Speech Guided Masked Image Modeling for Visually Grounded Speech
ICASSP 2024 - 2024 IEEE International Conference on Acoustics, Speech and Signal Processing (ICASSP)(2024)
摘要
The objective of this study is to investigate the learning process of Visually Grounded Speech (VGS) models through joint learning that combines contrastive learning and masked image modeling. Typically, VGS models ahn to establish audio-visual alignment between images and then spoken captions within a contrastive learning framework. Building upon this seminal concept, in this work, we explore whether visual reconstruction with the help of cross-modality can enhance alignment, given that spoken captions describe visual appearances. To achieve this, we extend the contrastive learning-based VGS models by incorporating a masked autoencoder that utilizes cross-attention in the decoder. Through this cross-modal interaction in the decoder, spoken caption features guide the model to reconstruct the masked patches and capture correspondence between the two modalities. Our findings suggest that integrating cross-modal reconstruction within the contrastive learning framework enhances audio-visual feature alignment. Consequently, our proposed method gives comparable performance to existing models that utilize prior knowledge or other modalities, such as object region proposals or Contrastive Language-Image Pretraining (CLIP).
更多查看译文
关键词
Visually Grounded Speech,Self-supervised Learning,Masked Autoencoder,Contrastive Learning
AI 理解论文
溯源树
样例
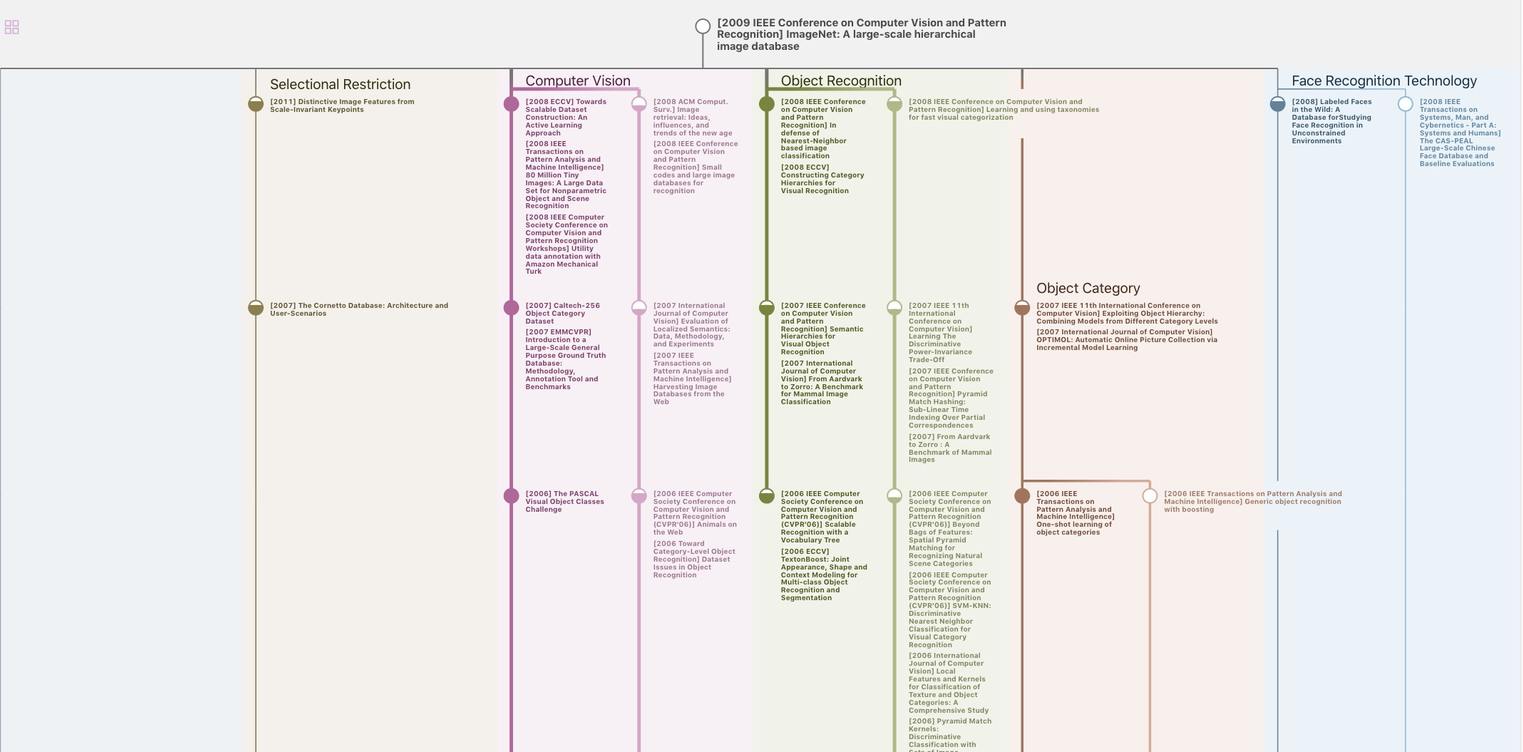
生成溯源树,研究论文发展脉络
Chat Paper
正在生成论文摘要