LL-UNet++:UNet++ Based Nested Skip Connections Network for Low-Light Image Enhancement
IEEE TRANSACTIONS ON COMPUTATIONAL IMAGING(2024)
摘要
Enhancing low-light images presents several challenges, such as image darkness, severe color distortion, and noise. To address these issues, we propose a novel low-light image enhancement algorithm with nested skip connections based on UNet++. This design facilitates the propagation of finer features and improves information transmission, resulting in better enhancement of image brightness, reduction of color distortion, and retention of finer details. To eliminate noise potentially introduced by skip connections, we designed a specific residual block based on Instance Normalization (IN). IN can process each sample independently, allowing the model to better adapt to each image's specific lighting conditions and noise levels. In addition, we propose a new hybrid loss function that simultaneously emphasizes multiple critical attributes of an image, yielding superior enhancement results on multiple key metrics. The proposed algorithm achieves advanced performance on the LOL dataset, scoring 23.0047 and 0.8682 on the PSNR and SSIM metrics, respectively. Extensive experiments demonstrate the effectiveness and superiority of our proposed algorithm.
更多查看译文
关键词
Low-light image enhancement,UNet++,deep neural network
AI 理解论文
溯源树
样例
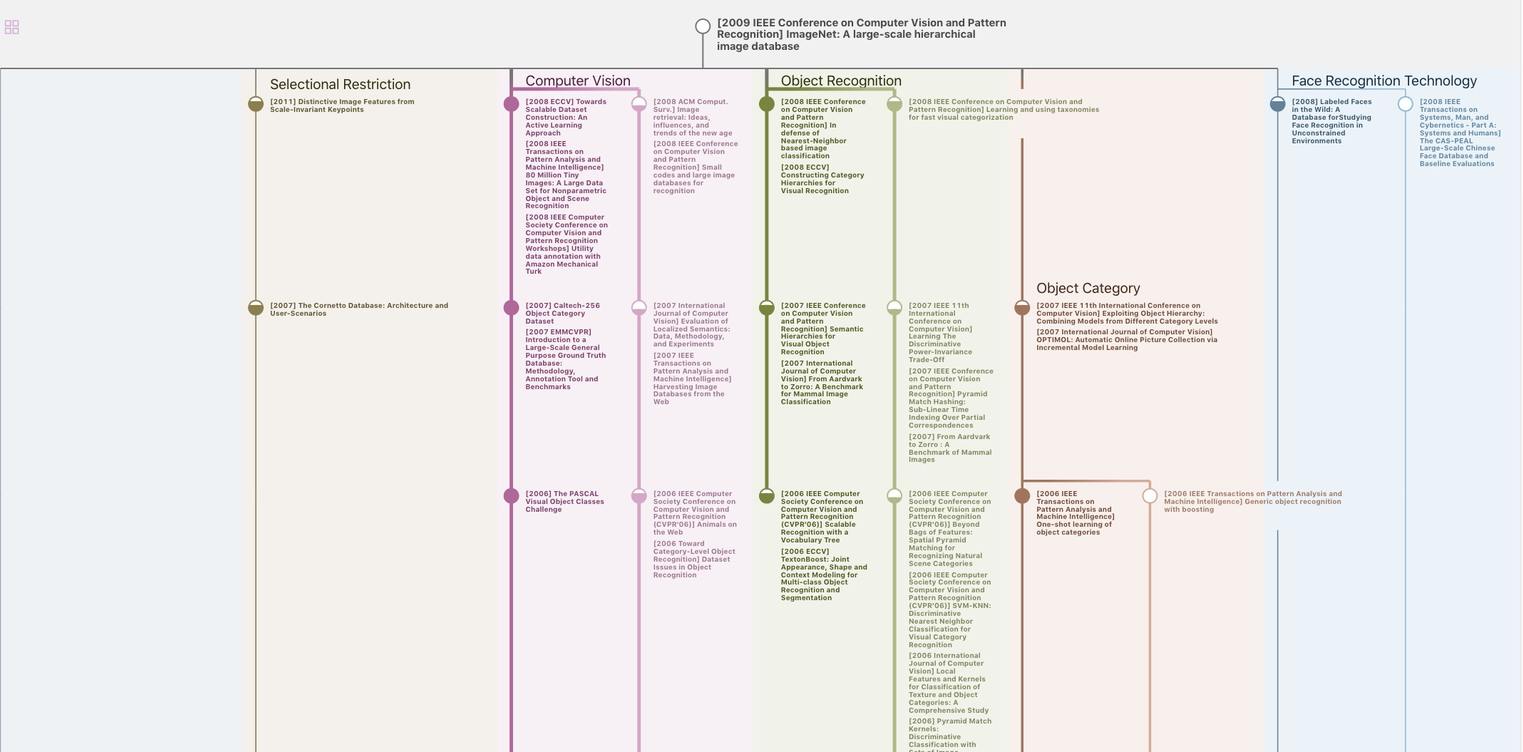
生成溯源树,研究论文发展脉络
Chat Paper
正在生成论文摘要