HLS-FGVC: Hierarchical Label Semantics Enhanced Fine-Grained Visual Classification
ICASSP 2024 - 2024 IEEE International Conference on Acoustics, Speech and Signal Processing (ICASSP)(2024)
摘要
Fine-grained visual classification (FGVC) intends to confirm the sub-classes of a specific object category, e.g., identifying the species of dogs or birds. It is a challenging problem with the inter-class similarity among these sub-categories and intra-class variance in every fine-grained class. Most of the recent works intend to learn discriminative representations and class-consistency features. However, they only take the finest labels into account. We argue that the hierarchical label structure (HLS) implied in the category names can enhance the FGVC task. In this paper, we proposed two modules to leverage the hierarchical label structure. (i) We build a weighted graph in each batch based on the hierarchical label structure, the nodes of which are image features. The messages are passed among graph nodes for feature interaction. (ii) A hierarchy-aware ranking loss is proposed to regularize the distribution in feature space. The ablation study and experimental results show that our proposed modules achieve significant improvements over previous works.
更多查看译文
关键词
Label semantic,representation learning,fine-grained classification,multi-granularity classification
AI 理解论文
溯源树
样例
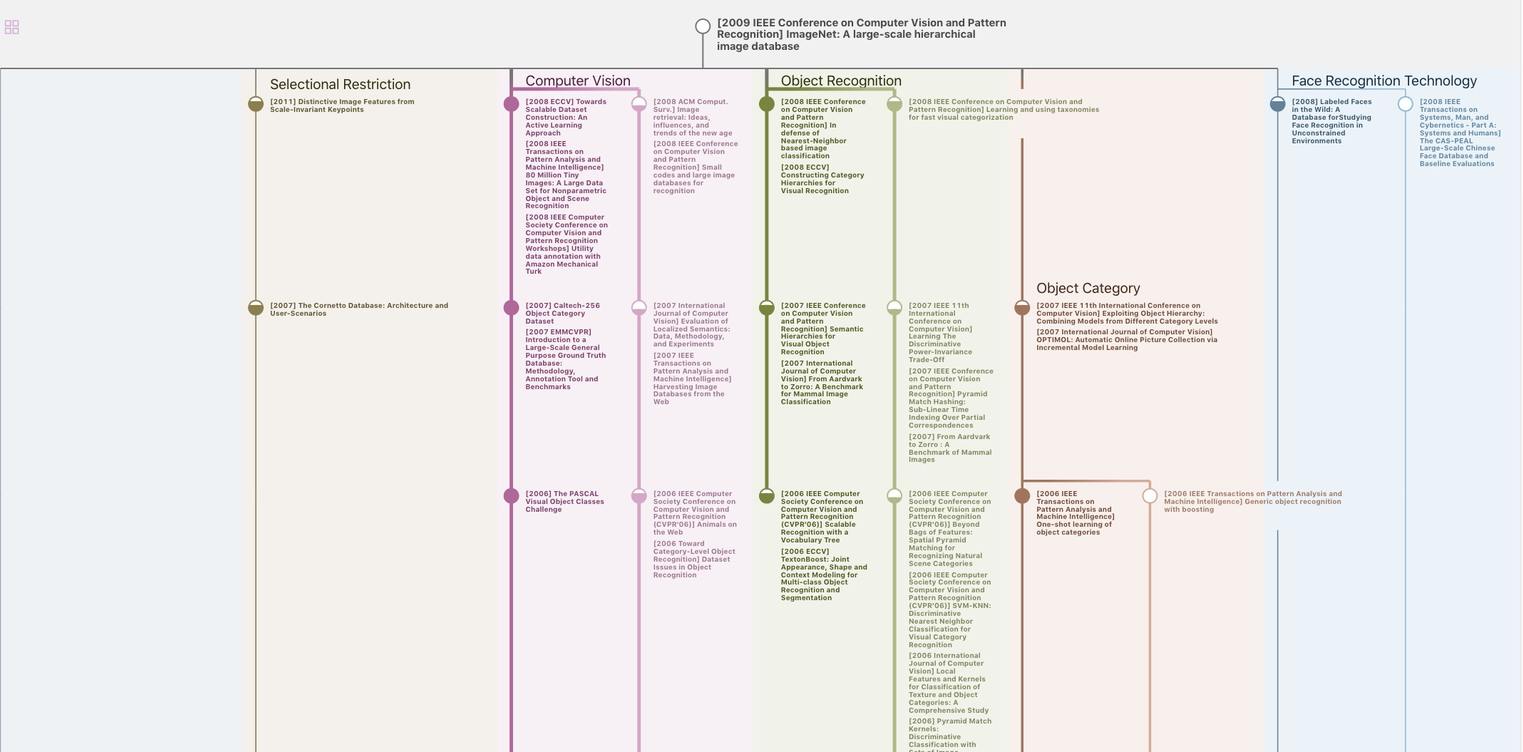
生成溯源树,研究论文发展脉络
Chat Paper
正在生成论文摘要