An interpretable deep learning multi-dimensional integration framework for exchange rate forecasting based on deep and shallow feature selection and snapshot ensemble technology
Engineering Applications of Artificial Intelligence(2024)
摘要
Accurate exchange rate forecasting is important for the better realization of international economic transactions and international currency investments. However, it is challenging to forecast exchange rates due to their high volatility, nonlinearity, and noisy characteristics. This paper creatively proposes a novel interpretable deep learning multi-dimensional integration framework for foreign exchange rate forecasting based on shallow and deep feature selection and snapshot ensemble technology. The main factors affecting exchange rates are considered at a deep and shallow level, and the feature selection project is implemented. A cluster-based multidimensional learning paradigm is proposed. The framework clusters data and constructs predictive sub-models and then embeds snapshot ensemble techniques into models to improve the robustness of single models. Finally, the results are nonlinearly integrated by a deep learning model. In addition, three datasets and twelve comparative models were used to demonstrate the performance of the models. The empirical results show that the proposed model has the smallest mean absolute percentage error (0.407859%, 0.47134%, and 0.470167%, respectively).
更多查看译文
关键词
Multi-dimensional integration,Deep learning,Fuzzy clustering,Interpretable artificial intelligence,Deep and shallow feature analysis
AI 理解论文
溯源树
样例
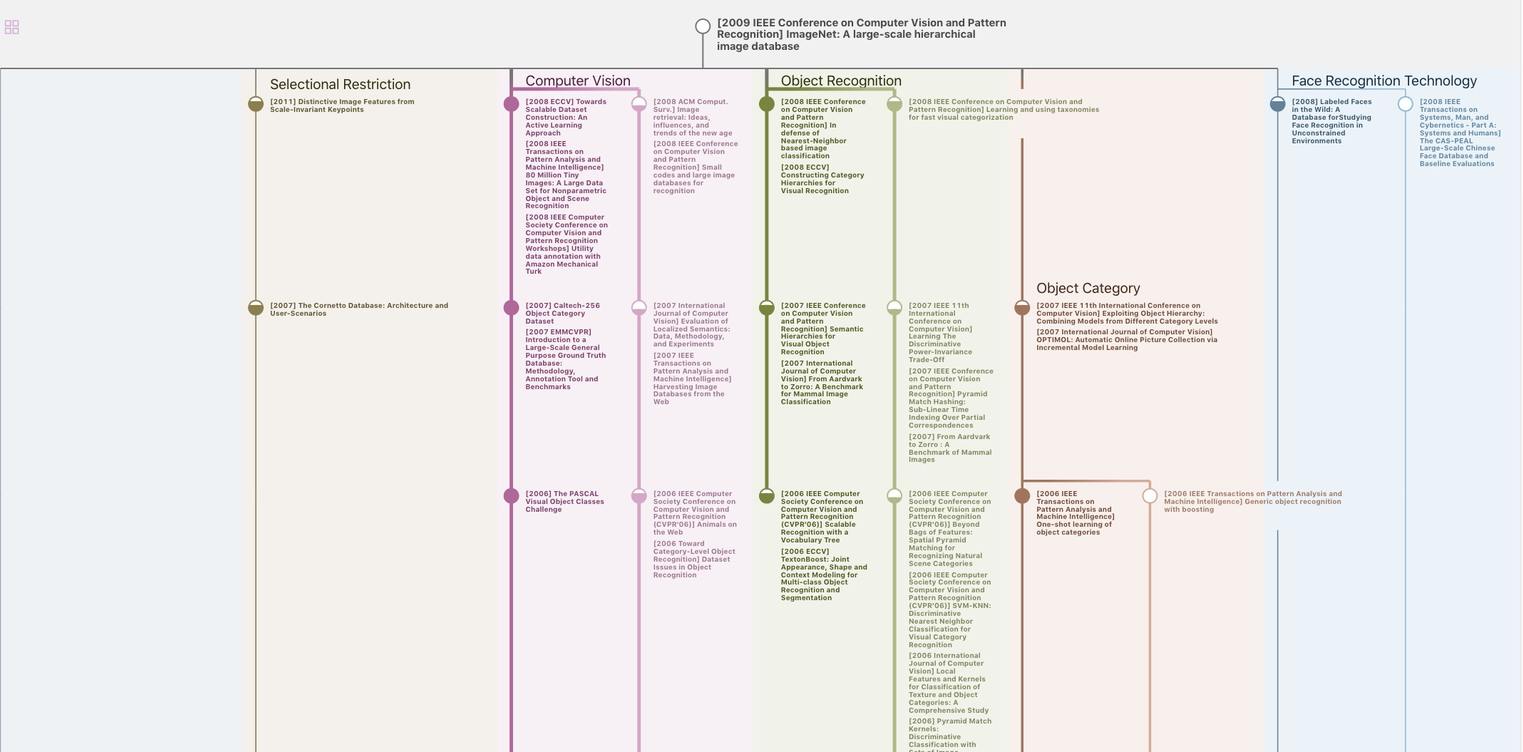
生成溯源树,研究论文发展脉络
Chat Paper
正在生成论文摘要