Subclassification of obesity for precision prediction of cardiometabolic diseases: an IMI SOPHIA study
crossref(2024)
摘要
Abstract Obesity and cardiometabolic disease often, but not always, coincide. Distinguishing sub-populations within which cardiometabolic risk diverges from the risk expected for a given body mass index (BMI) may facilitate precision prevention of cardiometabolic diseases. Accordingly, we performed unsupervised clustering in four European population-based cohorts (N ~ 173K). We detected five discordant profiles consisting of individuals with cardiometabolic biomarkers higher or lower than expected given their BMI, in total representing ~20% of the total population. Persons with discordant profiles differed from concordant individuals in prevalence and future risk of major adverse cardiovascular events (MACE) and diabetes. Subtle BMI-discordances in biomarkers affected disease risk. For instance, a 10% higher probability of having a discordant lipid profile was associated with a 5% higher risk of MACE (HR in women: 1.05, 95% CI: 1.03, 1.06, p = 4.19x10-10; HR in men: 1.05, 95% CI: 1.04, 1.06, p= 9.33x10-14). Multivariate prediction models for MACE and diabetes performed better when incorporating discordant profile information (likelihood ratio test P < 0.001). This enhancement represents an additional net benefit of 4 to 15 additional correct interventions, and 37 to 135 additional unnecessary interventions correctly avoided for every 10,000 individuals tested.
更多查看译文
AI 理解论文
溯源树
样例
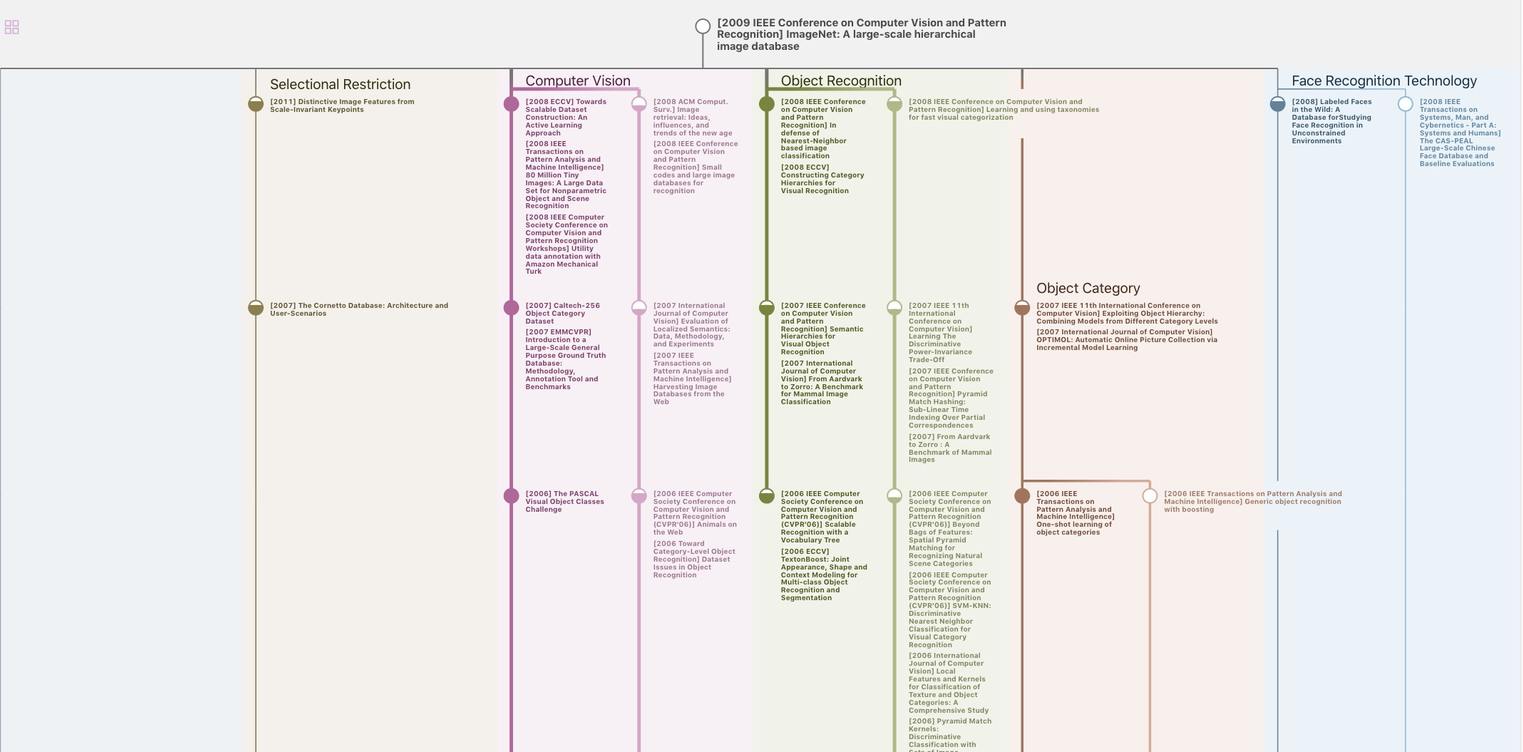
生成溯源树,研究论文发展脉络
Chat Paper
正在生成论文摘要