FDIG: A Fine-Grained Data Integration Approach for Group Recommendation
ICASSP 2024 - 2024 IEEE International Conference on Acoustics, Speech and Signal Processing (ICASSP)(2024)
摘要
Effective group recommendation systems play a pivotal role in enriching the information consumption of users from different groups. Existing group recommendation approaches face challenges such as the sparsity of the rating matrix and low specificity between user clusters, leading to cold-start issue. In this paper, we propose a Fine-grained Data Integration approach for Group Recommendation (FDIG) that enables the fine-grained fusion of items in the same group without impacting other groups. FDIG reformulate the data integration task as a bi-level optimization problem, with an end-to-end loss function. To solve the problem efficiently, we propose an effective learning algorithm to obtain an admissible solution. Experimental results demonstrate that FDIG own the ability to accurately fuse items in group recommendation, which offers a practical solution to enhance group recommendation performance and user personalization.
更多查看译文
关键词
group recommendation,data integration,bi-level optimization
AI 理解论文
溯源树
样例
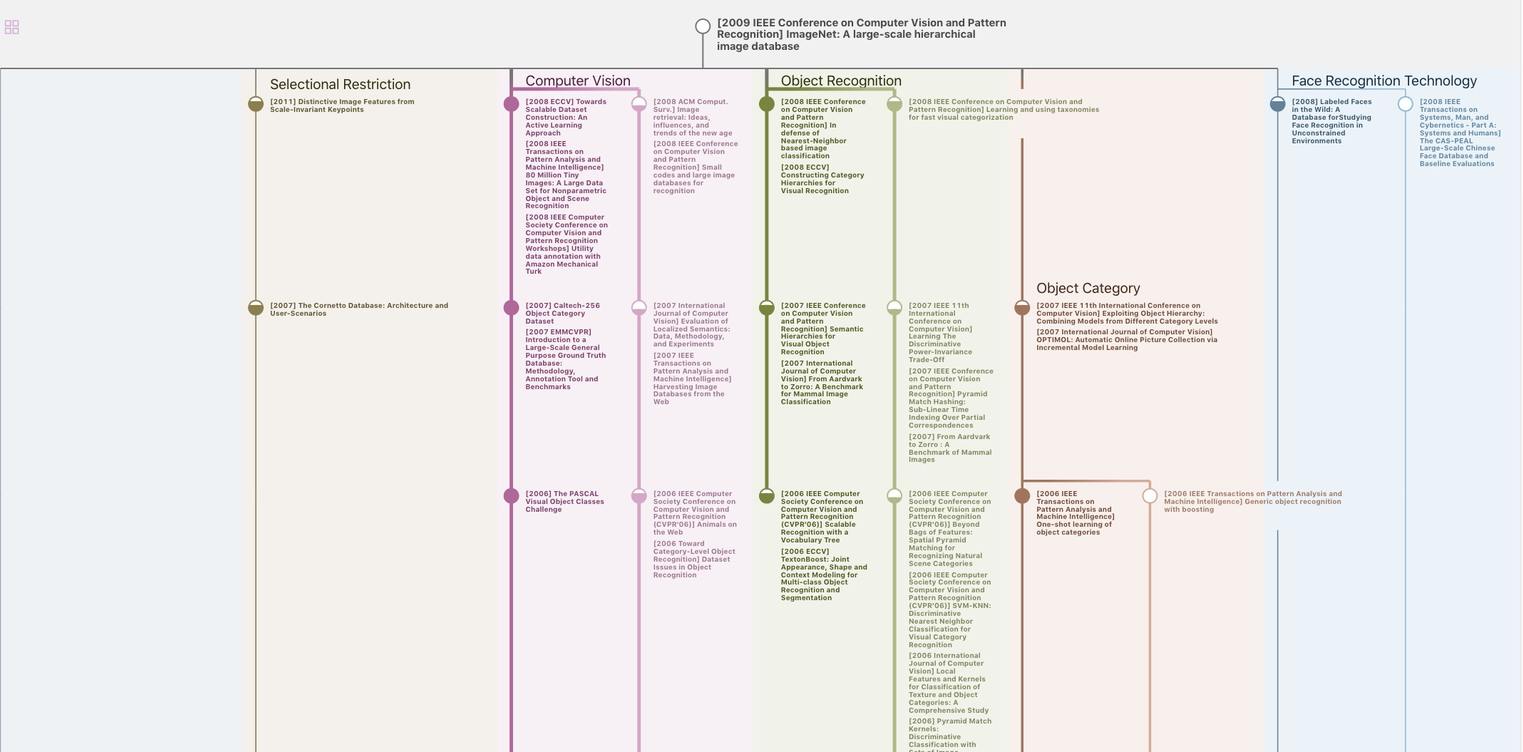
生成溯源树,研究论文发展脉络
Chat Paper
正在生成论文摘要