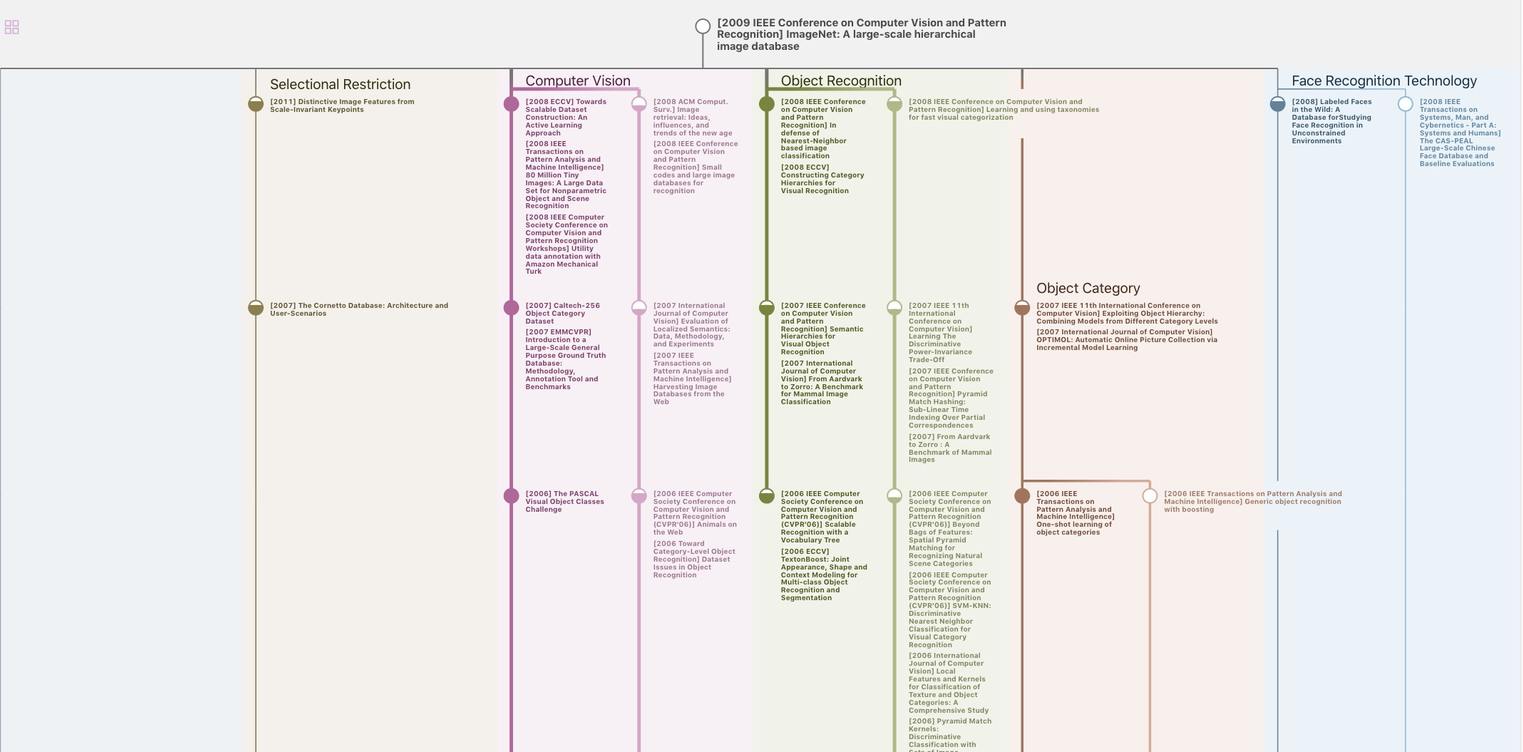
生成溯源树,研究论文发展脉络
Chat Paper
正在生成论文摘要