Dynamic Environment Joint Object Detection and Depth Image Segmentation for Visual SLAM
2023 China Automation Congress (CAC)(2023)
摘要
Addressing the challenges posed by inadequate localization precision and system vulnerability in conventional visual SLAM algorithms when applied in dynamic settings, this study introduces a visual SLAM framework designed for dynamic indoor environments. The system is based on the ORB-SLAM2 algorithm framework with improvements to its tracking thread. First, an independent object detection thread based on YOLOv7 is introduced to perform object detection on each incoming frame and extract potentially moving objects from the scene. Simultaneously, the tracking thread uses the feature point extraction method with adaptive thresholding proposed in this paper to extract feature points from the image and compute the optical flow of these feature points. By merging the outcomes of object detection with the optical flow data derived from the feature points, the motion status of the identified objects is ascertained. Subsequently, the bounding box of the dynamic object is amalgamated with the depth image segmentation algorithm presented in this paper, from which the foreground image mask of the target detection box is extracted and the feature points within the mask are eliminated. Ultimately, the remaining stationary feature points are employed for constructing maps and determining the location. Tests on the public TUM dataset show that the root mean square error of the absolute trajectory error is reduced by an average of 96.5% compared to ORB-SLAM2, validating that the suggested approach attains enhanced precision in localization and resilience in dynamic indoor settings.
更多查看译文
关键词
ORB-SLAM2,dynamic environments,object detection,depth segmentation
AI 理解论文
溯源树
样例
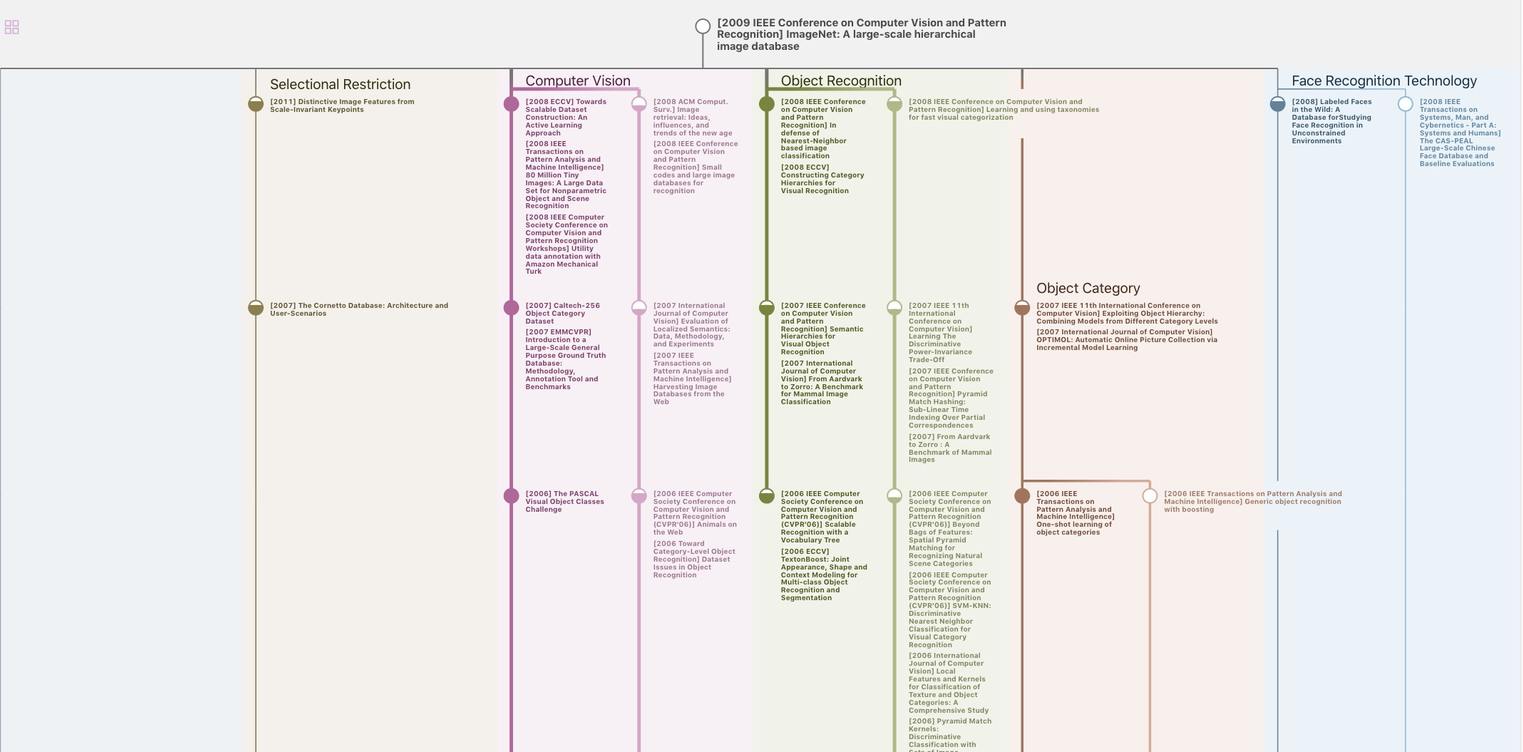
生成溯源树,研究论文发展脉络
Chat Paper
正在生成论文摘要