Eigen-CNN: Eigenimages plus Eigennoise Level Maps Guided Network for Hyperspectral Image Denoising
IEEE Transactions on Geoscience and Remote Sensing(2024)
摘要
In recent years, neural network-based methods have shown promising results in hyperspectral image (HSI) denoising area. Real HSIs exhibit substantial variations in noise distribution due to various factors such as different imaging techniques, camera variations, imaging environments, and hardware aging. In this paper, we develop an eigenimage plus eigennoise level map guided convolutional neural network for HSI denoising. Our main idea is to perform eigendecomposition on HSIs, utilize the low-rank property of HSIs in the spectral dimension and approximate the spectral vectors in a low-dimensional orthogonal subspace, where representation coefficients are called eigenimages. Besides eigenimages, we make use of estimated eigennoise level map as an input to guide the network for denoising. The proposed network can be constructed without restriction in the number of eigencomponents by using all eigenimages and eigennoise level maps of training noisy-clean pairs. In the inference part, the trained network can be used to remove noise in observed eigenimages without restriction in the number of eigencomponents, and an underlying clean image HSI can be estimated by performing orthogonal projection back. Experimental results on both simulated and real HSIs demonstrate the effectiveness of our trained Eigen-CNN compared with state-of-the-art HSI denoising methods. A MATLAB demo of this work is available at https://github.com/LinaZhuang/HSI-denoiser-Eigen-CNN for the sake of reproducibility.
更多查看译文
关键词
Hyperspectral image restoration,convolutional neural network,deep neural network,low-rank representation,subspace representation
AI 理解论文
溯源树
样例
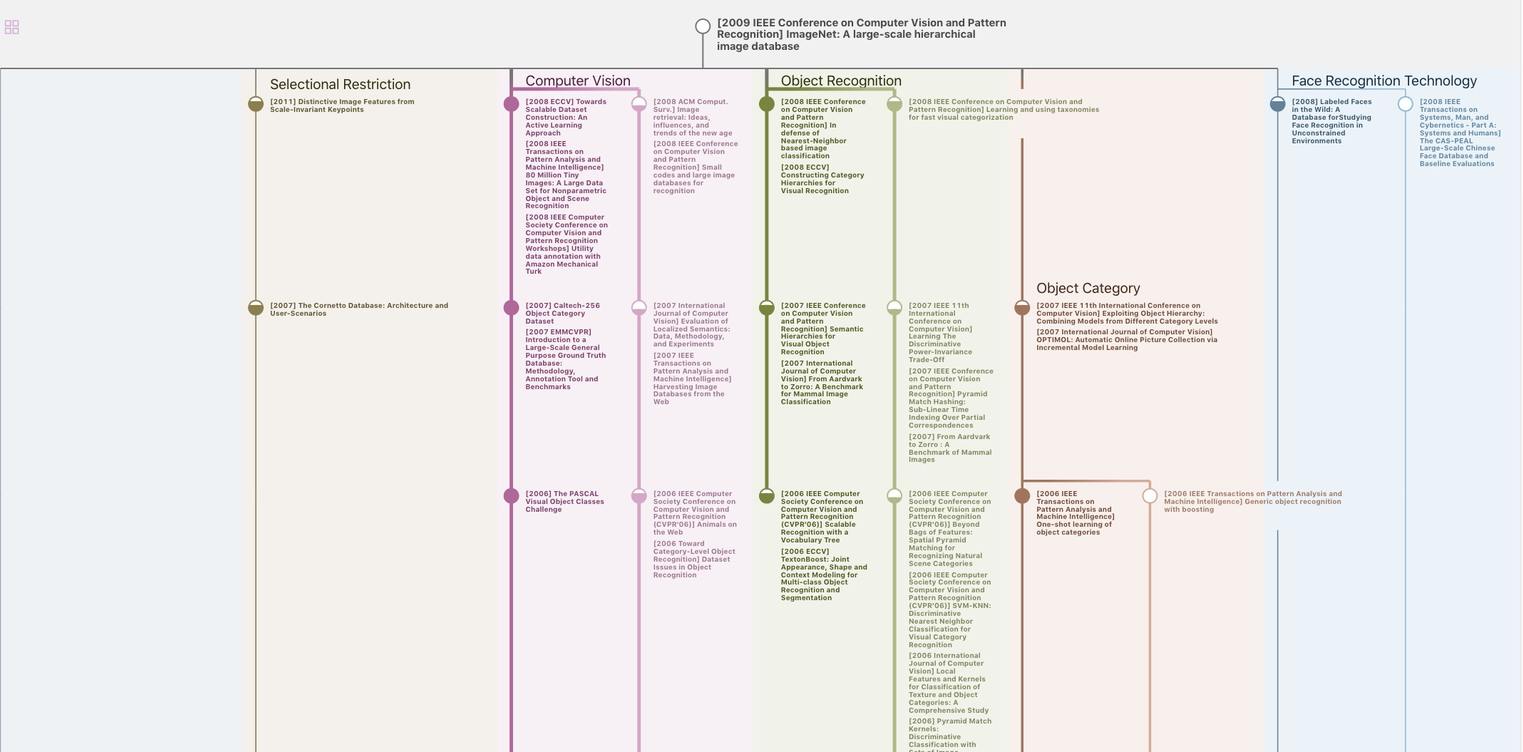
生成溯源树,研究论文发展脉络
Chat Paper
正在生成论文摘要