Beyond the Limit of Weight-Sharing: Pioneering Space-Evolving NAS with Large Language Models
ICASSP 2024 - 2024 IEEE International Conference on Acoustics, Speech and Signal Processing (ICASSP)(2024)
摘要
Large language models (LLMs) offer impressive performance across diverse fields, but their increasing complexity raises both design costs and the need for specialized expertise. These challenges are intensified for Neural Architecture Search (NAS) methods reliant on weight-sharing techniques. This paper introduces GNAS, a new NAS method that boosts the search process with the aid of LLMs for efficient model discovery. With insights from existing architectures, GNAS swiftly identifies superior models that can adapt to changing resource constraints. We provide a mathematical framework to facilitate the transfer of knowledge across different model sizes, thereby improving search efficiency. Our experiments conducted on ImageNet, NAS-Bench-Macro, and ChannelBench-Macro confirm the effectiveness of GNAS across both CNN and Transformer architectures.
更多查看译文
关键词
NAS,space evolving,LLM
AI 理解论文
溯源树
样例
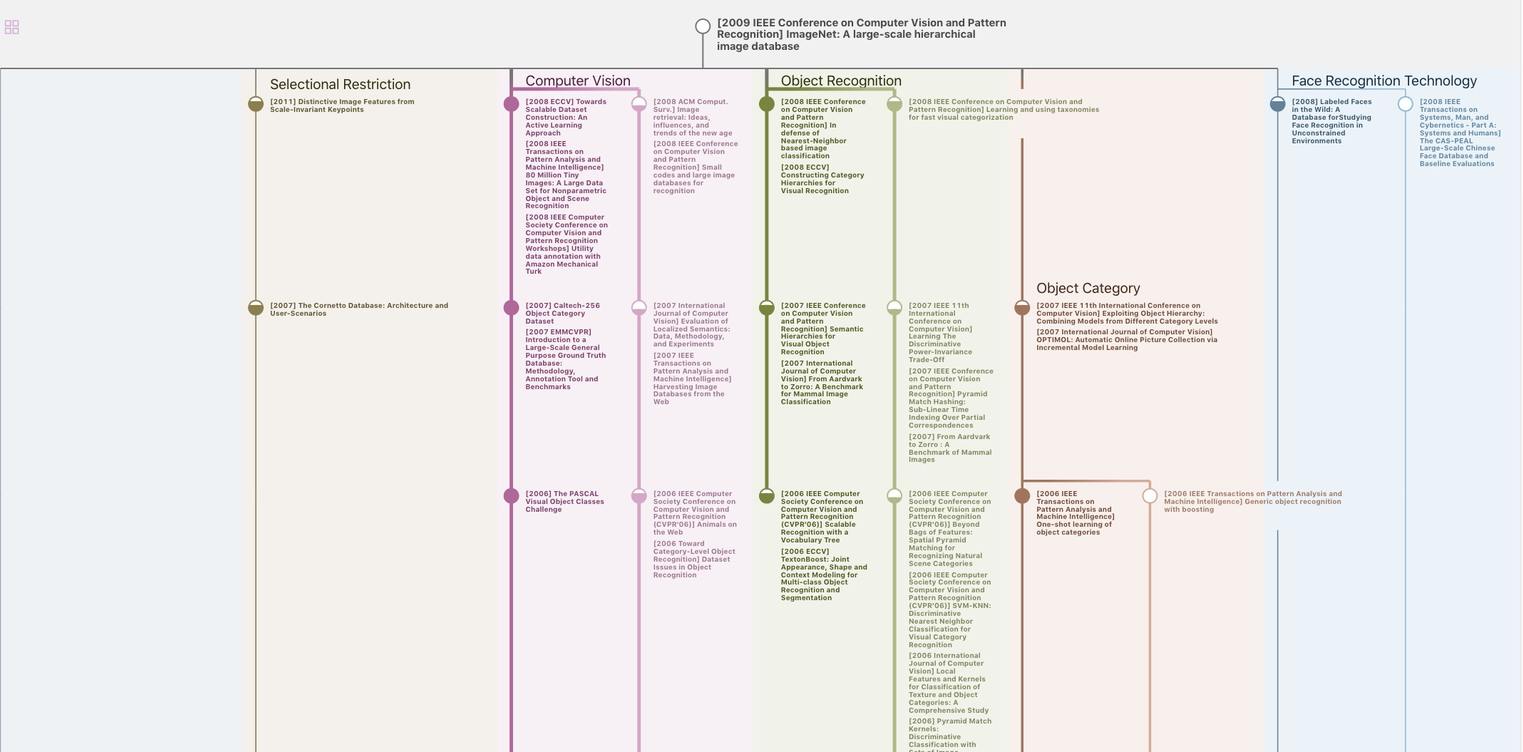
生成溯源树,研究论文发展脉络
Chat Paper
正在生成论文摘要