How to Bridge Graph and Sequence Patterns in Session-Based Recommendation? A Self-Supervised Method
ICASSP 2024 - 2024 IEEE International Conference on Acoustics, Speech and Signal Processing (ICASSP)(2024)
摘要
Session-based Recommendation aims to reveal the item distribution patterns in anonymous session sequences. Most existing approaches model the distribution patterns by utilizing either sequential or structural information individually to absorb different pattern knowledge, which can only model the distinct one-sided facet of item distribution in sessions, thus leading to suboptimal performance. Self-supervised learning provides a natural solution as a bridge to fill the gap between different learning paradigms in session-based recommendations, which remains unexplored. In this paper, we regard the distinct learning paradigm as an individual channel and then integrate the sequential and graphical channels with a contrastive bridge architecture. We name the novel framework DC-Rec, for Dual Channel Recommendation, to model the comprehensive session characteristics. Extensive experiments conducted on two real-world datasets demonstrate that our model consistently outperforms the state-of-the-art recommendation methods.
更多查看译文
关键词
Session-based Recommendation,Graph Neural Networks,Recurrent Neural Networks,Self-supervised Learning
AI 理解论文
溯源树
样例
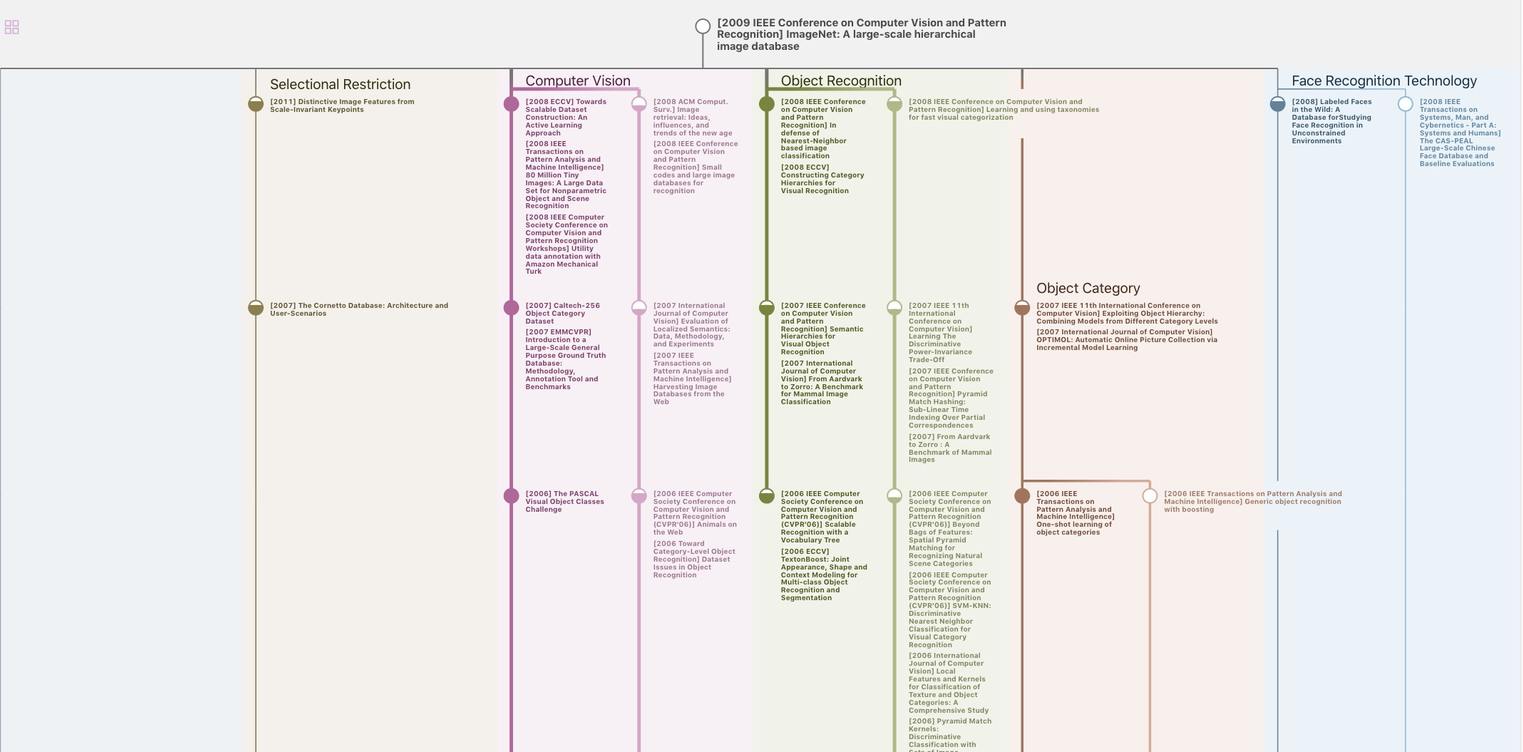
生成溯源树,研究论文发展脉络
Chat Paper
正在生成论文摘要