Thick Cloud Removal in Multitemporal Remote Sensing Images Using a Coarse-to-Fine Framework
IEEE Geoscience and Remote Sensing Letters(2024)
摘要
Abstract—Remote sensing (RS) images are widely used for Earth observation. However, cloud contamination greatly degrades the quality of RS images and limits their applications. In this letter, we propose a coarse-to-fine thick cloud removal method for a single pair of multitemporal RS images. First, we perform a global color transformation on a cloud-free reference image using linear regression coefficients between the pixels in the cloudy target image and the reference image in the same cloud-free regions, and obtain a coarse result. Then, a convolutional neural network (CNN) based on internal constraint is used to refine the coarse result, which does not require any construction of additional external training dataset in advance. We further design a multiscale feature extraction and fusion module and an auxiliary loss involving cloud regions to improve the performance of the CNN. Finally, Poisson image fusion is employed to generate a seamless cloud-free result. On a simulated test set containing 500 pairs of multitemporal RS images, the proposed method achieves satisfactory results with 25.1277 dB in peak signal-to-noise ratio (PSNR), 0.9077 in structural similarity (SSIM), and 0.9342 in correlation coefficient (CC). Qualitative and quantitative comparisons of our proposed against several state-of-the-art methods on the simulated and real cloudy images demonstrate the superiority of the proposed method.
更多查看译文
关键词
Thick cloud removal,multitemporal remote sensing images,convolutional neural network,internal constraint
AI 理解论文
溯源树
样例
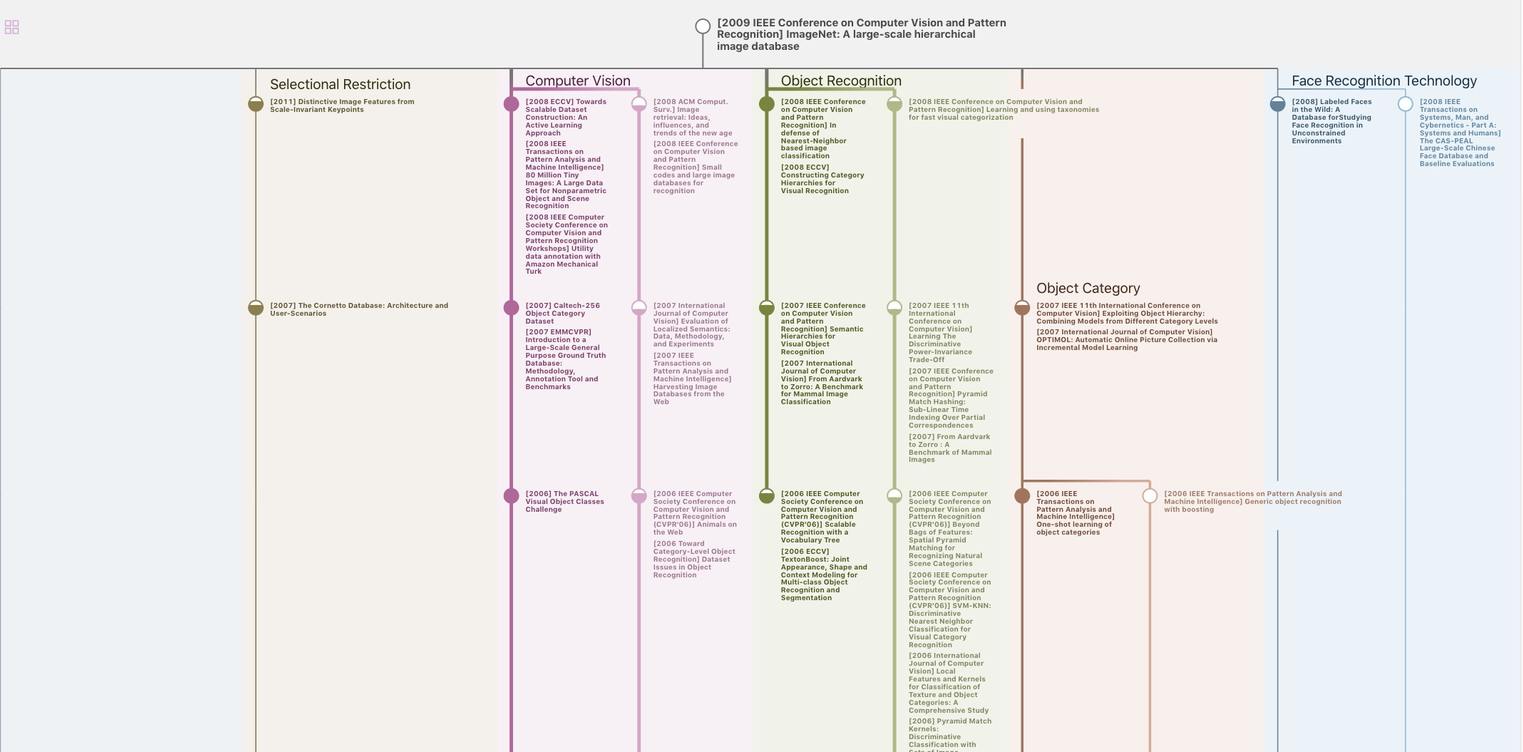
生成溯源树,研究论文发展脉络
Chat Paper
正在生成论文摘要