A two-step penalization and shrinkage approach for binary response data that is jointly separated and correlated: The effects of social networks on diarrheal disease
crossref(2024)
摘要
Epidemiologic data often violate common modeling assumptions of independence between subjects due to study design. Statistical separation is also common, particularly in the study of rare binary outcomes. Statistical separation for binary outcomes occurs when regions of the covariate space have no variation in the outcome, and separation can negatively impact the validity of logistic regression model parameters. When data are correlated, we generally use multi-level modeling for parameter estimation, and statistical approached have also been developed for handling statistical separation. Approaches for analyzing data with both separation and complex correlation, however, are not well-known. Extending prior work, we demonstrate a two-stage Bayesian modeling approach to account for both separated and highly correlated data through a motivating example examining the effect of social ties on Acute Gastrointestinal Illness (AGI) in rural Ecuador. The two-stage approach involves fitting a Bayesian hierarchical model to account for correlation using priors derived from parameter estimates from a Firth-corrected logistic regression model to account for separation. We compare estimates from the two-stage approach to standard regression methods that only account for either separation or correlation. Our results demonstrate that correctly accounting for separation and correlation when both are present can potentially provide better inference.
### Competing Interest Statement
The authors have declared no competing interest.
### Funding Statement
This work was funded by the National Institutes of Health (grant R01- AI050038) and the University of Michigan.
### Author Declarations
I confirm all relevant ethical guidelines have been followed, and any necessary IRB and/or ethics committee approvals have been obtained.
Yes
The details of the IRB/oversight body that provided approval or exemption for the research described are given below:
All study participants provided informed consent and all data collection protocols were approved by institutional review boards at the University of Michigan, Trinity College, Hartford, and the Universidad San Francisco de Quito.
I confirm that all necessary patient/participant consent has been obtained and the appropriate institutional forms have been archived, and that any patient/participant/sample identifiers included were not known to anyone (e.g., hospital staff, patients or participants themselves) outside the research group so cannot be used to identify individuals.
Yes
I understand that all clinical trials and any other prospective interventional studies must be registered with an ICMJE-approved registry, such as ClinicalTrials.gov. I confirm that any such study reported in the manuscript has been registered and the trial registration ID is provided (note: if posting a prospective study registered retrospectively, please provide a statement in the trial ID field explaining why the study was not registered in advance).
Yes
I have followed all appropriate research reporting guidelines, such as any relevant EQUATOR Network research reporting checklist(s) and other pertinent material, if applicable.
Yes
The data underlying this article cannot be shared publicly for the privacy of individuals that participated in the study and until other analyses that are part of the primary objectives of the study are complete. The data will be shared on reasonable request to the corresponding author. Code is available on github ().
更多查看译文
AI 理解论文
溯源树
样例
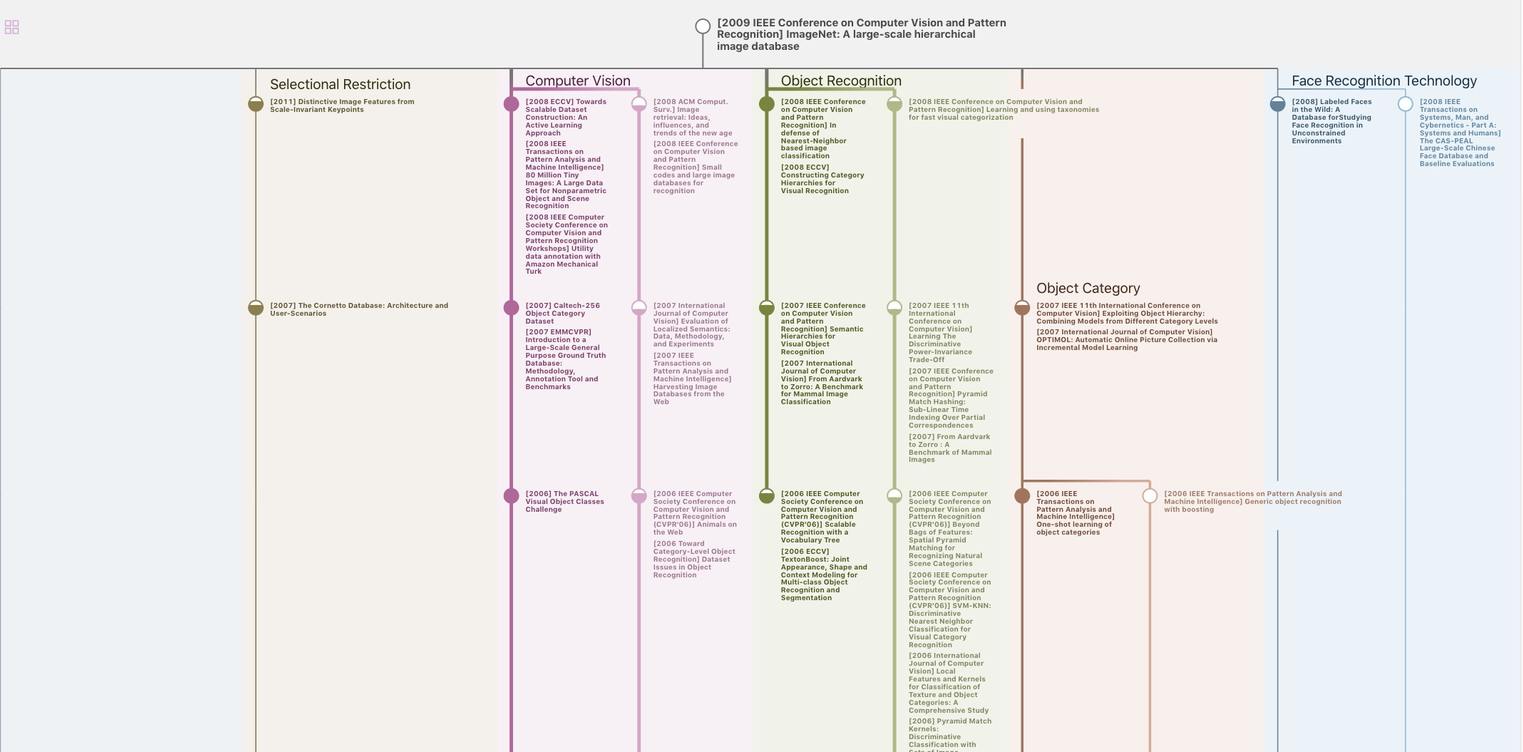
生成溯源树,研究论文发展脉络
Chat Paper
正在生成论文摘要