Dual Optimization-Based Distributed Tracking Control Under Completely Unknown Dynamics
IEEE TRANSACTIONS ON AUTOMATION SCIENCE AND ENGINEERING(2024)
摘要
Unlike existing results on output tracking control in multi-agent systems, which mainly focus on the relative state among agents, in some cases the state of the system may not be available or measurable. In this paper, we investigate the model-free learning-based distributed optimal tracking control for heterogeneous multi-agent systems with dynamic output feedback under a switching reinforcement learning algorithm. First, a relative output-based distributed observer is developed without exchanging state information, which can significantly reduce the interaction load and broaden the range of applications. Then, a distributed feedback-feedforward controller is proposed, where the optimal feedback and feedforward gain matrices can be learned online by solving two optimization problems, employing policy iteration-based reinforcement learning instead of relying on the leader's state as in existing studies. Subsequently, the policy iteration algorithm (PI) is modified into the value iteration (VI) learning algorithm, which can relax the requirement for an initial stabilizing control policy and does not depend on the known dynamical model. Additionally, a switching reinforcement learning algorithm is put forward by fully integrating the merits of the previously mentioned methods. The new algorithm not only overcomes the initial stabilizing assumption, but also ensures the convergence of the algorithm in a model-free fashion. Finally, a simulation example is provided to illustrate the theoretical analysis.
更多查看译文
关键词
Heterogeneous multi-agent systems (HMASs),distributed cooperative control,unknown dynamics
AI 理解论文
溯源树
样例
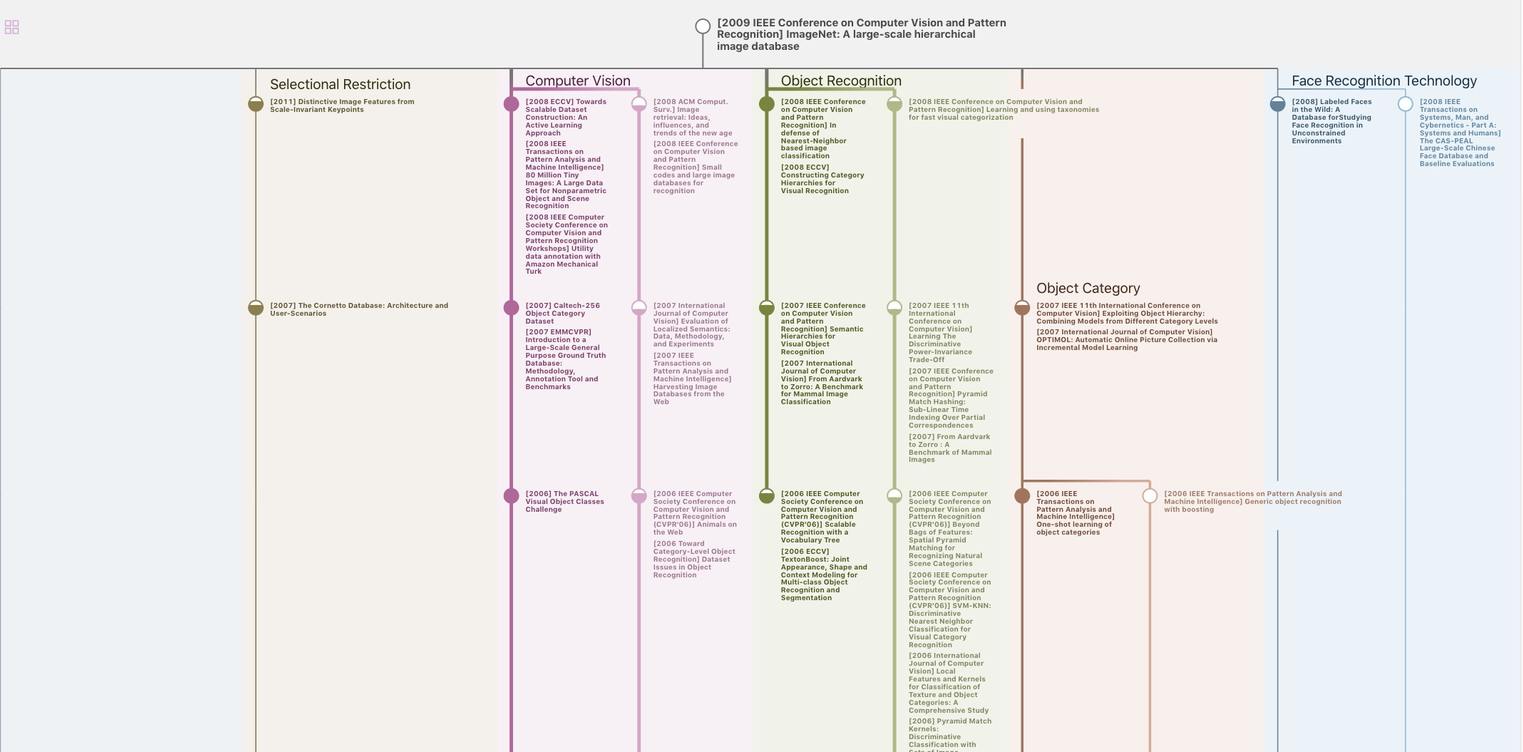
生成溯源树,研究论文发展脉络
Chat Paper
正在生成论文摘要